Evaluating Model Robustness to Adversarial Samples in Network Intrusion Detection.
IEEE BigData(2021)
摘要
Adversarial machine learning, a technique which seeks to deceive machine learning (ML) models, threatens the utility and reliability of ML systems. This is particularly relevant in critical ML implementations such as those found in Network Intrusion Detection Systems (NIDS). This paper considers the impact of adversarial influence on NIDS and proposes ways to improve ML based systems. Specifically, we consider five feature robustness metrics to determine which features in a model are most vulnerable, and four defense methods. These methods are tested on six ML models with four adversarial sample generation techniques. Our results show that across different models and adversarial generation techniques, there is limited consistency in vulnerable features or in effectiveness of defense method.
更多查看译文
关键词
machine learning,network security,adversarial artificial intelligence,model robustness evaluation
AI 理解论文
溯源树
样例
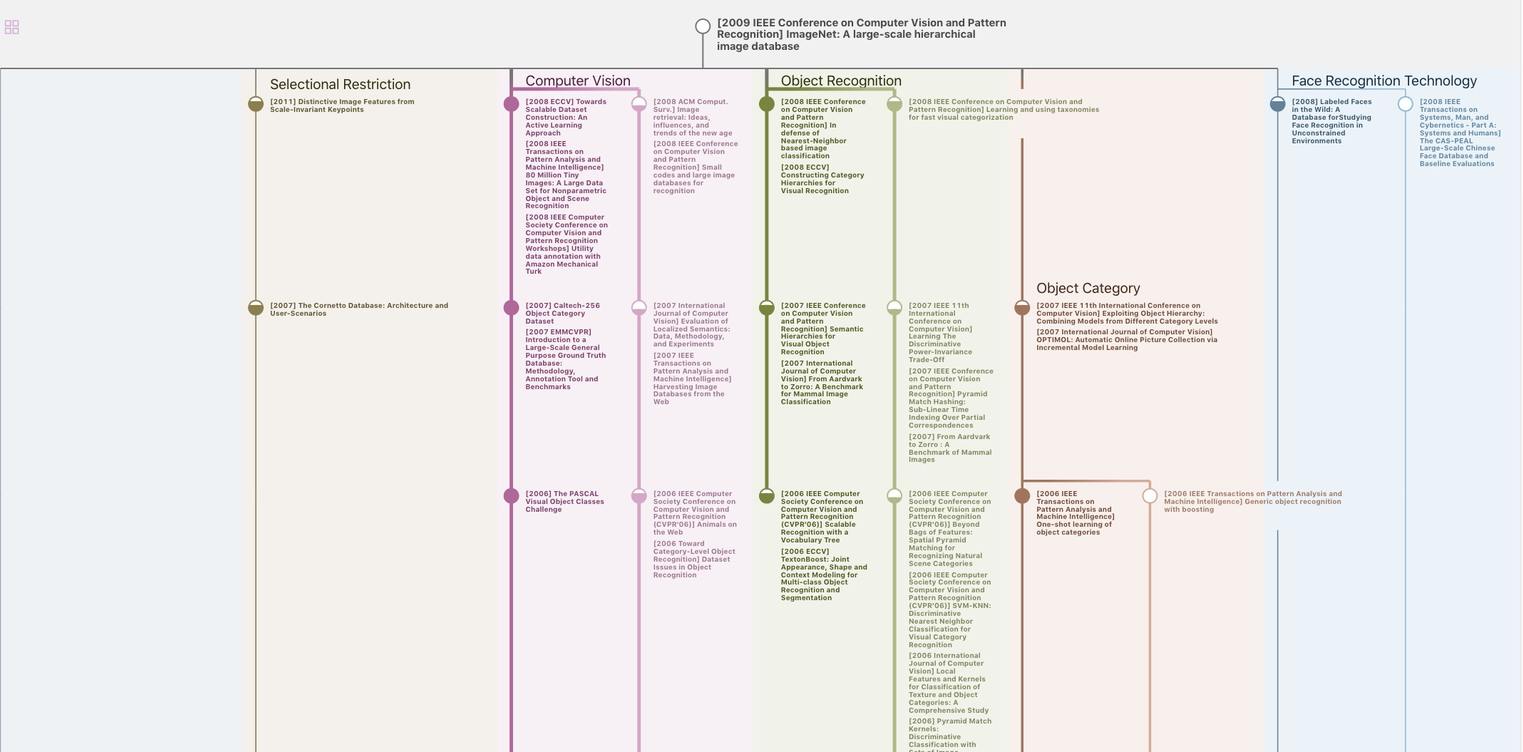
生成溯源树,研究论文发展脉络
Chat Paper
正在生成论文摘要