Human Activity Recognition models using Limited Consumer Device Sensors and Machine Learning
arxiv(2022)
摘要
Human activity recognition has grown in popularity with its increase of applications within daily lifestyles and medical environments. The goal of having efficient and reliable human activity recognition brings benefits such as accessible use and better allocation of resources; especially in the medical industry. Activity recognition and classification can be obtained using many sophisticated data recording setups, but there is also a need in observing how performance varies among models that are strictly limited to using sensor data from easily accessible devices: smartphones and smartwatches. This paper presents the findings of different models that are limited to train using such sensors. The models are trained using either the k-Nearest Neighbor, Support Vector Machine, or Random Forest classifier algorithms. Performance and evaluations are done by comparing various model performances using different combinations of mobile sensors and how they affect recognitive performances of models. Results show promise for models trained strictly using limited sensor data collected from only smartphones and smartwatches coupled with traditional machine learning concepts and algorithms.
更多查看译文
关键词
human activity recognition,machine learing
AI 理解论文
溯源树
样例
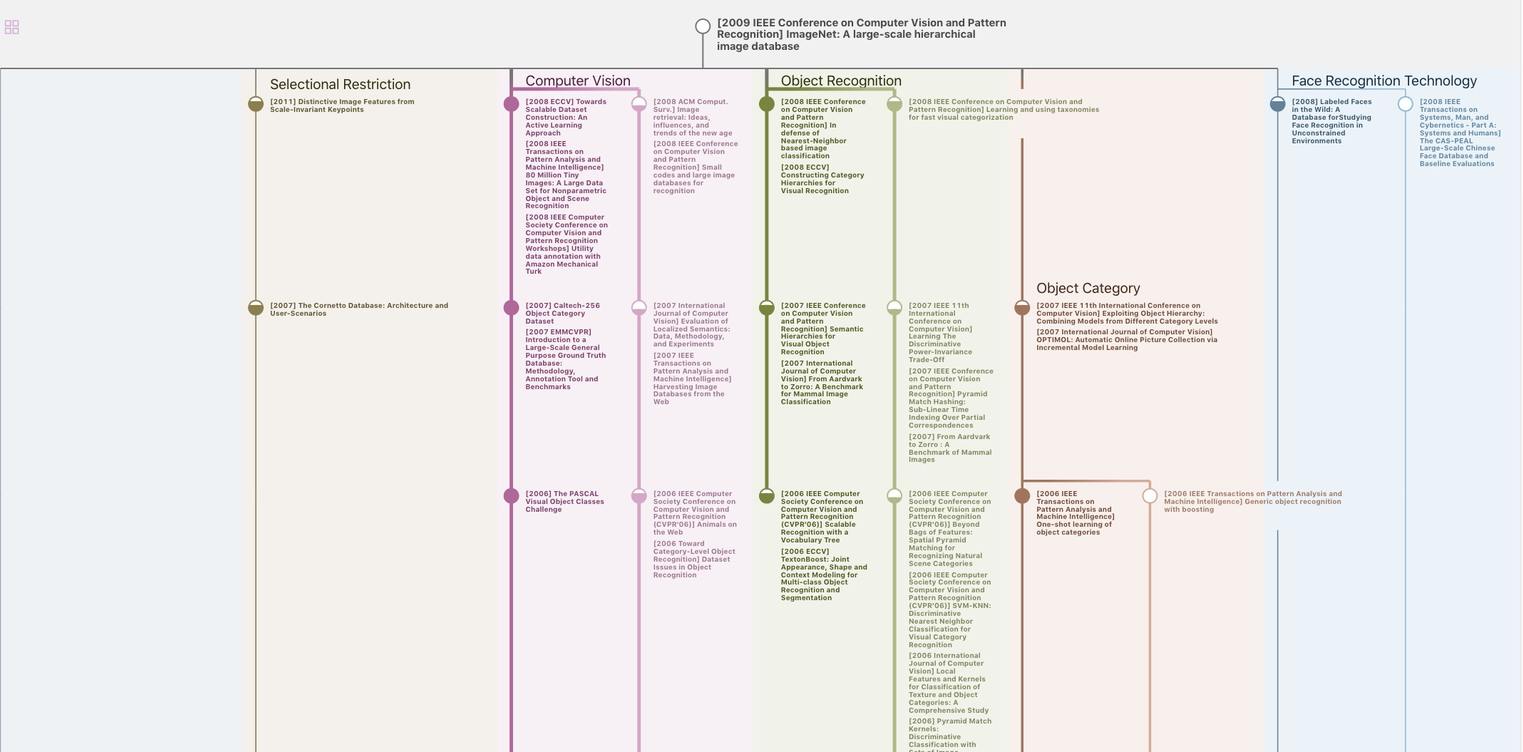
生成溯源树,研究论文发展脉络
Chat Paper
正在生成论文摘要