ReconFormer: Accelerated MRI Reconstruction Using Recurrent Transformer
IEEE TRANSACTIONS ON MEDICAL IMAGING(2024)
摘要
The accelerating magnetic resonance imaging (MRI) reconstruction process is a challenging ill-posed inverse problem due to the excessive under-sampling operation in k -space. In this paper, we propose a recurrent Transformer model, namely ReconFormer, for MRI reconstruction, which can iteratively reconstruct high-fidelity magnetic resonance images from highly under-sampled k-space data (e.g., up to 8 x acceleration). In particular, the proposed architecture is built upon Recurrent Pyramid Transformer Layers (RPTLs). The core design of the proposed method is Recurrent Scale-wise Attention (RSA), which jointly exploits intrinsic multi-scale information at every architecture unit as well as the dependencies of the deep feature correlation through recurrent states. Moreover, benefiting from its recurrent nature, ReconFormer is lightweight compared to other baselines and only contains 1.1 M trainable parameters. We validate the effectiveness of ReconFormer on multiple datasets with different magnetic resonance sequences and show that it achieves significant improvements over the state-of-the-art methods with better parameter efficiency. The implementation code and pre-trained weights are available at https://github.com/guopengf/ReconFormer.
更多查看译文
关键词
MRI,reconstruction,deep learning,transformer
AI 理解论文
溯源树
样例
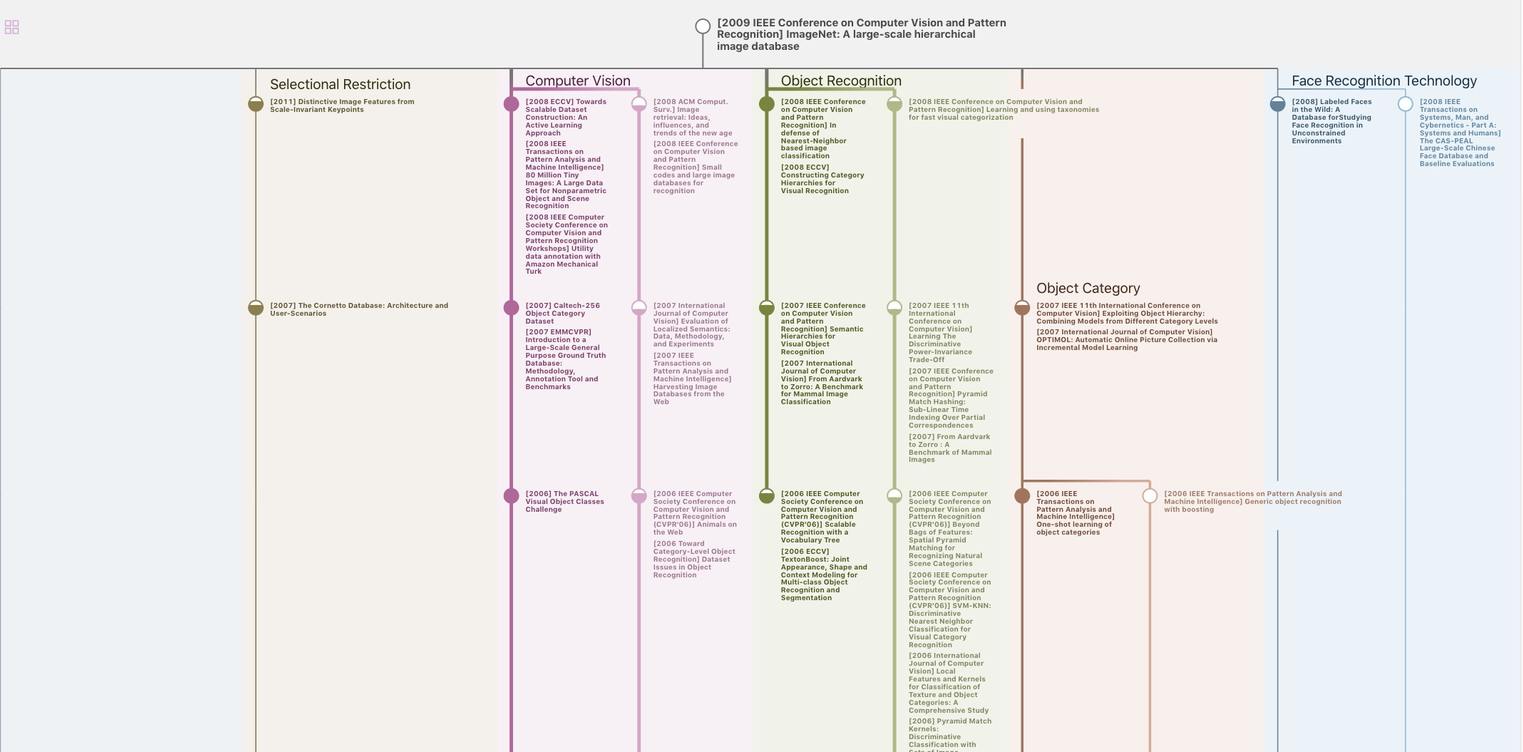
生成溯源树,研究论文发展脉络
Chat Paper
正在生成论文摘要