Text Fingerprinting and Topic Mining in the Prescription Opioid Use Literature
BIBM(2021)
摘要
Prescription opioids are powerful pain-reducing medications. Thousands of articles that focus on prescription opioid use (POU) and its associated medical disorders have been published. However, it is time-consuming and labor-intensive to extract and understand the information of all POU-related published articles. In this study, we applied the well-adapted topic modeling method, Latent Dirichlet Allocation (LDA), to perform text mining on POU-related literature. We have collected six large academic abstract datasets by searching PubMed using the Medical Subject Headings (MeSH): prescription opioid, codeine, morphine, hydrocodone, oxycodone, and methadone. We then applied topic modeling to identify topics and analyze topic similarities/differences in these six datasets. Word clouds and histograms were used to depict the distribution of vocabularies over each topic in which the most prevalent words conveyed a topic’s meaning. TreeMap and trend analysis were performed to fingerprint abstracts and explore the prevalent topic dynamics in the POU-related literature. Results showed the ability of topic modeling as a computational tool to segregate a vast quantity of articles into different themes that provide a systematic literature overview. The LDA topics recaptured the search keywords in PubMed and revealed further relevant themes by comparison analysis between different datasets.
更多查看译文
关键词
text mining,topic modeling,Latent Dirichlet Allocation,prescription opioid,codeine,morphine,hydrocodone,oxycodone,methadone
AI 理解论文
溯源树
样例
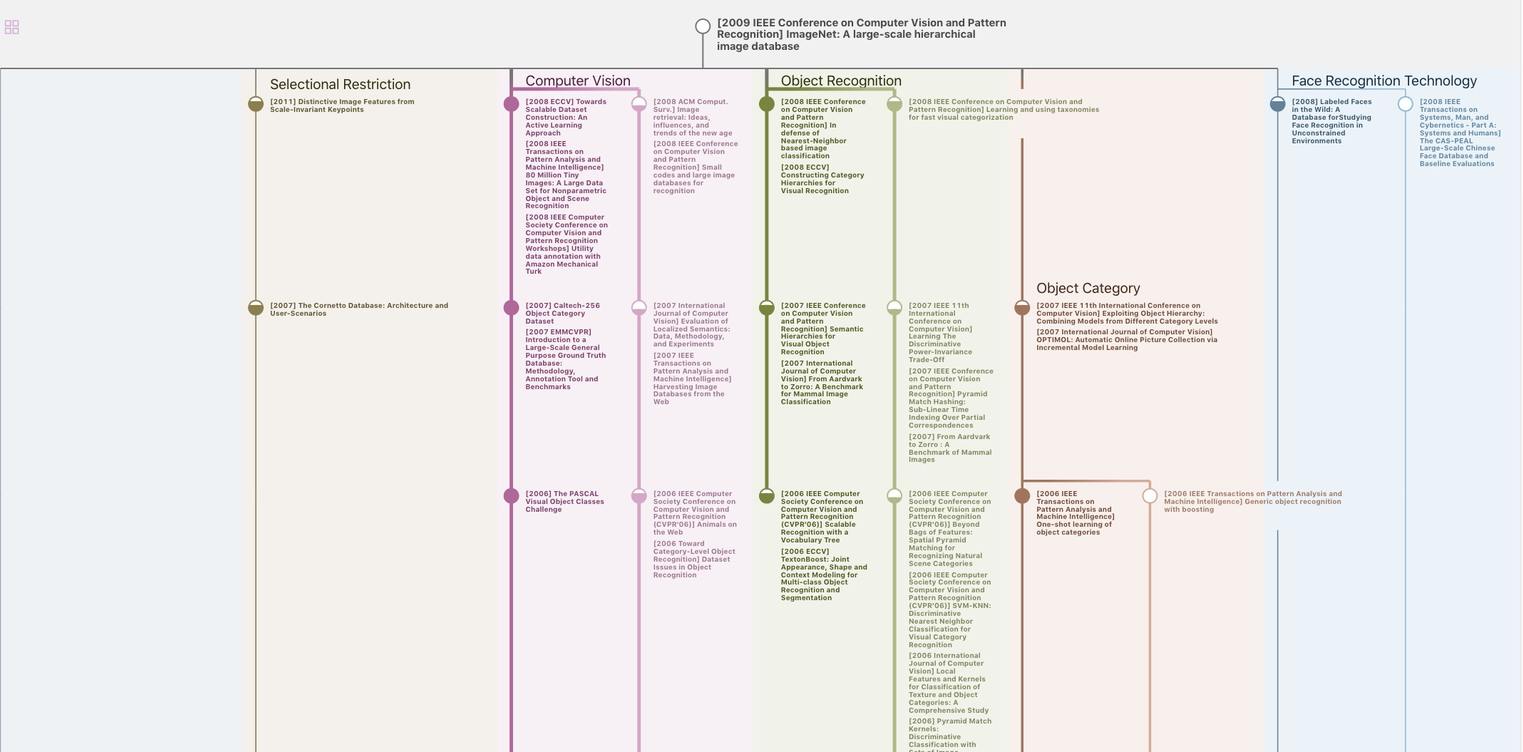
生成溯源树,研究论文发展脉络
Chat Paper
正在生成论文摘要