Analyzing Multispectral Satellite Imagery of South American Wildfires Using Deep Learning
2022 International Conference on Applied Artificial Intelligence (ICAPAI)(2022)
摘要
Since frequent severe droughts are lengthening the dry season in the Amazon Rainforest, it is important to detect wildfires promptly and forecast possible spread for effective suppression response. Current wildfire detection models are not versatile enough for the low-technology conditions of South American hot spots. This deep learning study first trains a Fully Convolutional Neural Network on Landsat 8 images of Ecuador and the Galapagos, using Green and Short-wave Infrared bands to predict pixel-level binary fire masks. This model achieves a 0.962 validation F2 score and a 0.932 F2 score on test data from Guyana and Suriname. Afterward, image segmentation is conducted on the Cirrus band using K-Means Clustering to simplify continuous pixel values into three discrete classes representing differing degrees of cirrus cloud contamination. Three additional Convolutional Neural Networks are trained to conduct a sensitivity analysis measuring the effect of simplified features on model accuracy and train time. The Experimental model trained on the segmented cirrus images provides a statistically significant decrease in train time compared to the Control model trained on raw cirrus images, without compromising binary accuracy. This proof of concept reveals that feature engineering can improve the performance of wildfire detection models by lowering computational expense.
更多查看译文
关键词
computer vision,wildfire detection,convolutional,neural networks,geospatial analysis,sensitivity analysis
AI 理解论文
溯源树
样例
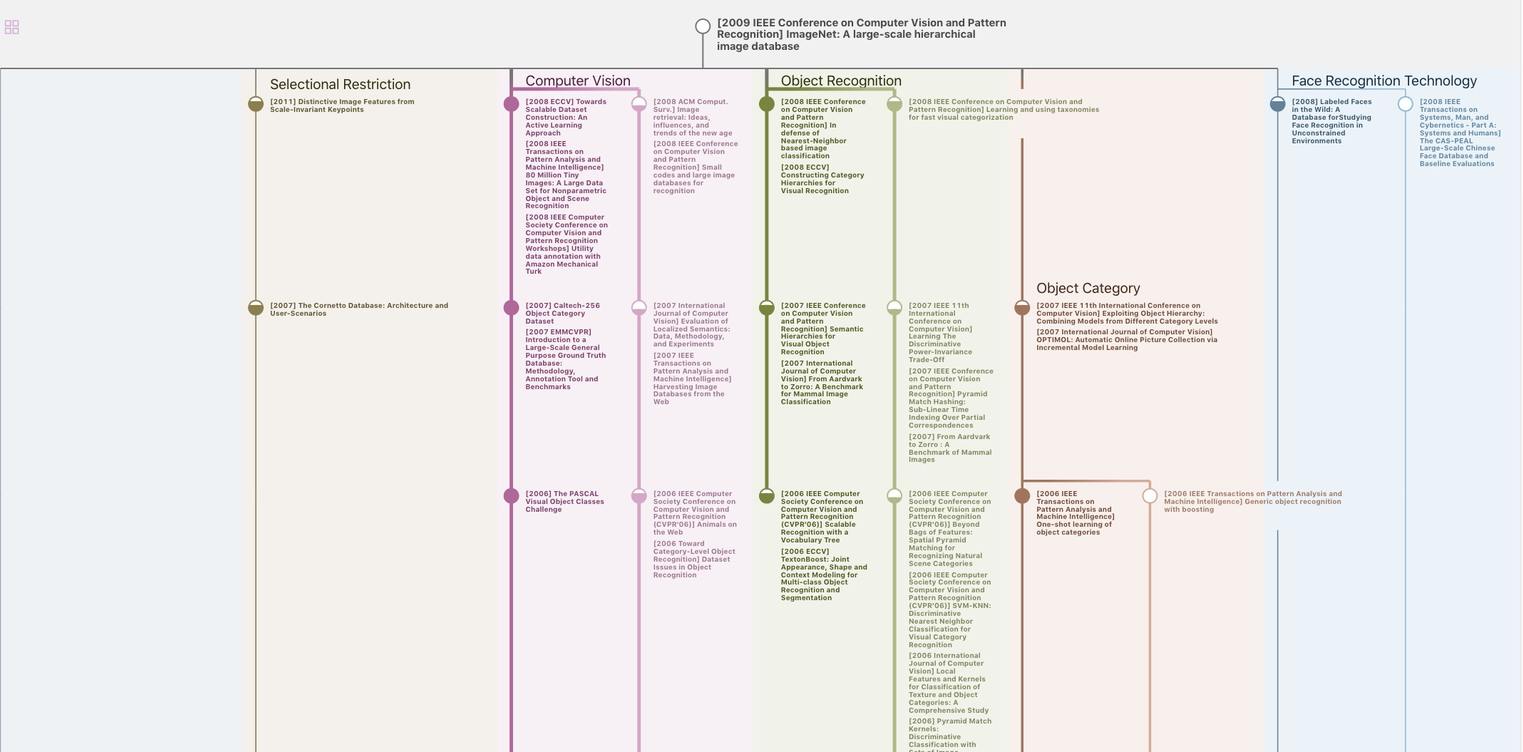
生成溯源树,研究论文发展脉络
Chat Paper
正在生成论文摘要