Variational Quantum Classifier for Binary Classification: Real vs Synthetic Dataset
IEEE ACCESS(2022)
摘要
Nowadays, quantum-enhanced methods have been widely studied to solve machine learning related problems. This article presents the application of a Variational Quantum Classifier (VQC) for binary classification. We utilized three datasets: a synthetic dataset with randomly generated values between 0 and 1, the publicly available University of California Intelligence Machine learning (UCI) sonar dataset consisting of mining data, and a proprietary diabetes dataset related to diabetes with acute diseases and diabetes without acute disease. To deal with the limitation of noisy intermediate-scale quantum systems (NISQ), we used a pre-processing method to enhance the prediction rate when applying the VQC method. The process includes feature selection and state preparation. Quantum state preparation is critical for obtaining a functioning pipeline in a quantum machine learning (QML) model. Amplitude encoding is a state preparation approach that enhances the performance of data encoding and the learning of quantum models. As a result, our proposed methods achieved accuracies of 75%, 71.4%, and 68.73% by using VQC model and in contrast, the amplitude encoding-based VQC achieved 98.40%, 67.3%, and 74.50% accuracies on the synthetic, sonar, and diabetes dataset, respectively.
更多查看译文
关键词
Diabetes, Encoding, Sonar, Computational modeling, Feature extraction, Rocks, Quantum state, Quantum machine learning, state preparation, amplitude encoding, variational quantum classifier and T2DM diabetes
AI 理解论文
溯源树
样例
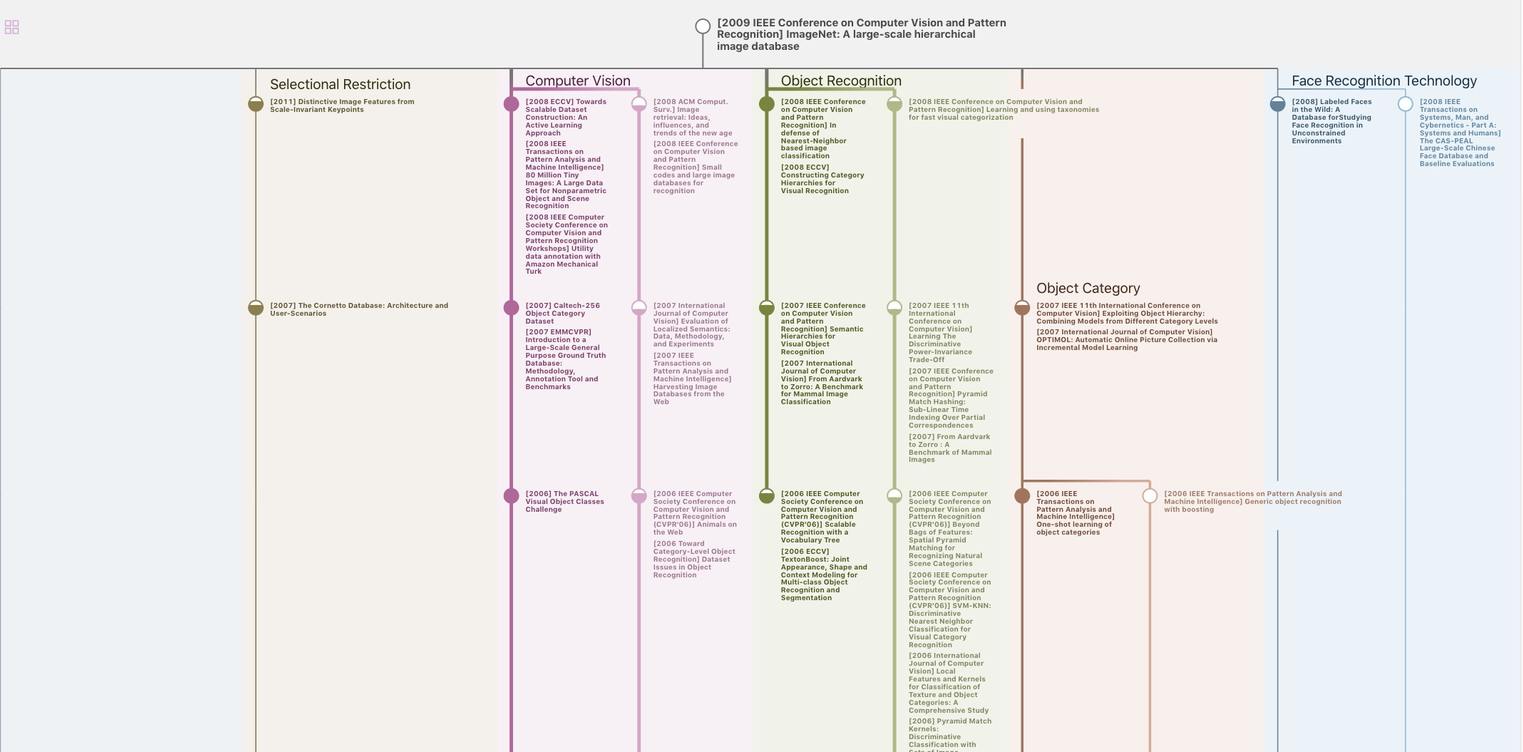
生成溯源树,研究论文发展脉络
Chat Paper
正在生成论文摘要