IEEG-TCN: A Concise and Robust Temporal Convolutional Network for Intracranial Electroencephalogram Signal Identification
BIBM(2021)
摘要
Intracranial electroencephalogram (IEEG) is an invasive procedure widely used for preoperative assessment of drug-resistant epilepsy (DRE). In recent years, methods based on machine learning achieved high accuracy in automatic EEG recognition. However, these models have also grown in complexity, requiring a large amount of time for various feature extraction or signal transformation, which makes it difficult to efficiently process the IEEG recordings that span days to weeks. In this study, we propose IEEG-TCN, a concise temporal convolutional network that performs nicely on different IEEG classification tasks while costing less time. According to experimental results on the Bern-Barcelona EEG dataset, our method reaches an impressive accuracy of 93.76% in detecting focal signals. The latency for processing each IEEG segment is reduced by 19 seconds. We also verify the robustness of IEEGTCN based on the Multicenter IEEG dataset that contains four categories of IEEG segments (physiological activity, pathological activity, artifacts, and noise). The results show that the model can be successfully generalized to multi-class problems.
更多查看译文
关键词
drug-resistant epilepsy (DRE),intracranial EEG (IEEG) signal identification,temporal convolutional network
AI 理解论文
溯源树
样例
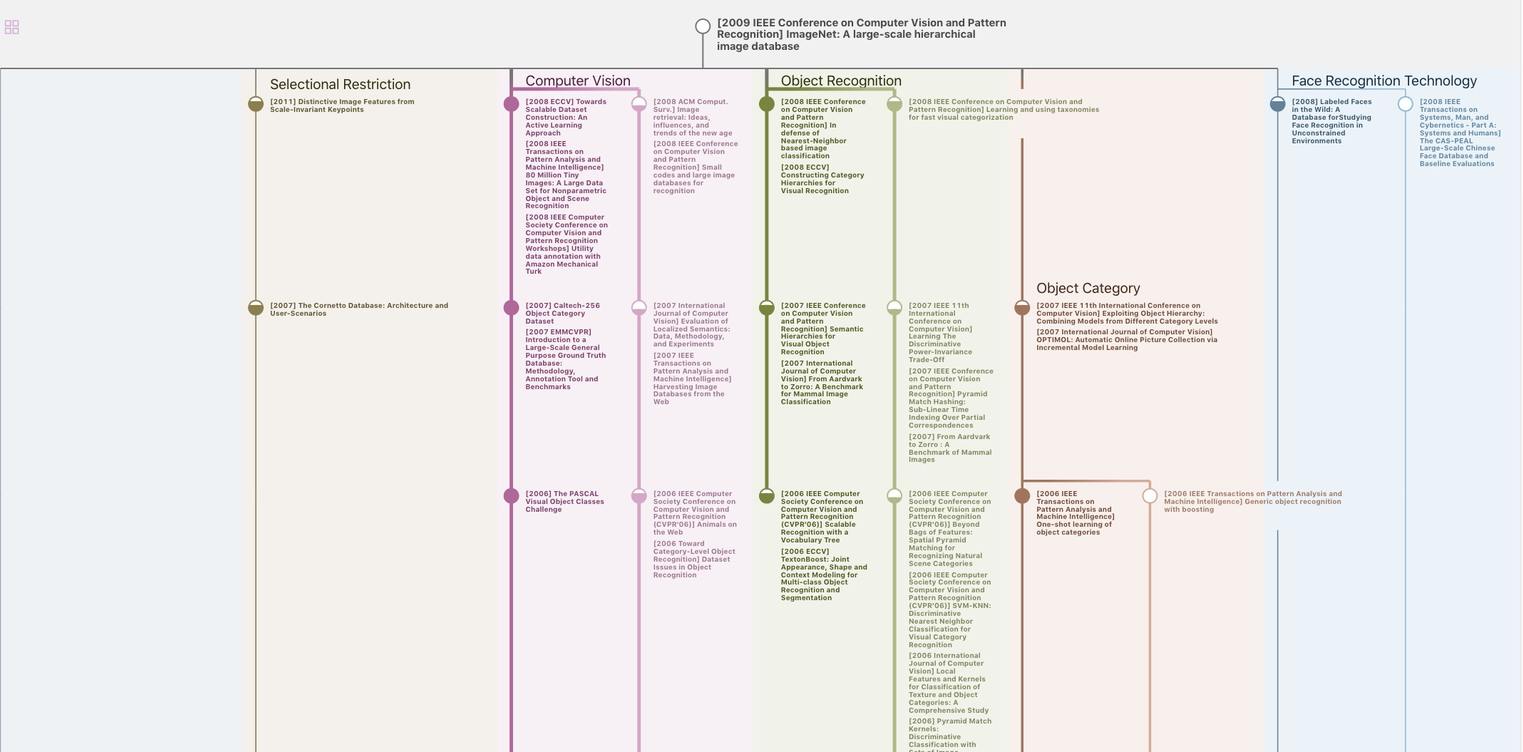
生成溯源树,研究论文发展脉络
Chat Paper
正在生成论文摘要