Event-based Product Carousel Recommendation with Query-Click Graph
2021 IEEE INTERNATIONAL CONFERENCE ON BIG DATA (BIG DATA)(2021)
摘要
Many current recommender systems mainly focus on the product-to-product recommendations and user-to-product recommendations even during the time of events rather than modeling the typical recommendations for the target event (e.g., festivals, seasonal activities, or social activities) without addressing the multiple aspects of the shopping demands for the target event. Product recommendations for the multiple aspects of the target event are usually generated by human curators who manually identify the aspects and select a list of aspect-related products (i.e., product carousel) for each aspect as recommendations. However, building a recommender system with machine learning is non-trivial due to the lack of both the ground truth of event-related aspects and the aspect-related products. To fill this gap, we define the novel problem as the event-based product carousel recommendations in e-commerce and propose an effective recommender system based on the query-click bipartite graph. We apply the iterative clustering algorithm over the query-click bipartite graph and infer the event-related aspects by the clusters of queries. The aspect-related recommendations are powered by the click-through rate of products regarding each aspect. We show through experiments that this approach effectively mines product carousels for the target event.
更多查看译文
关键词
product carousel recommendations,clustering,topic mining,bipartite graph
AI 理解论文
溯源树
样例
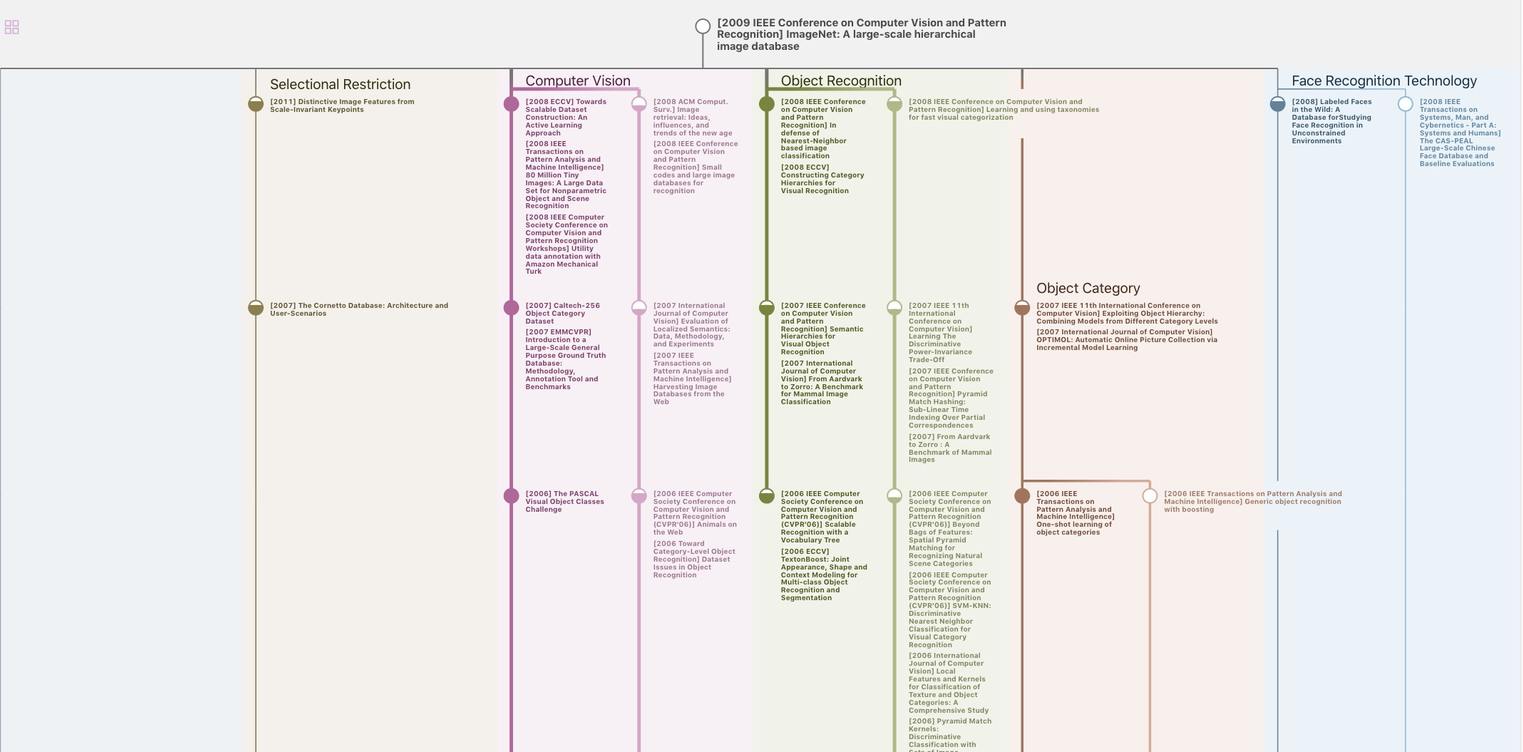
生成溯源树,研究论文发展脉络
Chat Paper
正在生成论文摘要