Under-Approximating Expected Total Rewards in POMDPs
TOOLS AND ALGORITHMS FOR THE CONSTRUCTION AND ANALYSIS OF SYSTEMS, TACAS 2022, PT II(2022)
摘要
We consider the problem: is the optimal expected total re- ward to reach a goal state in a partially observable Markov decision process (POMDP) below a given threshold? We tackle this-generally undecidable-problem by computing under-approximations on these total expected rewards. This is done by abstracting finite unfoldings of the infinite belief MDP of the POMDP. The key issue is to find a suitable under-approximation of the value function. We provide two techniques: a simple (cut-off) technique that uses a good policy on the POMDP, and a more advanced technique (belief clipping) that uses minimal shifts of probabilities between beliefs. We use mixed-integer linear programming (MILP) to find such minimal probability shifts and experimentally show that our techniques scale quite well while providing tight lower bounds on the expected total reward.
更多查看译文
AI 理解论文
溯源树
样例
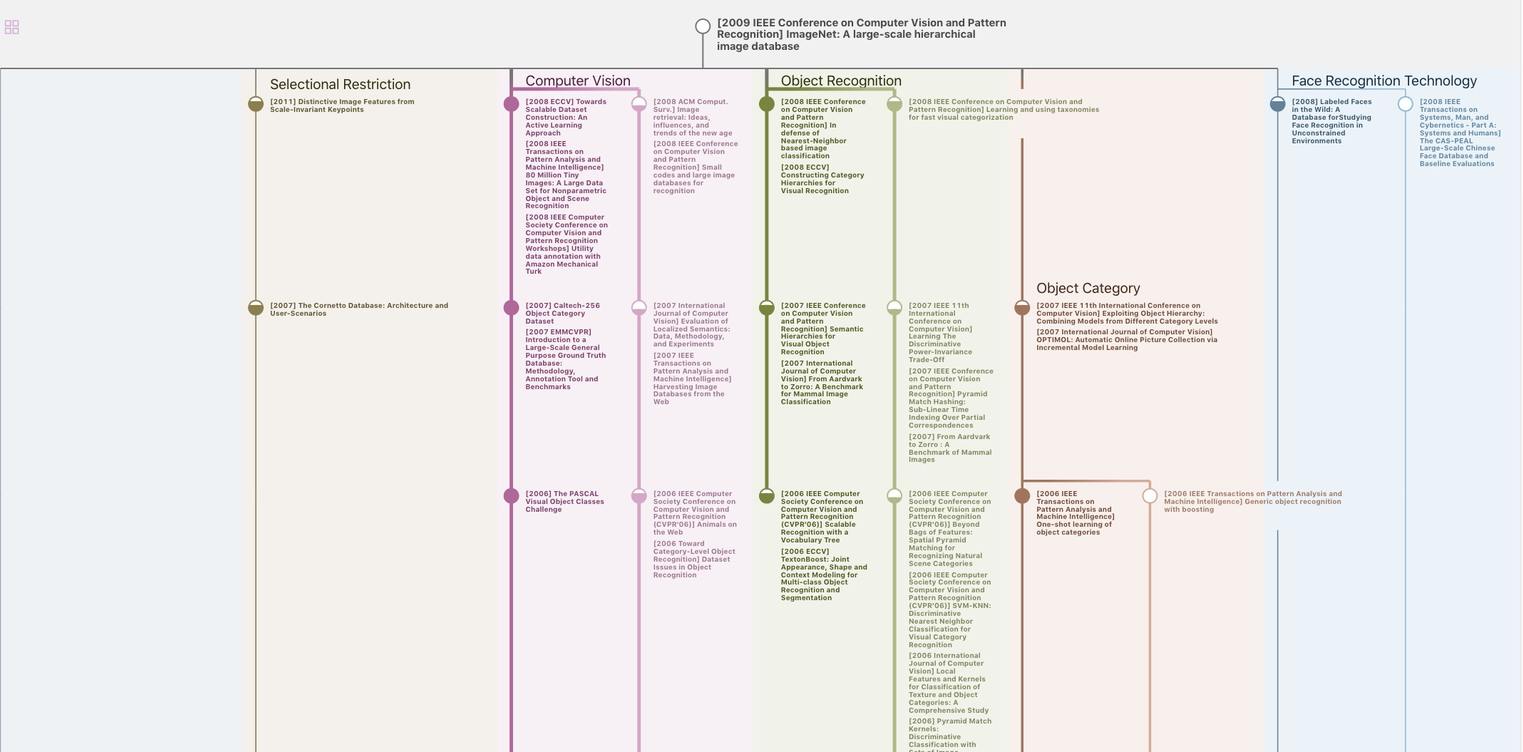
生成溯源树,研究论文发展脉络
Chat Paper
正在生成论文摘要