Grouping concentration response curves by features of their shape to aid rapid and consistent analysis of large data sets in high throughput screens.
SLAS discovery : advancing life sciences R & D(2021)
摘要
With ever increasing compound collection sizes one significant challenge in high throughput screening (HTS) is the rapid and consistent analysis of large numbers of concentration response (CR) curves. Current data analysis methods are well defined in the industry but we describe here a further addition of categorisation of concentration response data. In comparison to single point concentration data from an HTS primary screen the information generated in a concentration response curve contains much more complexity. A scientist needs to examine curves to understand their shape and features, which will determine the final summary of that compound's potency. Quite often scientists will look through every single curve individually to optimise each fit, and with increasing volumes of concentration curves being generated in HTS this can be a time-consuming task often taking days to complete. Furthermore, it is very difficult for a scientist to be consistently unbiased on decisions taken as they work through thousands of curves and it is even harder to get consistency between scientists. In this article we describe an original methodology and subsequent Genedata Screener R. extension that carries out automated classification of CR curves by applying a number of groupings and then presenting these categorised curves to the scientist for appraisal. Curves are annotated to allow them to be grouped together and ordered on increasing data quality, which acts as a guide for downstream analysis of structure activity relationships (SAR). This makes the analysis of thousands of CR curves fast and reliable leading to interpretation of HTS data being more consistent in drug discovery project teams.
更多查看译文
AI 理解论文
溯源树
样例
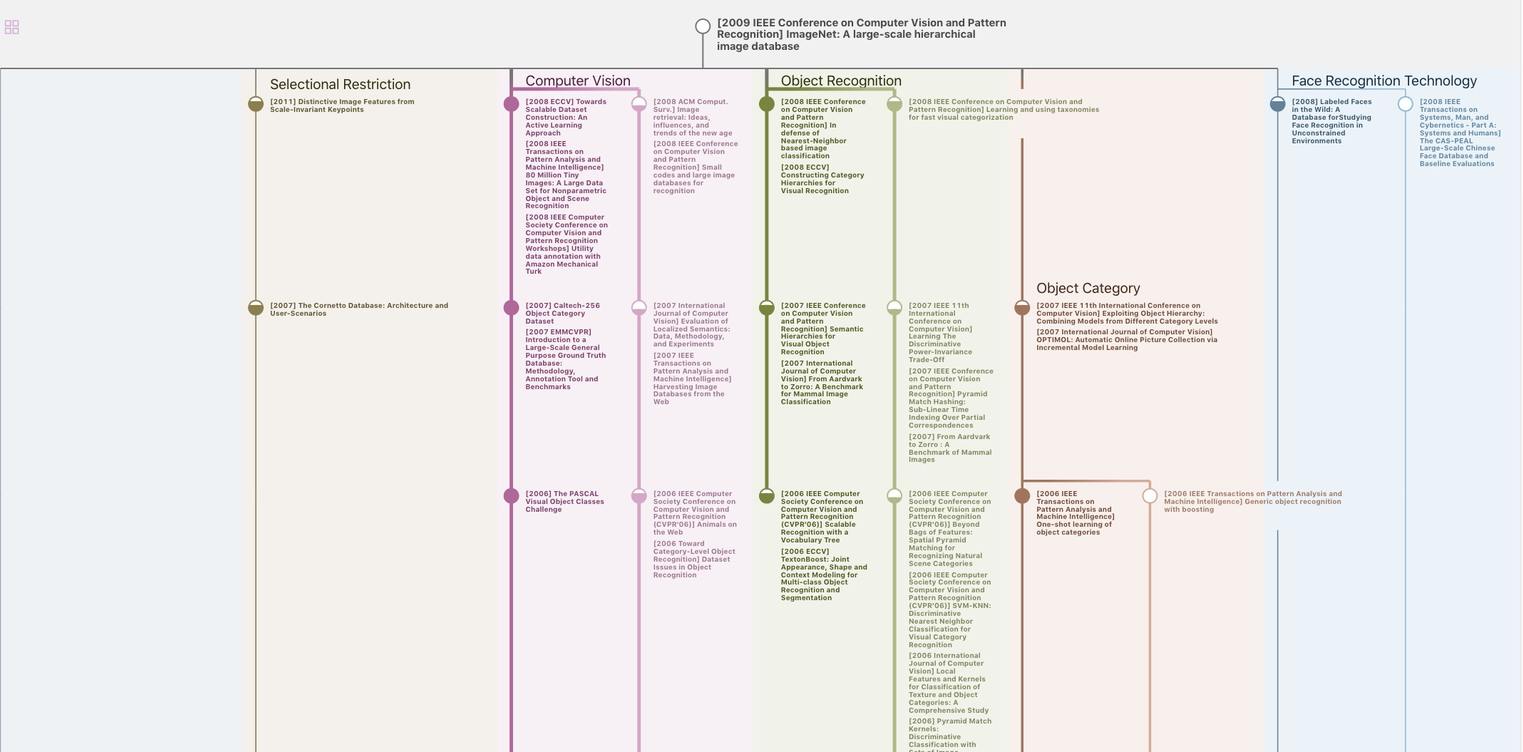
生成溯源树,研究论文发展脉络
Chat Paper
正在生成论文摘要