Fusion Branch Network with Class Learning Difficulty Loss Function for Recongnizition of Haematoma Expansion Signs in Intracerebral Haemorrhage
2021 IEEE International Conference on Bioinformatics and Biomedicine (BIBM)(2021)
摘要
The automatic identification of hematoma expansion signs is very important to the diagnosis and treatment of intracerebral hemorrhage. However, the brain computed tomography samples is uneven distribution, low variation in imaging performance between signs, developing such a solution is challenging.In this article, a novel deep learning network is presented to recognize hematoma expansion signs automatically, which provides a new method to assist in the diagnosis of hematoma growth. First, we propose the fusion branching network (FBN) for extracting and fusing the high-dimensional features of each category dynamically. Second, this paper designs a Class Learning Difficulty (CLD) loss function to dynamically adjust the class weights based on the FBN learning situation to help the model avoid learning bias and improve the performance in signs recognition. In addition, a visualization component is provided to improve the transparency of the model. In the experiment, we retrospectively collected DICOM images from the First Affiliated Hospital of C hongqing Medical University, in order to form a recognition process consistent with clinical work, the data is divided into three categories: Blend Sign (BS), Black Hole Sign (BHS), ICH without BS and BHS (ICHWBB) for sign recognition. The experimental results demonstrate that our method performed the best in terms of comprehensive evaluation indexes compared with other methods, and the recognition sensitivity reached 0.9404, 0.8056, 0.7586 for ICHWBB, BHS, and BS, respectively, which was significantly improved compared with other methods in the same environment.
更多查看译文
关键词
FBN,CLD,hematoma expansion signs in intracerebral hemorrhage,automatic identification
AI 理解论文
溯源树
样例
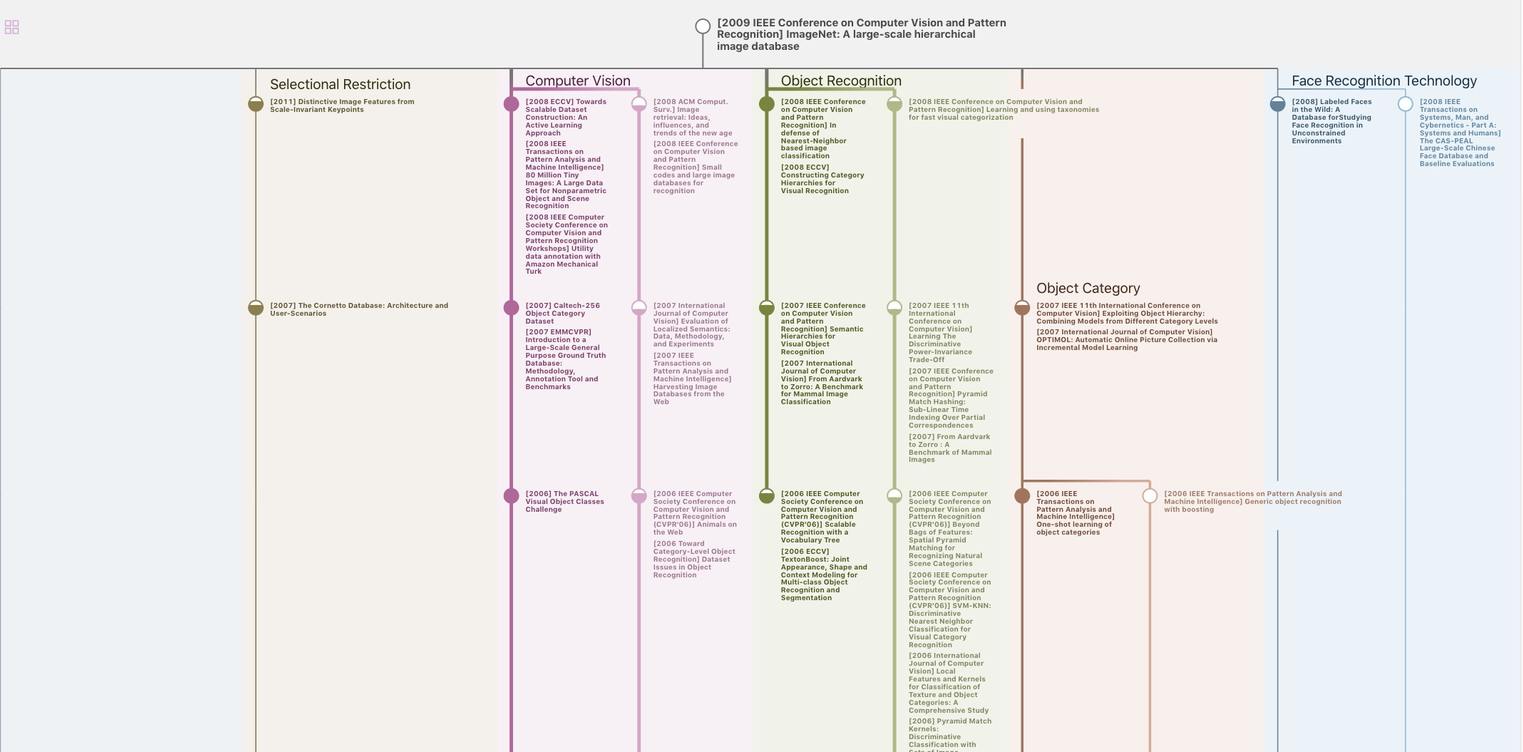
生成溯源树,研究论文发展脉络
Chat Paper
正在生成论文摘要