CellDet - Dual-Task Cell Detection Network for IHC-Stained Image Analysis.
BIBM(2021)
摘要
Cell detection on immunohistochemistry stained (IHC-stained) images plays an essential role in computer assisted prediction of tumor progression and treatment response. Currently available cell detection datasets provide either point level or bounding box level annotations for deep object detection network training. And these widely used networks usually employ standard pyramid structured multi-scale feature fusion. However, we find that these methods have obvious limitation when facing large amounts of cells in similar scale with severe overlapping. To address this problem, we propose a novel CellDet network with (1) Scale Consistency Feature Fusion Module (SCFFM) and (2) Dual Task Detection Module to simultaneously exploit the complementary information from both point and bounding box annotations. In order to verify the effectiveness our proposed method, We make efforts to relabel the public SHIDC-B-Ki-67 dataset with bounding box annotations. Extensive experimental results show that the proposed CellDet outperforms other state-of-the-art cell detection methods with a remarkable margin. We will release our source code and dataset in https://github.com/JiweiMaster/celldet.
更多查看译文
关键词
Immunohistochemistry Stained Histopathological Image Analysis,Cell Detection,Dual-Task Learning,Scale Consistency Feature Fusion
AI 理解论文
溯源树
样例
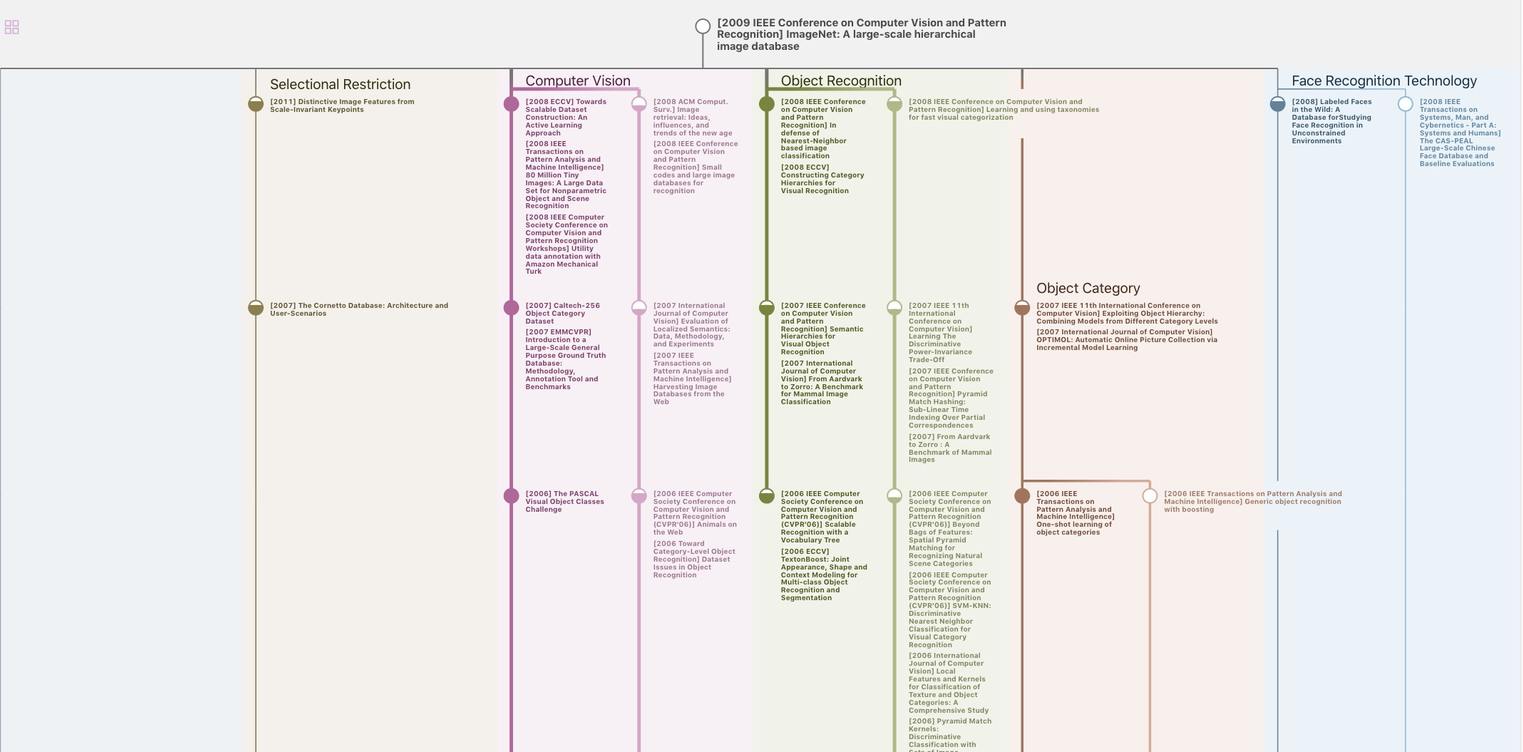
生成溯源树,研究论文发展脉络
Chat Paper
正在生成论文摘要