Fast and lightweight binary and multi-branch Hoeffding Tree Regressors.
2021 International Conference on Data Mining Workshops (ICDMW)(2021)
摘要
Incremental Hoeffding Tree Regressors (HTR) are powerful non-linear online learning tools. However, the commonly used strategy to build such structures limits their applicability to real-time scenarios. In this paper, we expand and evaluate Quantization Observer (QO), a feature discretization-based tool to speed up incremental regression tree construction and save memory resources. We enhance the original QO proposal to create multi-branch trees when dealing with numerical attributes, creating a mix of interval and binary splits rather than binary splits only. We evaluate the multi-branch and strictly binary QO-based HTRs against other tree-building strategies in an extensive experimental setup of 15 data streams. In general, the QO-based HTRs are as accurate as traditional HTRs, incurring one-third of training time at only a fraction of the memory resource usage. The obtained numerical multi-branch HTRs are shallower than the strictly binary ones, significantly faster to train, and they keep predictive performance similar to the traditional incremental trees.
更多查看译文
关键词
Hoeffding tree regressor,online learning,incremental learning,computational resource savings
AI 理解论文
溯源树
样例
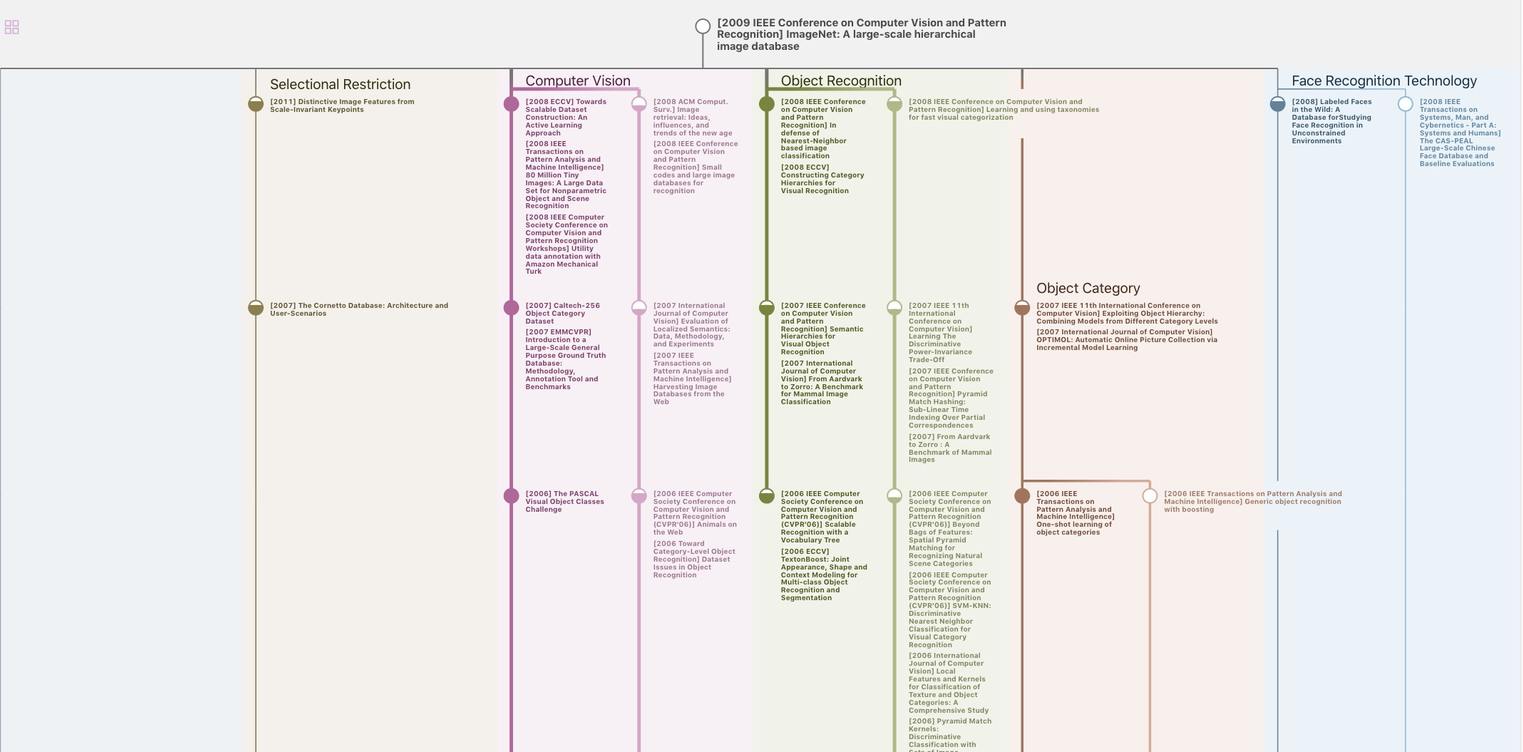
生成溯源树,研究论文发展脉络
Chat Paper
正在生成论文摘要