Deep Reinforcement Learning With Adversarial Training for Automated Excavation Using Depth Images
IEEE ACCESS(2022)
摘要
Excavation, which is one of the most frequently performed tasks during construction often poses danger to human operators. To reduce potential risks and address the problem of workforce shortage, automation of excavation is essential. Although previous studies have yielded promising results based on the use of reinforcement learning (RL) for automated excavation, the properties of excavation task in the context of RL have not been sufficiently investigated. In this study, we investigate Qt-Opt, which is a variant of Q-learning algorithms for continuous action space, for learning the excavation task using depth images. Inspired by virtual adversarial training in supervised learning, we propose a regularization method that uses virtual adversarial samples to reduce overestimation of Q-values in a Q-learning algorithm. Our results reveal that Qt-Opt is more sample-efficient than state-of-the-art actor-critic methods in our problem setting, and we verify that the proposed method further improves the sample efficiency of Qt-Opt. Our results demonstrate that multiple optimal actions often exist within the process of excavation and the choice of policy representation is crucial for satisfactory performance.
更多查看译文
关键词
Excavation, Task analysis, Training, Q-learning, Automation, Licenses, Approximation algorithms, Construction industry, automation, machine learning, reinforcement learning
AI 理解论文
溯源树
样例
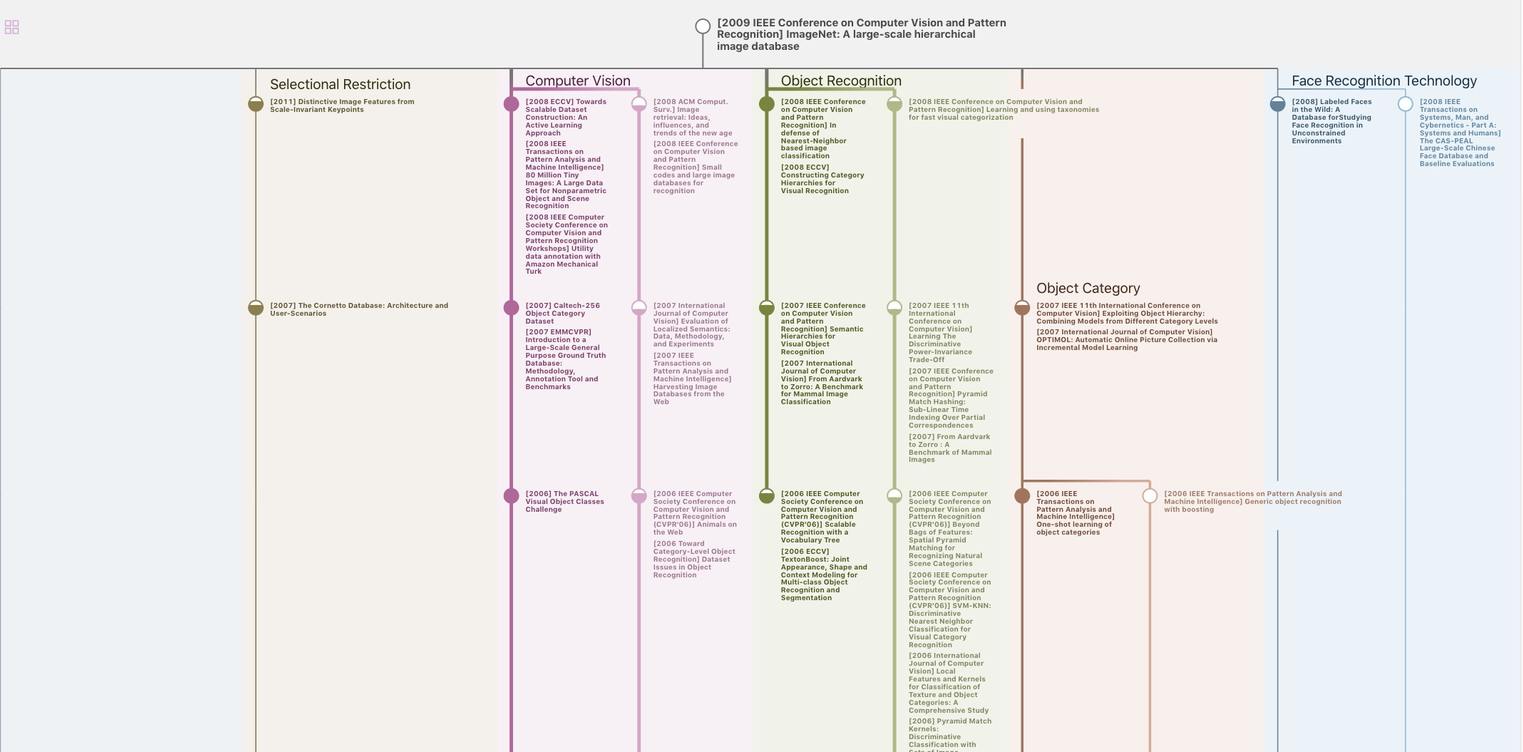
生成溯源树,研究论文发展脉络
Chat Paper
正在生成论文摘要