Cross-Subject Driving Fatigue Detection Model Based on Feature Selection of Critical Functional Connectivity
2021 27TH INTERNATIONAL CONFERENCE ON MECHATRONICS AND MACHINE VISION IN PRACTICE (M2VIP)(2021)
摘要
Driving fatigue in monotonous or demanding driving situations has been a major concern for modern traffic and public safety. Continuous efforts have been made to develop a practical method for driving fatigue detection. Because of its non-invasive and high-temporal resolution, EEG has long been considered as a salient biomarker for driving fatigue detection in recent studies. It is noteworthy that most of the studies up to date focused on single-subject fatigue detection, scattered studies have attempted to develop feasible method for driving fatigue detection across different subjects. In the current work, we used critical functional connectivity (FC) as features to build both single-subject and cross-subject mental fatigue detection models. EEG data were recorded from 19 subjects during a 90-min simulated driving experiment. Multi-band FC matrices were established using Phase Lag Index. Three feature selection methods (including SVM-RFE, Sparse Regression, and PCA) were then used to determine the most contributing FC features for driving fatigue detection. We achieved a superior classification accuracy (99.02%) in single-subject driving fatigue detection using the discriminative connection features from 3 frequency bands (i.e., theta, alpha, and beta) and a comparable accuracy of 97.34% in cross-subject driving fatigue detection. Our results demonstrated that critical connection is an efficient feature for cross-subject driving fatigue detection, and the feature selection results may help build a practical EEG-based driving fatigue detection system.
更多查看译文
关键词
single-subject driving fatigue detection,fatigue detection system,cross-subject driving fatigue detection model,critical functional connectivity,monotonous demanding driving situations,single-subject fatigue detection,cross-subject mental fatigue detection models,90-min simulated driving experiment,feature selection methods
AI 理解论文
溯源树
样例
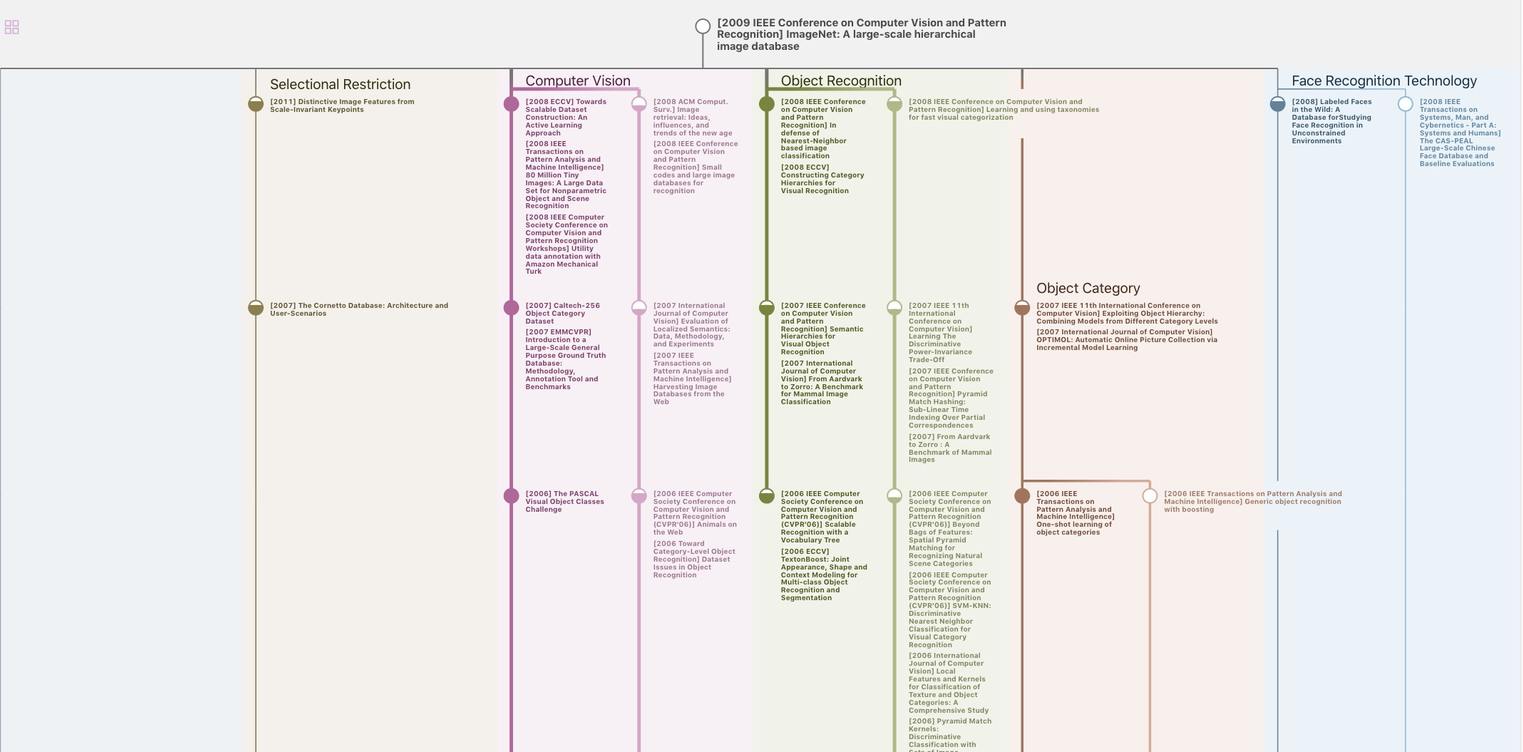
生成溯源树,研究论文发展脉络
Chat Paper
正在生成论文摘要