Decomposition-Based Job-Shop Scheduling with Constrained Clustering.
PADL(2022)
摘要
Scheduling is a crucial problem appearing in various domains, such as manufacturing, transportation, or healthcare, where the goal is to schedule given operations on available resources such that the operations are completed as soon as possible. Unfortunately, most scheduling problems cannot be solved efficiently, so that research on suitable approximation methods is of primary importance. This work introduces a novel approximation approach based on problem decomposition with data mining methodologies. We propose a constrained clustering algorithm to group operations into clusters, corresponding to time windows in which the operations must be scheduled. The decomposition process consists of two main phases. First, features are extracted, either from the problem itself or from solutions obtained by heuristic methods, to predict the execution sequence of operations on each resource. The second phase deploys our constrained clustering algorithm to assign each operation into a time window. We then schedule the operations by time windows using Answer Set Programming. Evaluation results show that our proposed approach outperforms other heuristic schedulers in most cases, where incorporating features like Remaining Processing Time , Machine Load , and Earliest Starting Time significantly improves the solution quality.
更多查看译文
关键词
constrained clustering,decomposition-based,job-shop
AI 理解论文
溯源树
样例
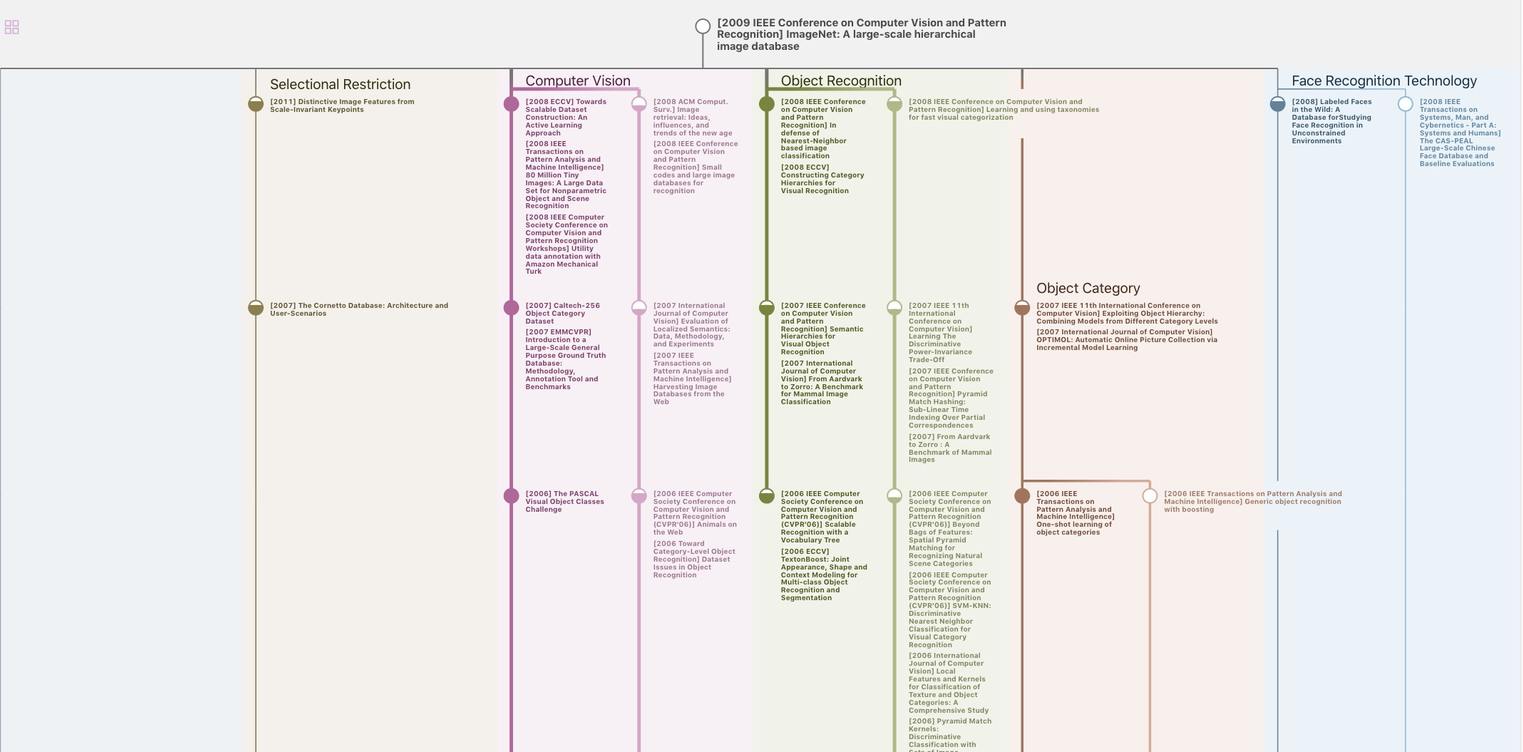
生成溯源树,研究论文发展脉络
Chat Paper
正在生成论文摘要