Embedding Normalization: Significance Preserving Feature Normalization for Click-Through Rate Prediction.
2021 International Conference on Data Mining Workshops (ICDMW)(2021)
摘要
Normalization techniques are known to provide faster model convergence and good generalization performance, having achieved great success in computer vision and natural language processing. Recently, several deep neural network-based click-through rate (CTR) prediction models have applied such normalization techniques to their deep network components to make model training stable. However, we observe that applying existing normalization techniques (e.g. Batch Normalization and Layer Normalization) to feature embedding of the models leads to the degradation of model performance. In this study, we conjecture that existing normalization techniques can easily ignore the significance of each feature embedding, leading to suboptimal performance. To support our claim, we theoretically show that existing normalization techniques tend to equalize the norm of individual feature embedding. To overcome this limitation, we propose a theory-inspired normalization technique, called Embedding Normalization, which not only makes model training stable but also improves the performance of CTR prediction models by preserving the significance of each feature embedding. Through extensive experiments on various real-world CTR prediction datasets, we show that our proposed normalization technique leads to faster model convergence and achieves better or comparable performance than other normalization techniques. Especially, our Embedding Normalization is effective in not only deep neural network-based CTR prediction models but also shallow CTR prediction models that do not utilize deep neural network components.
更多查看译文
关键词
Click-Through Rate Prediction,Embedding Normalization,Factorization Machines
AI 理解论文
溯源树
样例
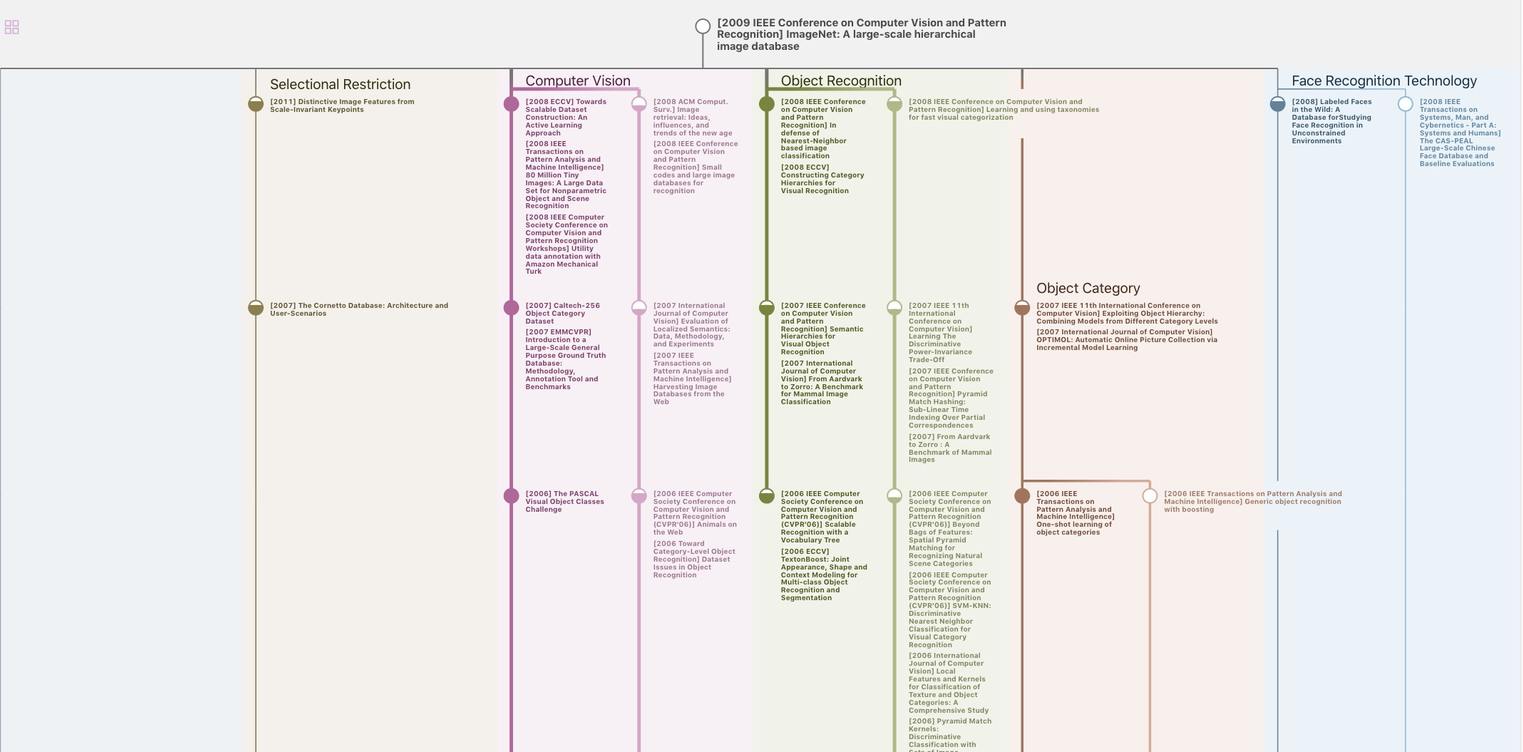
生成溯源树,研究论文发展脉络
Chat Paper
正在生成论文摘要