Unicorn: reasoning about configurable system performance through the lens of causality
European Conference on Computer Systems(2022)
摘要
ABSTRACTModern computer systems are highly configurable, with the total variability space sometimes larger than the number of atoms in the universe. Understanding and reasoning about the performance behavior of highly configurable systems, over a vast and variable space, is challenging. State-of-the-art methods for performance modeling and analyses rely on predictive machine learning models, therefore, they become (i) unreliable in unseen environments (e.g., different hardware, workloads), and (ii) may produce incorrect explanations. To tackle this, we propose a new method, called Unicorn, which (i) captures intricate interactions between configuration options across the software-hardware stack and (ii) describes how such interactions can impact performance variations via causal inference. We evaluated Unicorn on six highly configurable systems, including three on-device machine learning systems, a video encoder, a database management system, and a data analytics pipeline. The experimental results indicate that Unicorn outperforms state-of-the-art performance debugging and optimization methods in finding effective repairs for performance faults and finding configurations with near-optimal performance. Further, unlike the existing methods, the learned causal performance models reliably predict performance for new environments.
更多查看译文
关键词
Configurable Systems, Performance Modeling, Performance Debugging, Performance Optimization, Causal Inference, Counterfactual Reasoning
AI 理解论文
溯源树
样例
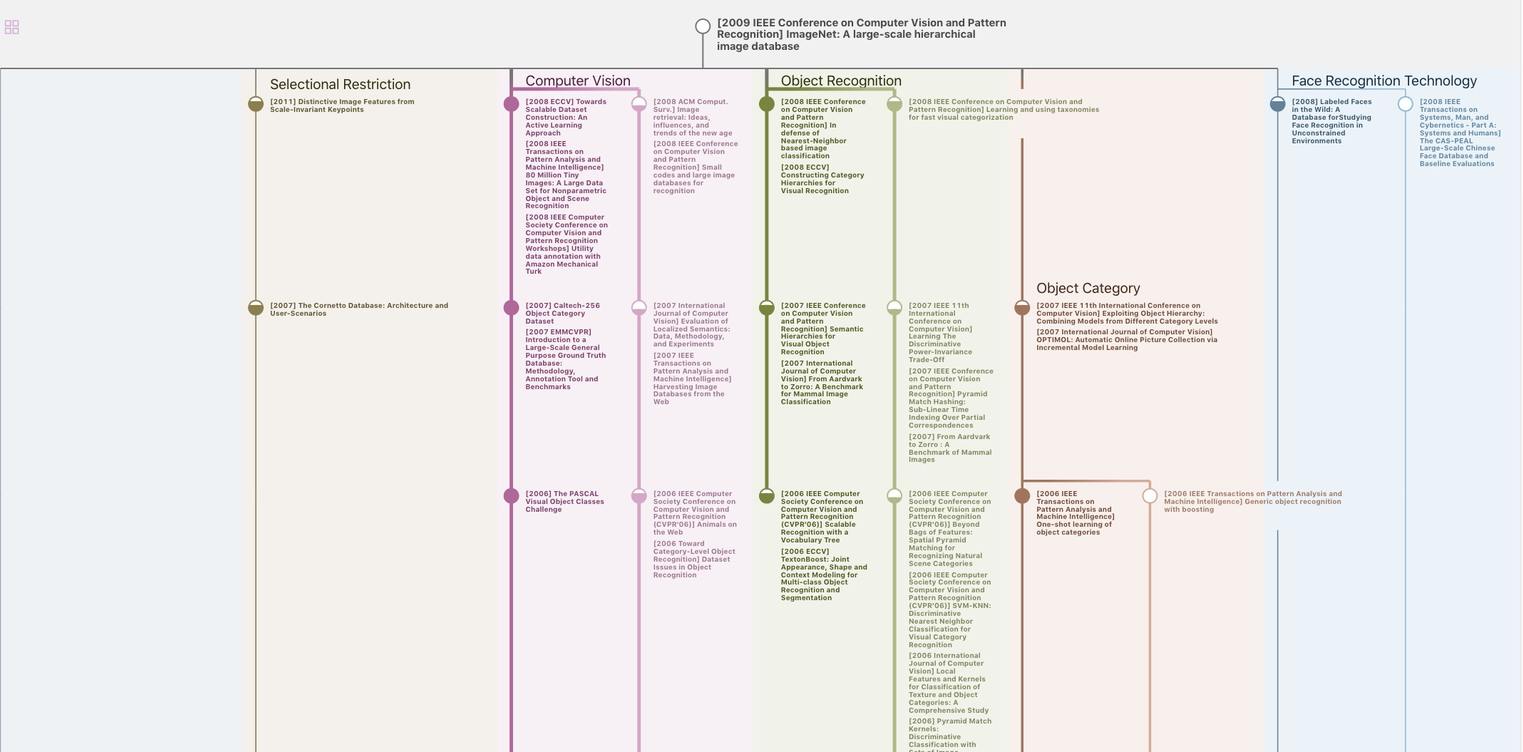
生成溯源树,研究论文发展脉络
Chat Paper
正在生成论文摘要