Detection and Classification of Coronary Artery Plaques in Coronary Computed Tomography Angiography Using 3D CNN.
STACOM@MICCAI(2021)
摘要
Measuring the existence of coronary artery plaques and stenoses is a standard way of evaluating the risk of cardiovascular diseases. Coronary Computed Tomography Angiography (CCTA) is one of the most common assessments of cardiovascular disease. Manually detecting stenoses over the complete coronary artery tree of each patient takes an expert about 10 min. Previous works about automated detection of plaques required carefully controlling parameters of region-based segmentation algorithms or hand-craft features extraction for machine learning algorithms. Current works presented the feasibility of deep learning algorithms for plaque and stenosis detection. This study aims to develop an efficient workflow for detecting plaques and classification using a 3D convolutional neural network (CNN). Based on a fully convolutional structure, our model has better stability than recurrent layers at the training stage. Furthermore, our method is efficient since the model takes only cross-sectional planes (CSP) images as the input, rather than including other views containing replicate information. For each patient, it takes less than 25 s to perform the whole workflow. The first step of our workflow is to extract CSP images on each control point of a given set of coronary artery centerlines. We then stack several contiguous CSP images together to form a 3D volume, which is the input of the CNN model. The model will predict the type of plaque (soft, mixed, or calcified) at each control point. With this information, we can not only detect the precise location of plaques but also summarize the existence of plaque in each artery segment. In addition, we consider the lumen radius estimation as the next step to approach stenosis detection.
更多查看译文
关键词
Coronary plaque, Coronary Computed Tomography Angiography (CCTA), Convolutional neural network (CNN)
AI 理解论文
溯源树
样例
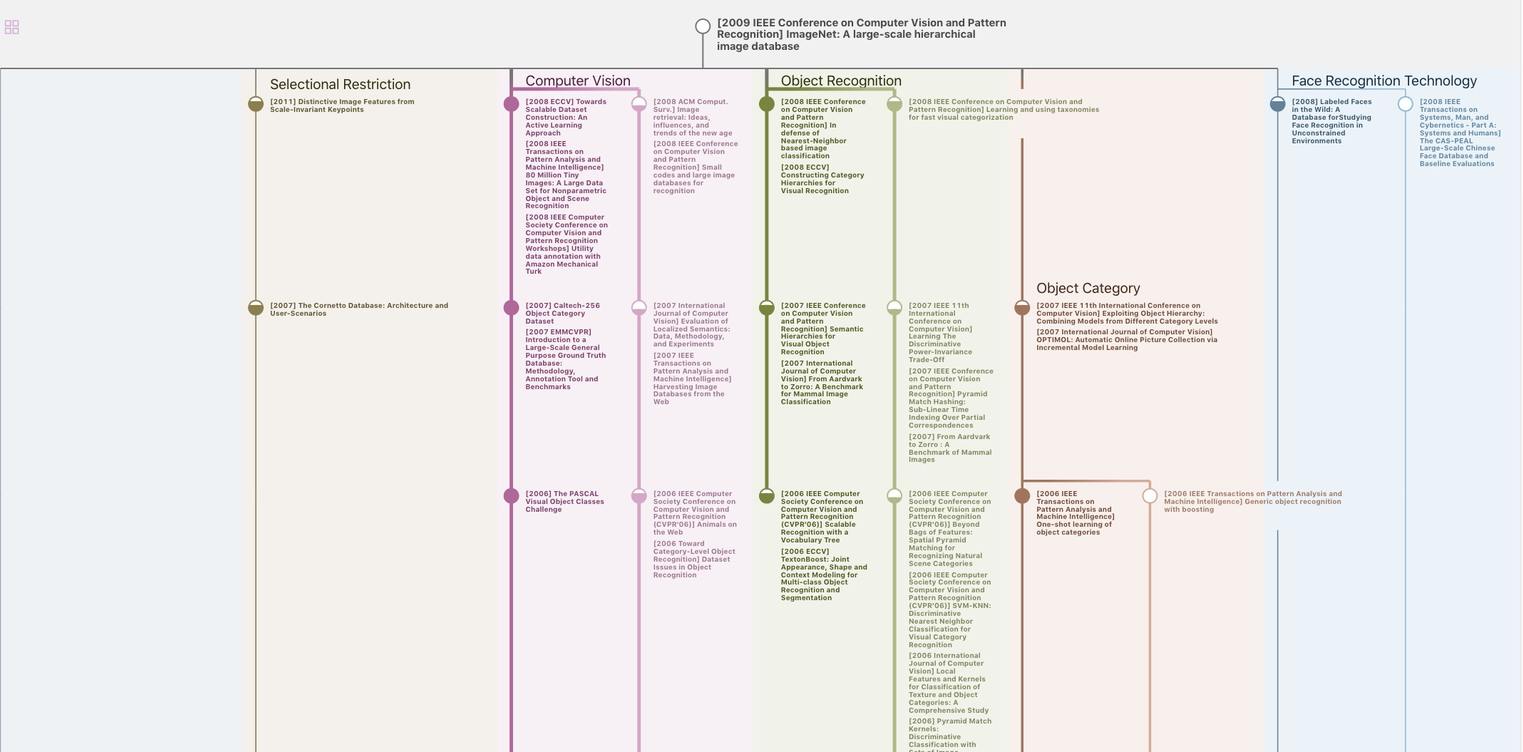
生成溯源树,研究论文发展脉络
Chat Paper
正在生成论文摘要