Towards Building a Flexible Online Learning Model for Data Stream Classification.
COMAD/CODS(2022)
摘要
In the current era, data are generated by almost every electronic devices. These data are often produced continuously from many IoT devices resulting in an enormous corpus of data streams. To learn and model such data streams, an adaptive, robust and scalable learning algorithm is needed which can handle concept drift and also able to retain previously learned knowledge. In real-world applications, labeling every data item in data streams is time and resource consuming. Therefore, in this work, we propose a self-evolving online learning algorithm that not only efficiently learn the data streams under limited labeled data conditions but also handle concept drift and retain the previously learned knowledge by evolving its network structure on-demand and from scratch. For experimental purpose, we have utilized a publicly available streaming data having i) massive and highly imbalanced network intrusion detection and, ii) binary and multi-class classification problem. Our results show the network evolution and adaption to obtain a test accuracy of above 99% for a least of 1% of labeled data.
更多查看译文
AI 理解论文
溯源树
样例
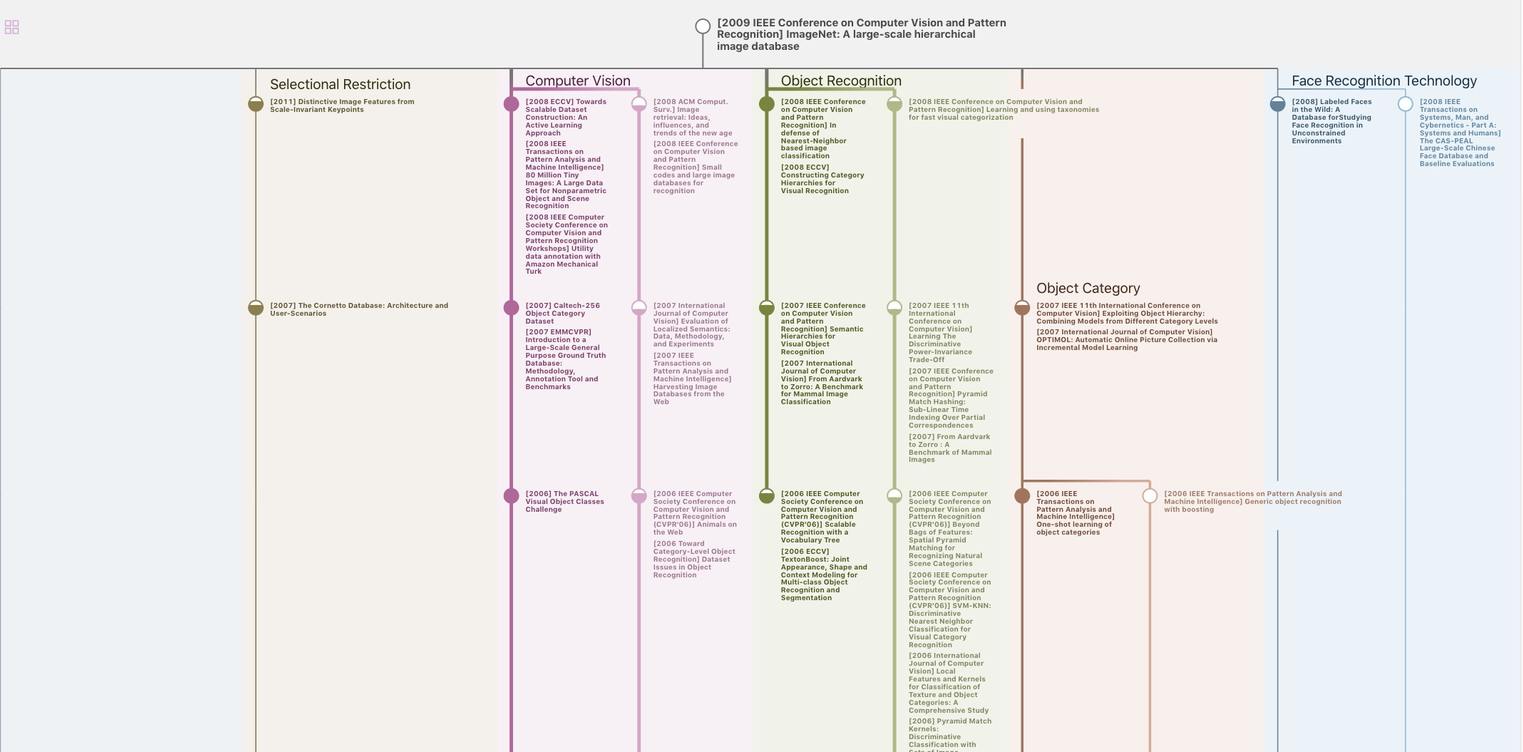
生成溯源树,研究论文发展脉络
Chat Paper
正在生成论文摘要