RSP-Hist: Approximate Histograms for Big Data Exploration on Hadoop Clusters
2021 IEEE 28th International Conference on High Performance Computing, Data, and Analytics (HiPC)(2021)
摘要
We propose a sampling-based method, called RSP-Hist, to construct approximate equi-width histograms and help data scientists explore the probability distribution of big data on Hadoop clusters. In RSP-(list, the Random Sample Partition (RSP) model is used to store a big data set as ready-to-use random sample data blocks, called RSP blocks, in the Hadoop Distributed File System (HDFS). An approximate histogram is computed by applying a sequential histogram algorithm in parallel to each block in a block-level sample of RSP blocks. Local histograms from individual RSP blocks are combined to produce an approximate histogram for the entire data. We tested RSP-Ilist on four data sets using a small computing cluster. In this paper, we demonstrate the effect of the sampling rate and the number of buckets on the histogram accuracy and show that RSP-based approximate histograms are equivalent to the exact histograms computed from the entire data. RSP-Ilist can avoid the data correlation issue in IIDFS blocks and significantly reduce both computation and communication costs. It enables iterative and interactive exploration of big data sets on small computing clusters and can be used for multivariate data exploration.
更多查看译文
关键词
Big Data,Histogram,Random Sample Partition,Data Exploration,Block-Level Sampling,Cluster Computing
AI 理解论文
溯源树
样例
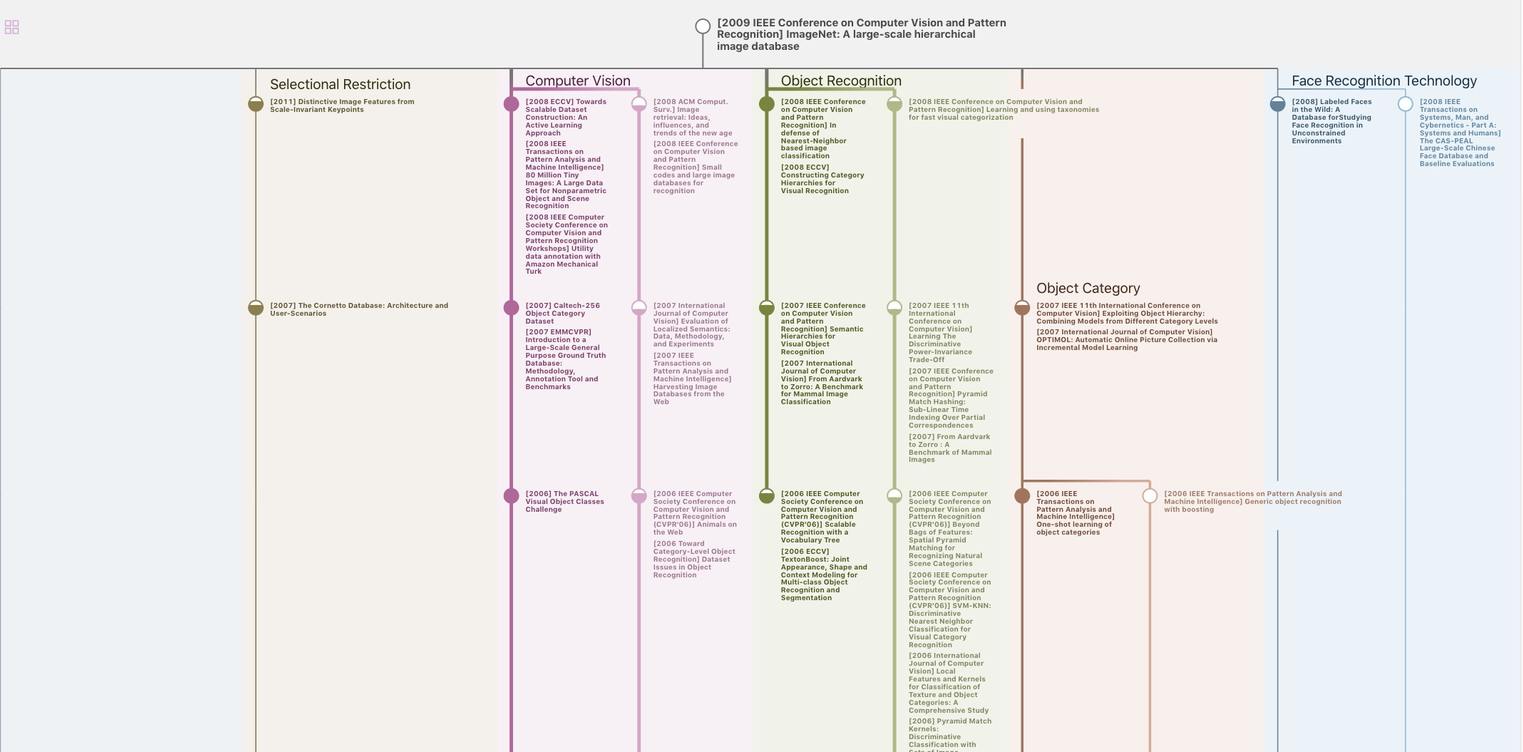
生成溯源树,研究论文发展脉络
Chat Paper
正在生成论文摘要