Predicting high-resolution turbulence details in space and time
ACM Transactions on Graphics(2021)
摘要
AbstractPredicting the fine and intricate details of a turbulent flow field in both space and time from a coarse input remains a major challenge despite the availability of modern machine learning tools. In this paper, we present a simple and effective dictionary-based approach to spatio-temporal upsampling of fluid simulation. We demonstrate that our neural network approach can reproduce the visual complexity of turbulent flows from spatially and temporally coarse velocity fields even when using a generic training set. Moreover, since our method generates finer spatial and/or temporal details through embarrassingly-parallel upsampling of small local patches, it can efficiently predict high-resolution turbulence details across a variety of grid resolutions. As a consequence, our method offers a whole range of applications varying from fluid flow upsampling to fluid data compression. We demonstrate the efficiency and generalizability of our method for synthesizing turbulent flows on a series of complex examples, highlighting dramatically better results in spatio-temporal upsampling and flow data compression than existing methods as assessed by both qualitative and quantitative comparisons.
更多查看译文
关键词
Fluid Simulation, Dictionary Learning, Neural Networks, Smoke Animation
AI 理解论文
溯源树
样例
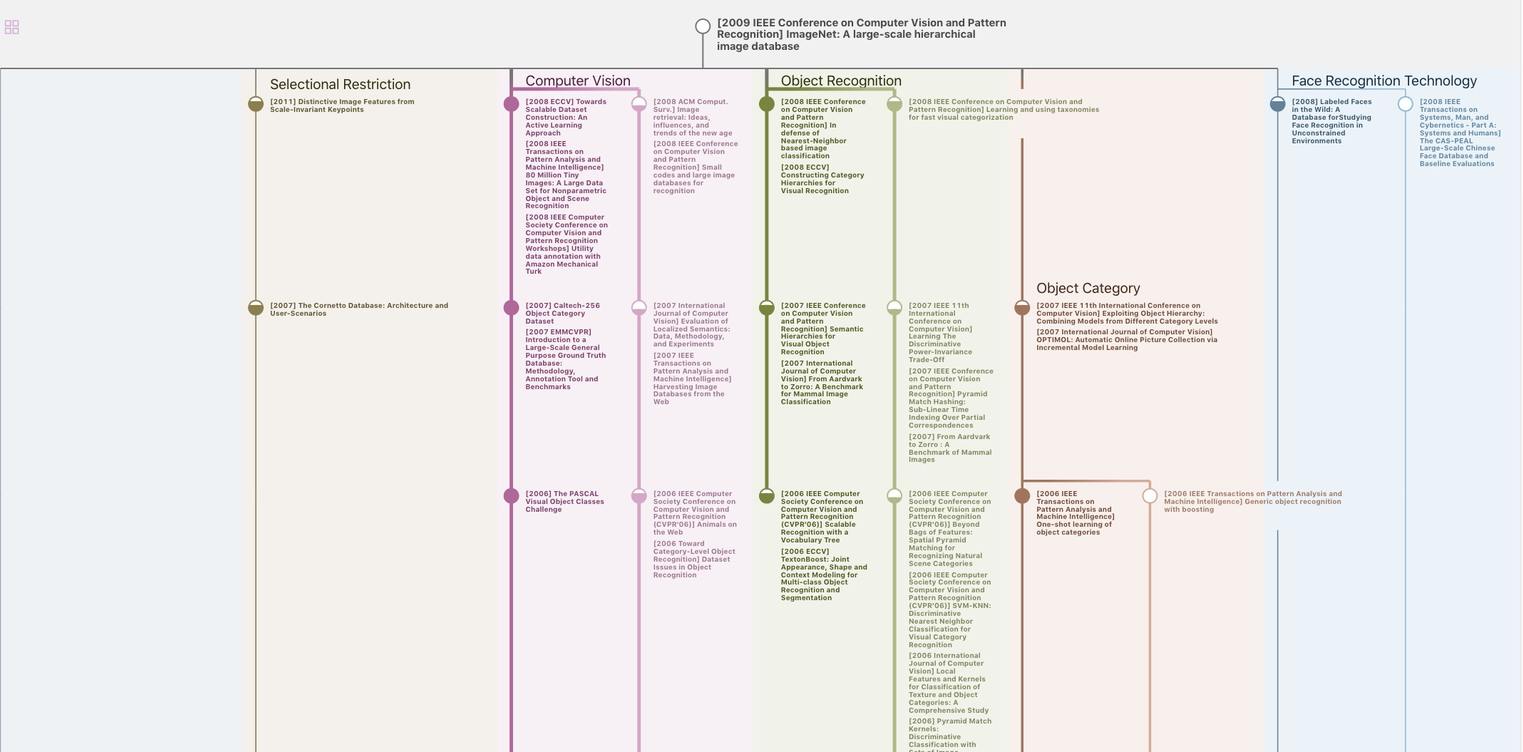
生成溯源树,研究论文发展脉络
Chat Paper
正在生成论文摘要