LIME-Based Data Selection Method for SAR Images Generation Using GAN
REMOTE SENSING(2022)
摘要
Deep learning has obtained remarkable achievements in computer vision, especially image and video processing. However, in synthetic aperture radar (SAR) image recognition, the application of DNNs is usually restricted due to data insufficiency. To augment datasets, generative adversarial networks (GANs) are usually used to generate numerous photo-realistic SAR images. Although there are many pixel-level metrics to measure GAN's performance from the quality of generated SAR images, there are few measurements to evaluate whether the generated SAR images include the most representative features of the target. In this case, the classifier probably categorizes a SAR image into the corresponding class based on "wrong" criterion, i.e., "Clever Hans". In this paper, local interpretable model-agnostic explanation (LIME) is innovatively utilized to evaluate whether a generated SAR image possessed the most representative features of a specific kind of target. Firstly, LIME is used to visualize positive contributions of the input SAR image to the correct prediction of the classifier. Subsequently, these representative SAR images can be selected handily by evaluating how much the positive contribution region matches the target. Experimental results demonstrate that the proposed method can ally "Clever Hans" phenomenon greatly caused by the spurious relationship between generated SAR images and the corresponding classes.
更多查看译文
关键词
generative adversarial networks (GAN), synthetic aperture radar (SAR), deep neural network understanding, target interpretation and recognition, local interpretable model-agnostic explanation (LIME)
AI 理解论文
溯源树
样例
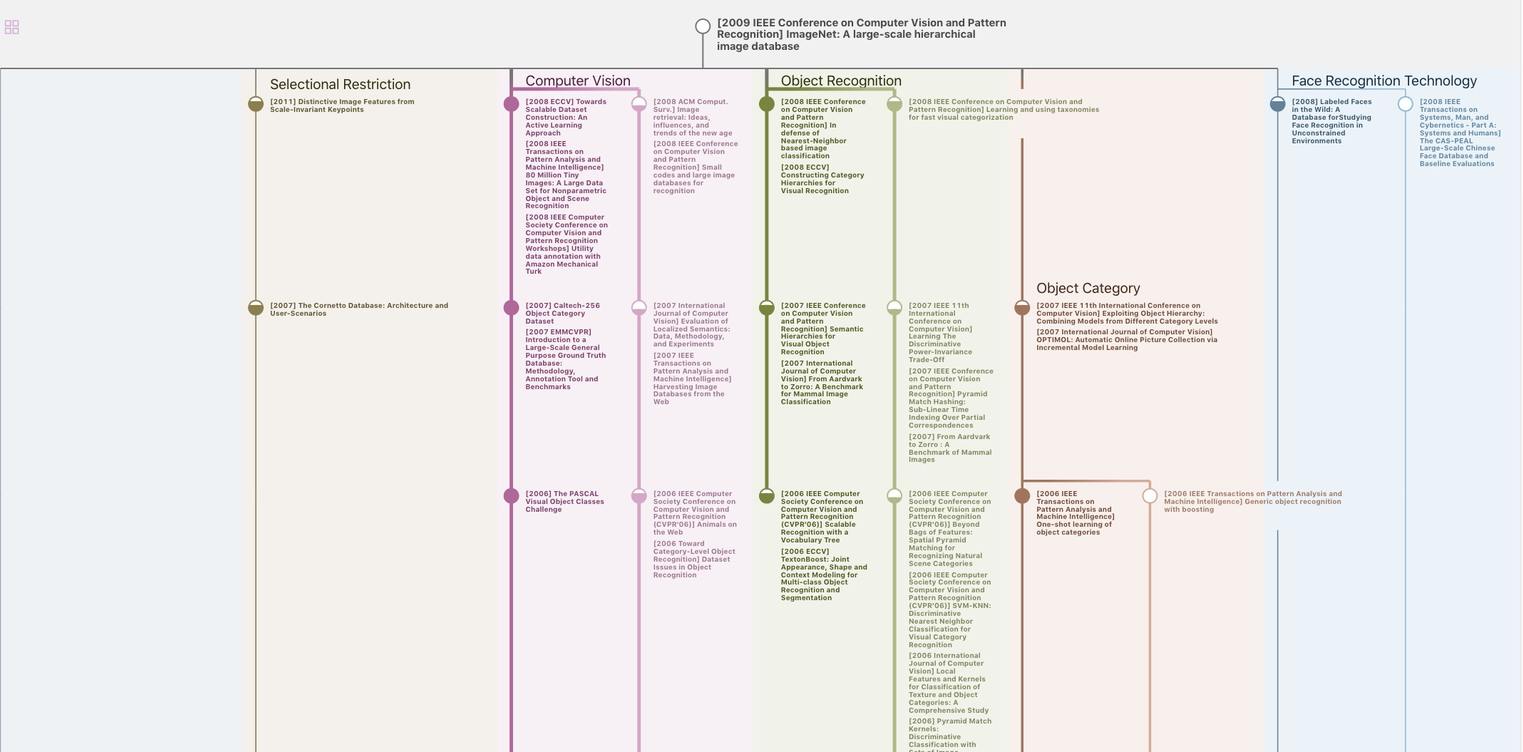
生成溯源树,研究论文发展脉络
Chat Paper
正在生成论文摘要