Channel Self-Attention Deep Learning Framework for Multi-Cardiac Abnormality Diagnosis from Varied-Lead ECG Signals.
CinC(2021)
摘要
Electrocardiogram (ECG) signals are widely used to diagnose heart health. Experts can detect multiple cardiac abnormalities using the ECG signal. In a clinical setting, 12-lead ECG is mainly used. But using fewer leads can make the ECG more pervasive as it can be integrated with wearable devices. At the same time, we need to build systems that can diagnose cardiac abnormalities automatically. This work develops a channel self-attention-based deep neural network to diagnose cardiac abnormality using a different number of ECG lead combinations. Our approach takes care of the temporal and spatial interdependence of multi-lead ECG signals. Our team participates under the name "cardiochallenger" in the "PhysioNet/Computing in Cardiology Challenge 2021". Our method achieves the challenge metric score of 0.55, 0.51, 0.53, 0.51, and 0.53 (ranked 2nd; 5th; 4th; 5th and 4th) for the 12-lead, 6-lead, 4-lead, 3-lead, and 2-lead cases, respectively, on the test data set.
更多查看译文
关键词
multicardiac abnormality diagnosis,electrocardiogram signals,heart health,cardiac abnormalities,ECG signal,wearable devices,cardiac abnormality,multilead ECG signals,self-attention-based deep neural network
AI 理解论文
溯源树
样例
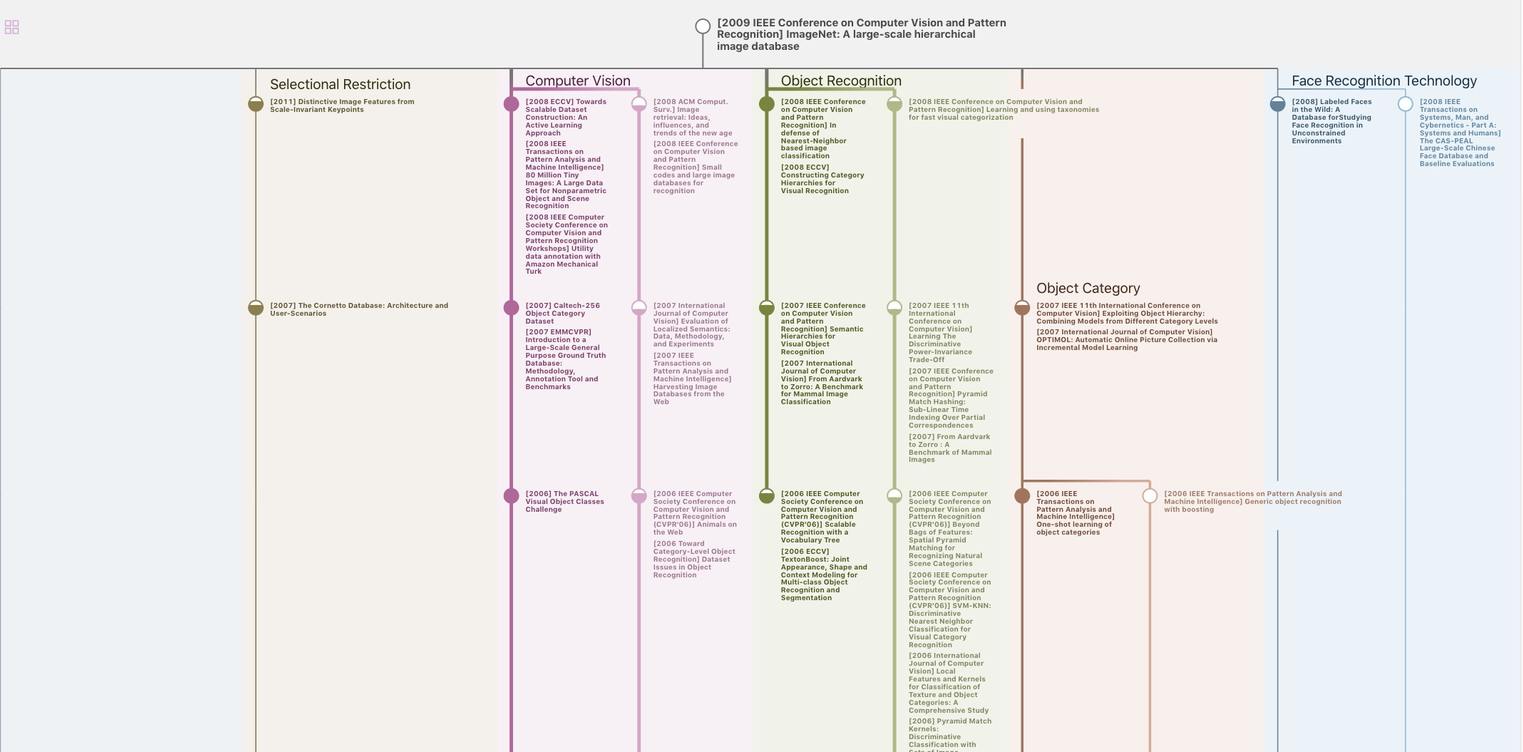
生成溯源树,研究论文发展脉络
Chat Paper
正在生成论文摘要