SGML: A Symmetric Graph Metric Learning Framework for Efficient Hyperspectral Image Classification
IEEE Journal of Selected Topics in Applied Earth Observations and Remote Sensing(2022)
摘要
Recently, the semi-supervised graph convolutional network (SSGCN) has been verified effective for hyperspectral image (HSI) classification. However, constrained by the limited training data and spectral uncertainty, the classification performance is remained to be further improved. Moreover, attribute to the massive data, the SSGCN with complex computation is generally too time- and resource-consuming to be applicable in real-time needs. To conquer these issues, we propose an efficient symmetric graph metric learning (SGML) framework by incorporating metric learning into the SSGCN paradigm. Specifically, we first conduct multilevel pixel-to-superpixel projection (P-SP) on the HSI to investigate the multiscale spatial information, where the suitable superpixel numbers are adaptively determined. Then, to extract more expressive representations, we design a new structure denoted as GSvolution, comprising the graph convolution (G-Conv) and a novel self-channel-enhanced convolution (S-Conv), to propagate the labeled and unlabeled graph node information and simultaneously enhance the critical intranode channel features. Finally, the superpixel node features are reprojected to the pixel level (SP-P) so that the distilled multistream features can be integrated to obtain the final decision. Noticeably, this ingenious symmetric mechanism (P-SP and SP-P) can alleviate the spectral variability and facilitate the framework to be an efficient model. Furthermore, in the metric learning module, we propose an innovative metric loss function to enhance the discrimination of the embedding features, i.e., inter class far apart and intraclass close. In the experiments, we demonstrate that the classification capacity of the proposed SGML can surpass the comparators on three benchmark data sets.
更多查看译文
关键词
Graph convolutional network,HSI classification,metric learning,multilevel superpixel segmentation
AI 理解论文
溯源树
样例
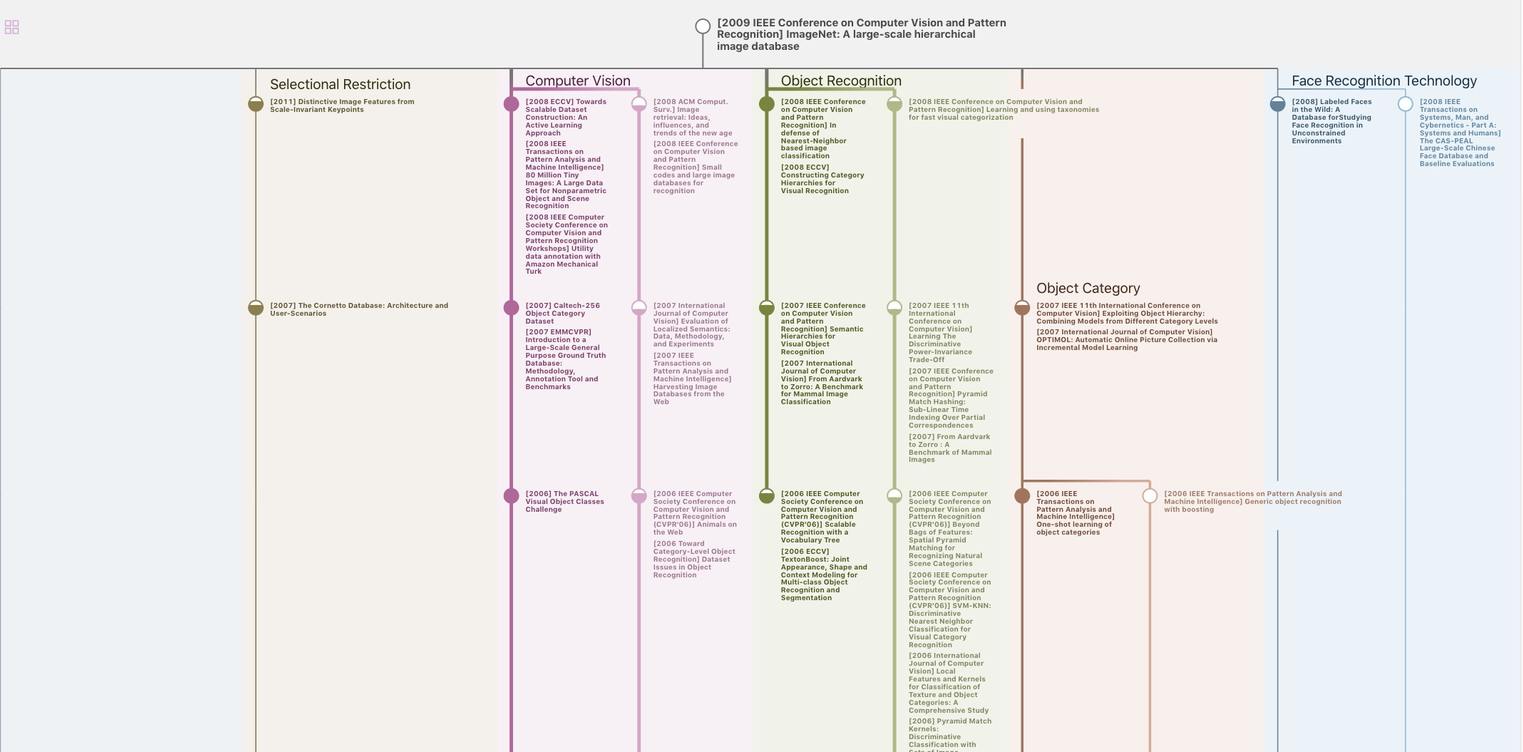
生成溯源树,研究论文发展脉络
Chat Paper
正在生成论文摘要