Simulator acceleration and inverse design of fin field-effect transistors using machine learning
SCIENTIFIC REPORTS(2022)
摘要
The simulation and design of electronic devices such as transistors is vital for the semiconductor industry. Conventionally, a device is intuitively designed and simulated using model equations, which is a time-consuming and expensive process. However, recent machine learning approaches provide an unprecedented opportunity to improve these tasks by training the underlying relationships between the device design and the specifications derived from the extensively accumulated simulation data. This study implements various machine learning approaches for the simulation acceleration and inverse-design problems of fin field-effect transistors. In comparison to traditional simulators, the proposed neural network model demonstrated almost equivalent results (R 2 = 0.99) and was more than 122,000 times faster in simulation. Moreover, the proposed inverse-design model successfully generated design parameters that satisfied the desired target specifications with high accuracies (R 2 = 0.96). Overall, the results demonstrated that the proposed machine learning models aided in achieving efficient solutions for the simulation and design problems pertaining to electronic devices. Thus, the proposed approach can be further extended to more complex devices and other vital processes in the semiconductor industry.
更多查看译文
关键词
Mathematics and computing,Nanoscience and technology,Physics,Science,Humanities and Social Sciences,multidisciplinary
AI 理解论文
溯源树
样例
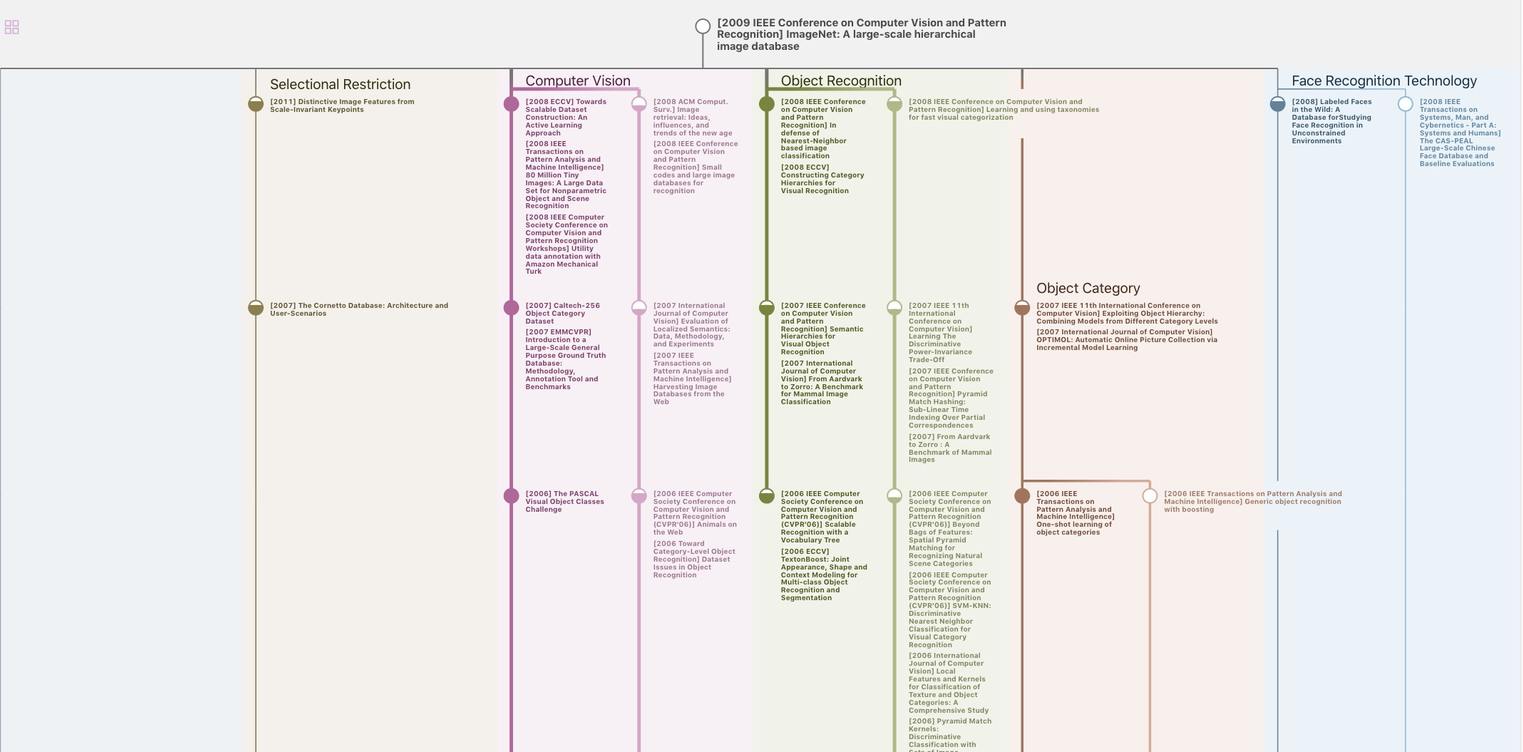
生成溯源树,研究论文发展脉络
Chat Paper
正在生成论文摘要