Fluid Overload Phenotypes in Critical Illness-A Machine Learning Approach
JOURNAL OF CLINICAL MEDICINE(2022)
摘要
Background: The detrimental impact of fluid overload (FO) on intensive care unit (ICU) morbidity and mortality is well known. However, research to identify subgroups of patients particularly prone to fluid overload is scarce. The aim of this cohort study was to derive "FO phenotypes" in the critically ill by using machine learning techniques. Methods: Retrospective single center study including adult intensive care patients with a length of stay of >= 3 days and sufficient data to compute FO. Data was analyzed by multivariable logistic regression, fast and frugal trees (FFT), classification decision trees (DT), and a random forest (RF) model. Results: Out of 1772 included patients, 387 (21.8%) met the FO definition. The random forest model had the highest area under the curve (AUC) (0.84, 95% CI 0.79-0.86), followed by multivariable logistic regression (0.81, 95% CI 0.77-0.86), FFT (0.75, 95% CI 0.69-0.79) and DT (0.73, 95% CI 0.68-0.78) to predict FO. The most important predictors identified in all models were lactate and bicarbonate at admission and postsurgical ICU admission. Sepsis/septic shock was identified as a risk factor in the MV and RF analysis. Conclusion: The FO phenotypes consist of patients admitted after surgery or with sepsis/septic shock with high lactate and low bicarbonate.
更多查看译文
关键词
fluid resuscitation, fluid overload, intensive care, risk factors
AI 理解论文
溯源树
样例
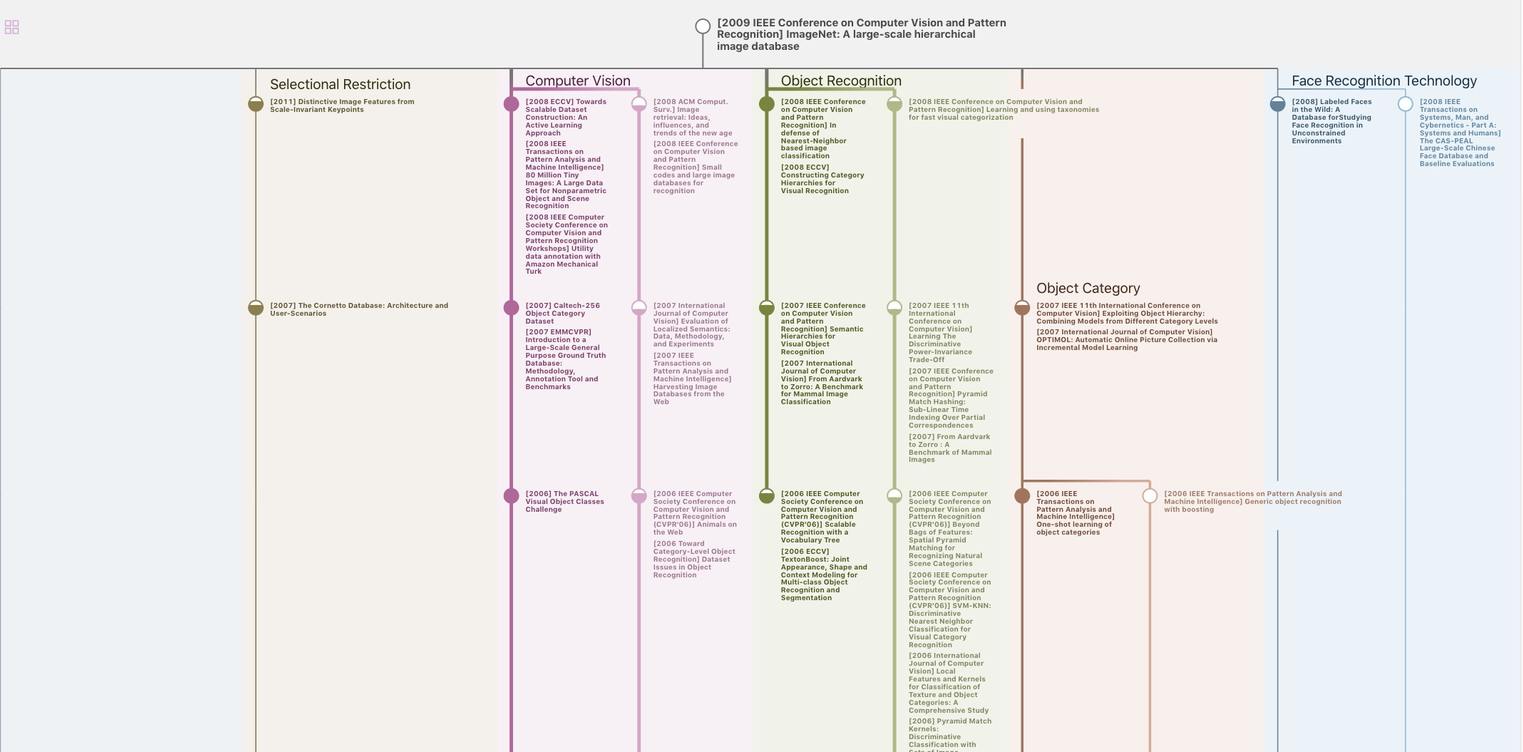
生成溯源树,研究论文发展脉络
Chat Paper
正在生成论文摘要