Performance comparison of different classification algorithms applied to the diagnosis of familial hypercholesterolemia in paediatric subjects
SCIENTIFIC REPORTS(2022)
摘要
Familial Hypercholesterolemia (FH) is an inherited disorder of lipid metabolism, characterized by increased low density lipoprotein cholesterol (LDLc) levels. The main purpose of the current work was to explore alternative classification methods to traditional clinical criteria for FH diagnosis, based on several biochemical and biological indicators. Logistic regression (LR), decision tree (DT), random forest (RF) and naive Bayes (NB) algorithms were developed for this purpose, and thresholds were optimized by maximization of Youden index (YI). All models presented similar accuracy ( Acc ), specificity ( Spec ) and positive predictive values ( PPV ). Sensitivity ( Sens ) and G -mean values were significantly higher in LR and RF models, compared to the DT. When compared to Simon Broome (SB) biochemical criteria for FH diagnosis, all models presented significantly higher Acc , Spec and G -mean values ( p < 0.01), and lower negative predictive value ( NPV , p < 0.05). Moreover, LR and RF models presented comparable Sens values. Adjustment of the cut-off point by maximizing YI significantly increased Sens values, with no significant loss in Acc . The obtained results suggest such classification algorithms can be a viable alternative to be used as a widespread screening method. An online application has been developed to assess the performance of the LR model in a wider population.
更多查看译文
关键词
Computational models,Diagnostic markers,Disease genetics,Machine learning,Predictive markers,Predictive medicine,Statistical methods,Science,Humanities and Social Sciences,multidisciplinary
AI 理解论文
溯源树
样例
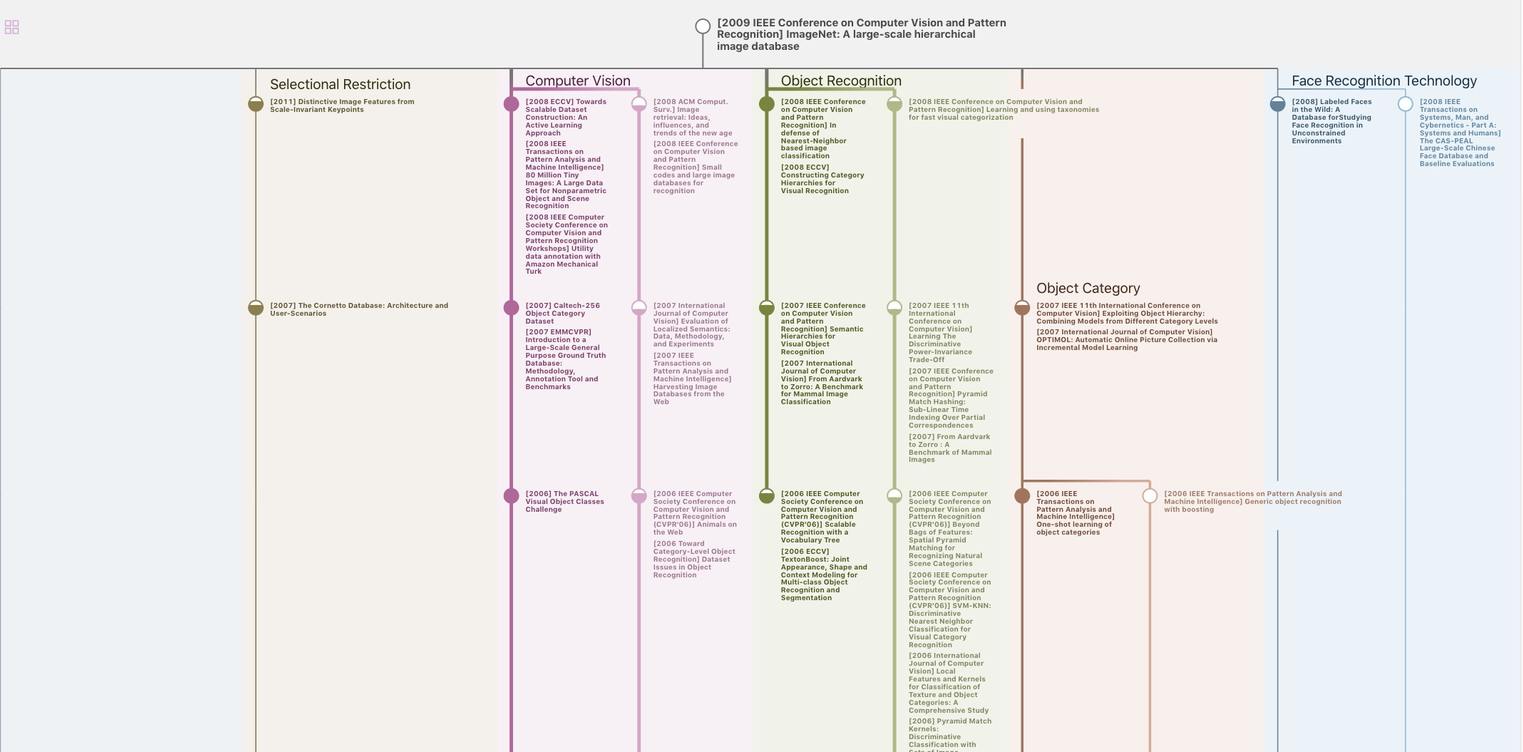
生成溯源树,研究论文发展脉络
Chat Paper
正在生成论文摘要