Object Delineation by Iterative Dynamic Trees.
CIARP(2021)
摘要
While semantic segmentation networks can approximate well the shape of objects (e.g., people, chairs, tables) in images, boundary adherence is still inaccurate. We present an unsupervised image segmentation approach, named Iterative Dynamic Trees (IDT), for improved object delineation. We intend to combine IDT and semantic segmentation networks in future work towards improving object segmentation. For a given number of objects in an image graph, the IDT algorithm (i) estimates one seed per object (and background), (ii) delineates each object as one optimum-path tree, (iii) improves seed estimation and repeats steps (ii)–(iii) for a preset number of iterations or until the seed set convergence is achieved. Then, (iv) the optimum-path forest found with the lowest total cost in the loop (ii)–(iii) is selected as final segmentation. The IDT algorithm is a new method based on the Iterative Spanning Forest (ISF) framework, in which the number of superpixels is drastically reduced to the number of objects. It adds step (iv) and exploits, for the first time, dynamic arc-weight estimation in ISF for unsupervised object segmentation. We show that IDT can outperform its counterparts in two image datasets – a result that motivates its combination with semantic segmentation networks in future work.
更多查看译文
关键词
dynamic,delineation,trees,object
AI 理解论文
溯源树
样例
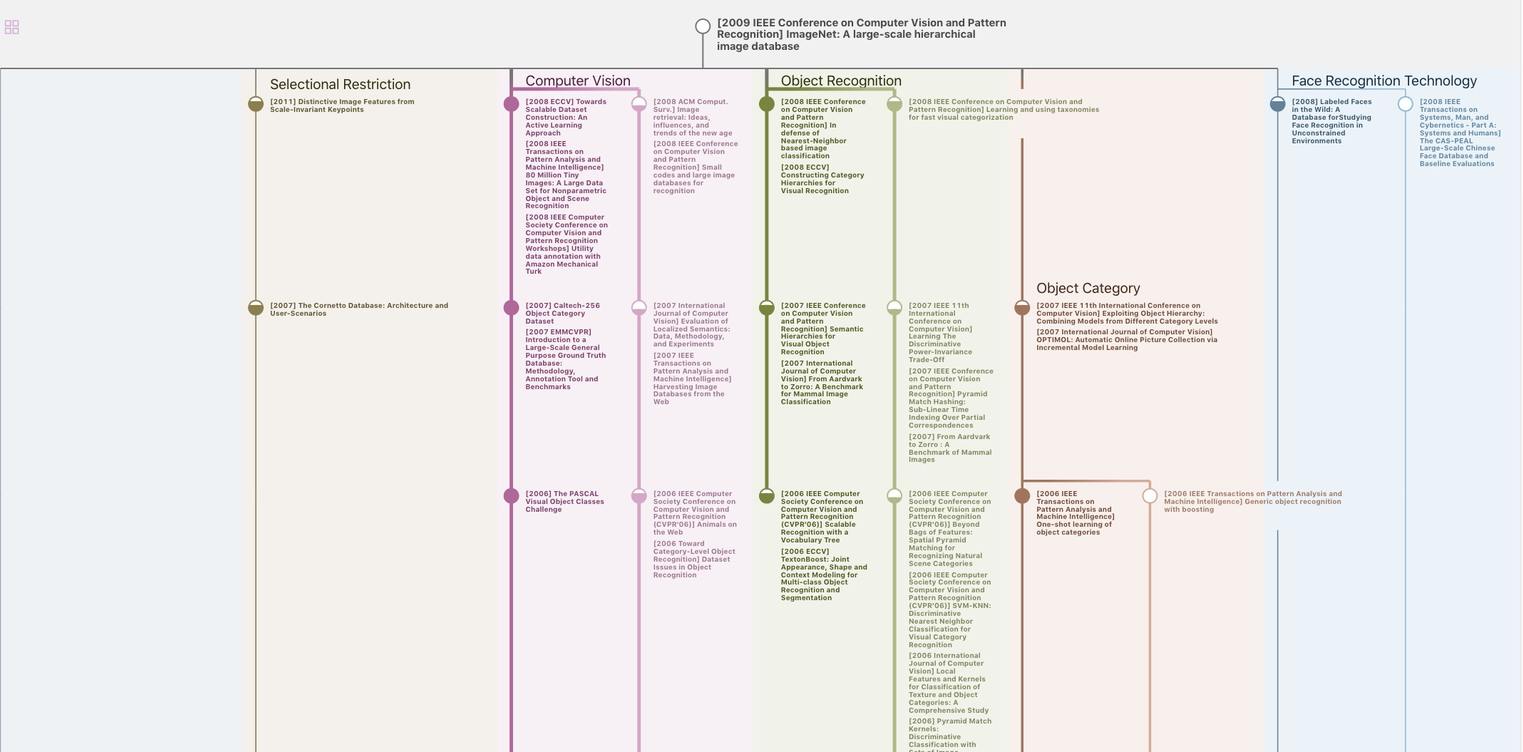
生成溯源树,研究论文发展脉络
Chat Paper
正在生成论文摘要