Attention-enhanced Graph Cross-convolution for Protein-Ligand Binding Affinity Prediction
BIBM(2021)
摘要
The binding affinity between drugs and proteins is a substantial part of the drug discovery process. Graph neural networks (GNNs) have shown great promise in graph-related structure by learning the representations of graphs, which are suitable for tasks such as binding affinity prediction. However, most of the existing GNN architectures only pay attention to the information flow on a single graph, while the interaction between two graphs is unconcerned. In this paper, we propose an attention-enhanced graph cross-convolution network (GCAT) to explore binding affinity on pure 3D atomistic geometry. It consists of two components: cross-convolution and self-attention pooling. Specifically, cross-convolution performs an aggregate-update mechanism to simulate the interaction between the protein and the drug, then self-attention pooling is adopted to capture global interactions and get graph-level representations. Extensive experiments conducted on the PDB-Bind dataset demonstrate the effectiveness of our GCAT.
更多查看译文
关键词
protein-ligand affinity prediction,graph neural network,cross convolution,self-attention,interaction modeling
AI 理解论文
溯源树
样例
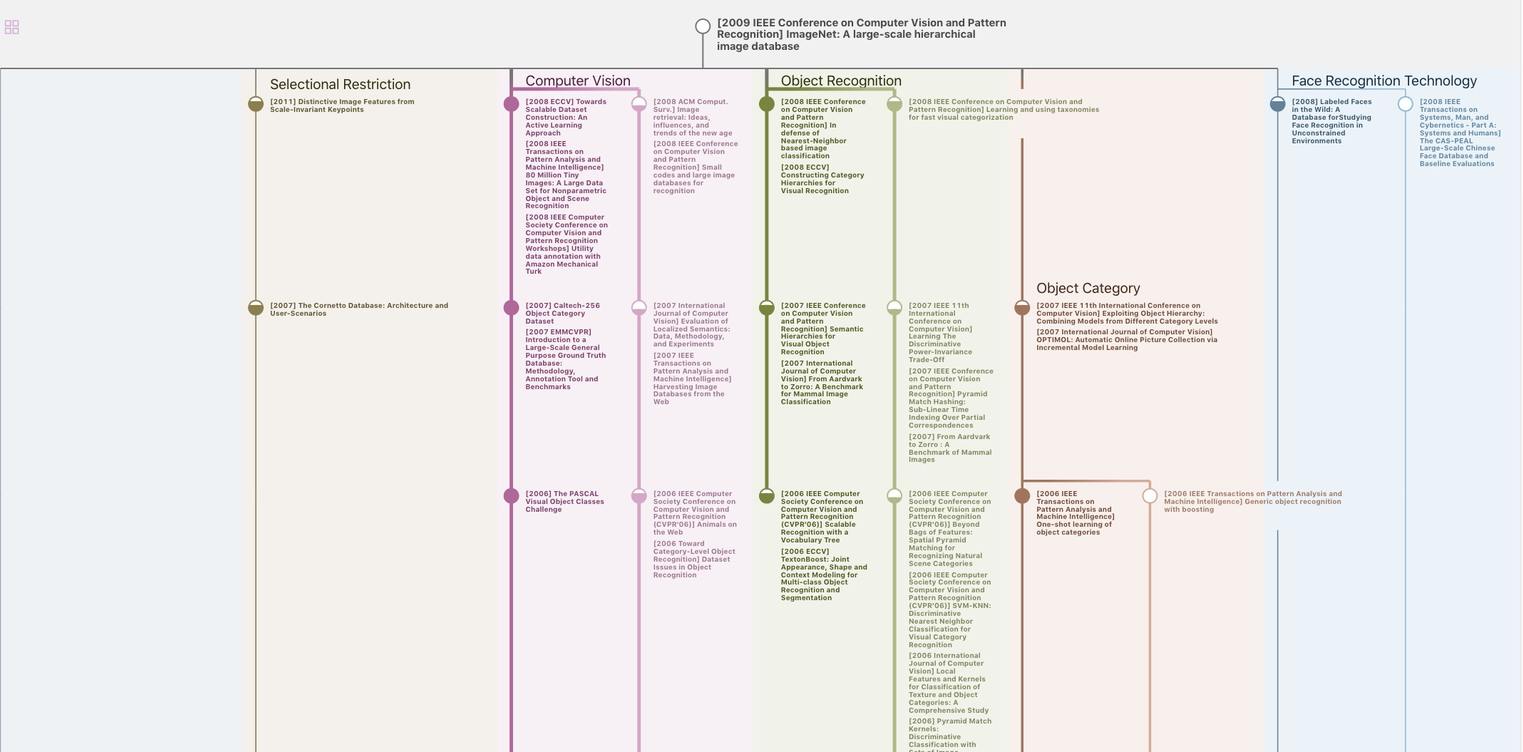
生成溯源树,研究论文发展脉络
Chat Paper
正在生成论文摘要