Shared Trained Models Selection and Management for Transfer Reinforcement Learning in Open IoT.
SMC(2021)
摘要
This paper proposes a new paradigm that utilizes trained models shared by multiple systems and also proposes a mandatory method to achieve it. Reinforcement learning and transfer learning are powerful technologies to automatically build systems composed of shared devices with various combinations of installation positions and device types. However, current works are premised on an individual system, and learning knowledge is not shared across multiple systems. Our proposed method enables systems to select and transfer an appropriate trained model from shared models across multiple systems. The trained model is selected on the basis of the similarity of input data of learning. The proposed method enables individual systems to complete learning with a small number of demonstrations while maintaining an appropriate number of trained models in the learning model pool. Moreover, since the proposed method does not depend on a specific learning algorithm, it can be applied to various systems. In the simulation of the robot navigation task, the selection of the transfer model by the proposed method increased the sum of rewards by 32% compared with randomly selecting the transfer model. In addition, in experiments on multiple successive learning requests, the proposed method updated the learning model pool and increased the sum of rewards of all systems by 34% compared with the case without transfer learning.
更多查看译文
关键词
Internet of Things,reinforcement learning,transfer learning,sensors,robot
AI 理解论文
溯源树
样例
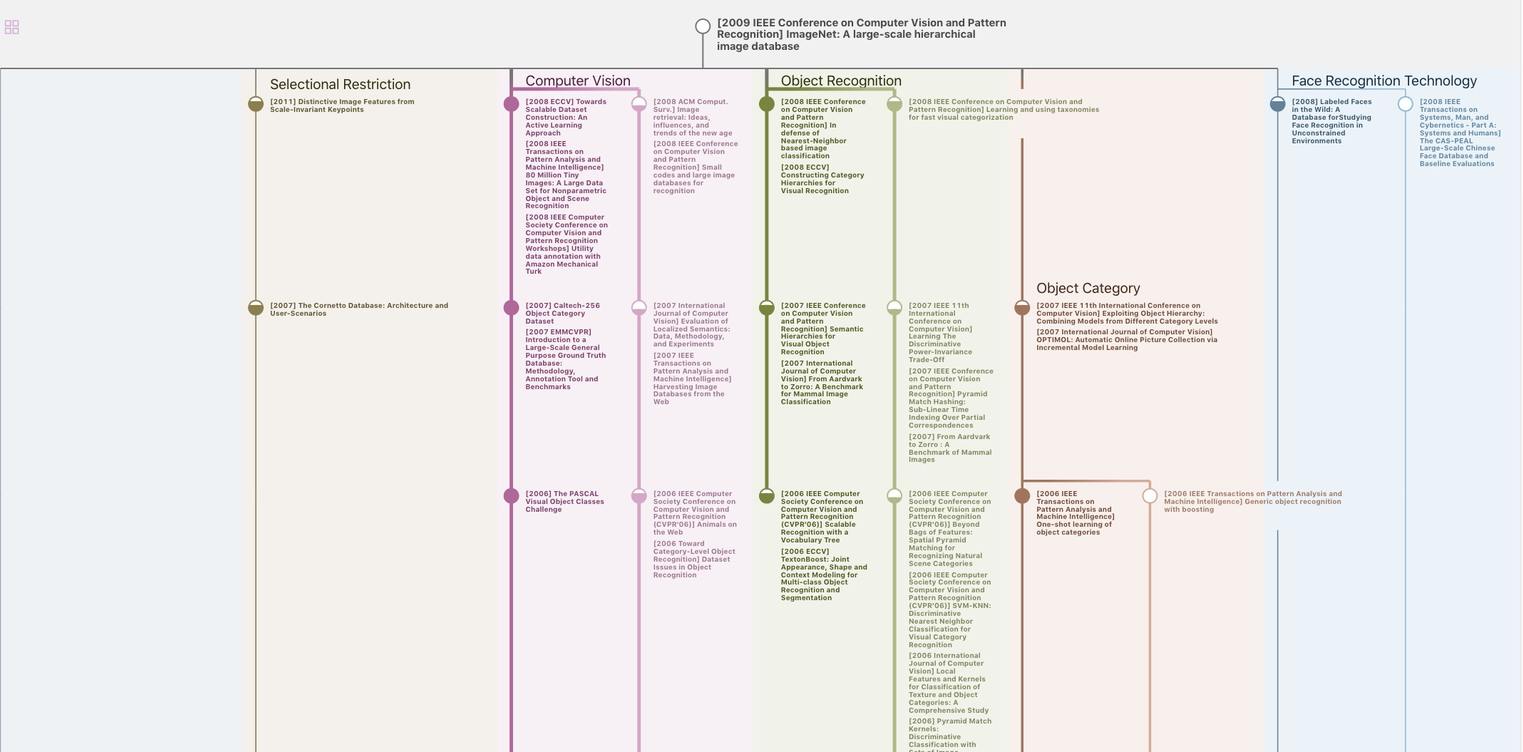
生成溯源树,研究论文发展脉络
Chat Paper
正在生成论文摘要