Automated text-level semantic markers of Alzheimer's disease.
Alzheimer's & dementia (Amsterdam, Netherlands)(2022)
摘要
INTRODUCTION:Automated speech analysis has emerged as a scalable, cost-effective tool to identify persons with Alzheimer's disease dementia (ADD). Yet, most research is undermined by low interpretability and specificity.
METHODS:Combining statistical and machine learning analyses of natural speech data, we aimed to discriminate ADD patients from healthy controls (HCs) based on automated measures of domains typically affected in ADD: semantic granularity (coarseness of concepts) and ongoing semantic variability (conceptual closeness of successive words). To test for specificity, we replicated the analyses on Parkinson's disease (PD) patients.
RESULTS:Relative to controls, ADD (but not PD) patients exhibited significant differences in both measures. Also, these features robustly discriminated between ADD patients and HC, while yielding near-chance classification between PD patients and HCs.
DISCUSSION:Automated discourse-level semantic analyses can reveal objective, interpretable, and specific markers of ADD, bridging well-established neuropsychological targets with digital assessment tools.
更多查看译文
AI 理解论文
溯源树
样例
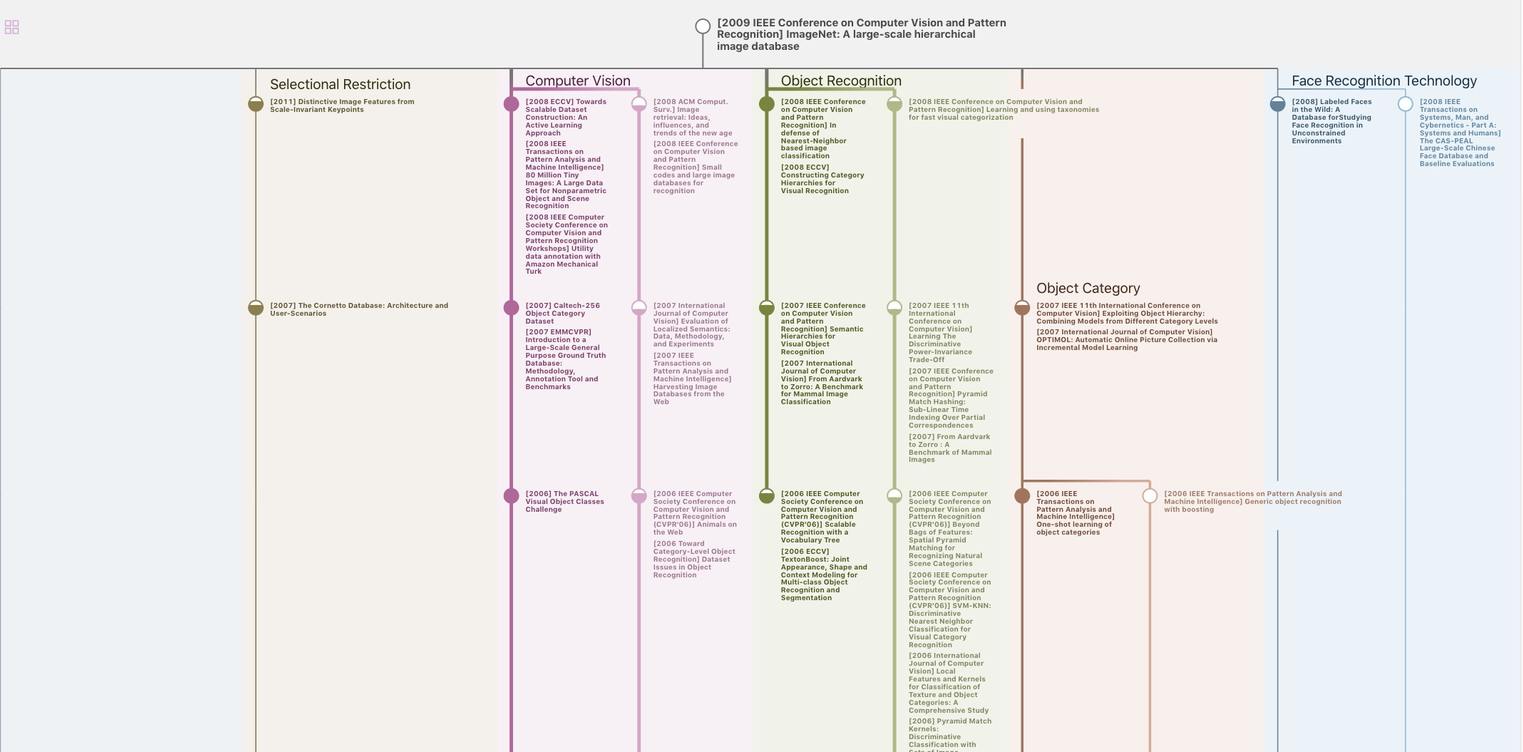
生成溯源树,研究论文发展脉络
Chat Paper
正在生成论文摘要