Mining On-shelf High-utility Quantitative Itemsets.
IEEE BigData(2021)
摘要
A recently emerged branch of utility-based research, called high-utility quantitative itemset mining (HUQIM), has been widely applied in real-life, and it considers not only the utility factor but also the quantity with ranges of itemsets. However, most existing utility-mining algorithms assume that patterns always appear regardless of the period. For instance, some products may sell well at certain times of the year. Considering the rich information in the database, such as quantity and time, we propose an effective and efficient approach for discovering on-shelf high-utility quantitative itemsets (OHUQIs). To avoid scanning the database multiple times, we adopt a data structure to maintain some necessary information, and thus, OHUQI only accesses the database twice. Several pruning strategies are also designed to prune a large number of unpromising itemsets in advance to shrink the search space. Finally, the subsequent experimental results show that OHUQI performs well on several real-world datasets.
更多查看译文
关键词
utility mining,high-utility itemset,quantitative information,on-shelf data
AI 理解论文
溯源树
样例
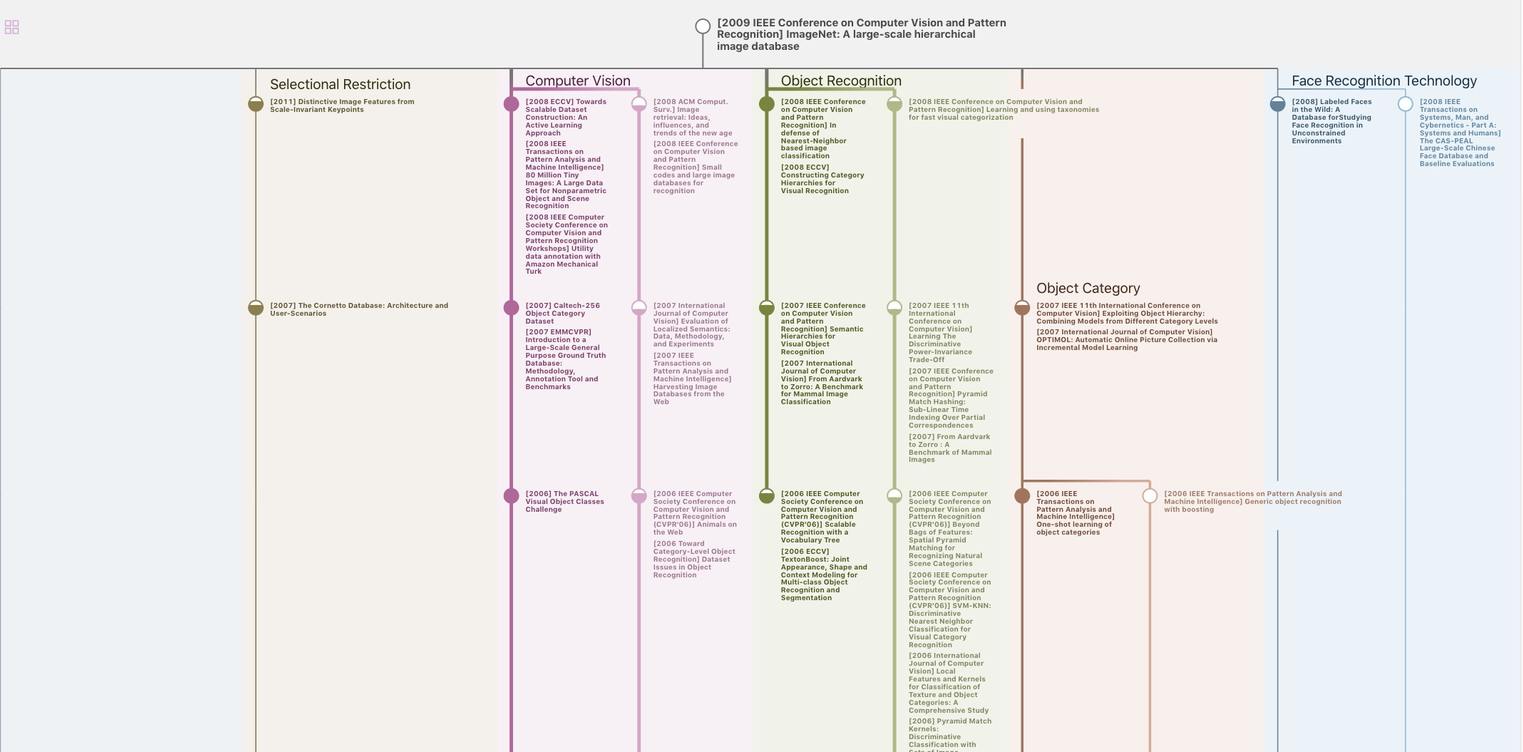
生成溯源树,研究论文发展脉络
Chat Paper
正在生成论文摘要