TransPicker - a Transformer-based Framework for Particle Picking in cryoEM Micrographs.
BIBM(2021)
摘要
Single-particle cryo-electron microscopy (cryoEM) methods are powerful for solving high-resolution structures of biological macromolecules. Locating numerous particles from micrographs is essential for three-dimensional reconstruction but challenging due to the extremely low signal-to-noise ratio and various particle shapes in micrographs. In this study, we devise the TransPicker, a two-dimensional particle picking framework based on a novel end-to-end transformer-based detective method named crDETR (cryoEM DEtection TRansformer). crDETR applies an improved deformable Transformer to perform inference in parallel on particle relocation and global context, without hand-crafted components like anchors, non-maximum suppression procedure, or sliding windows. Also, it uses a combined loss function to guarantee fast convergence. It uses divide-and-conquer to overcome the limitations of the object query number. Moreover, we develop a series of optimizations, including denoising, enhancing, bad particle filtering, adding masks on carbon areas and ice contaminants to decrease the false-positive ratio and improve the accuracy of particle picking. Experimental results on various datasets demonstrate that TransPicker can select particles with more accuracy, especially in high noise compared with other methods. To our knowledge, TransPicker is the first application of the transformer technique in cryoEM particle picking.
更多查看译文
关键词
Transformer,particle-picking,cryoEM,object detection,deep learning
AI 理解论文
溯源树
样例
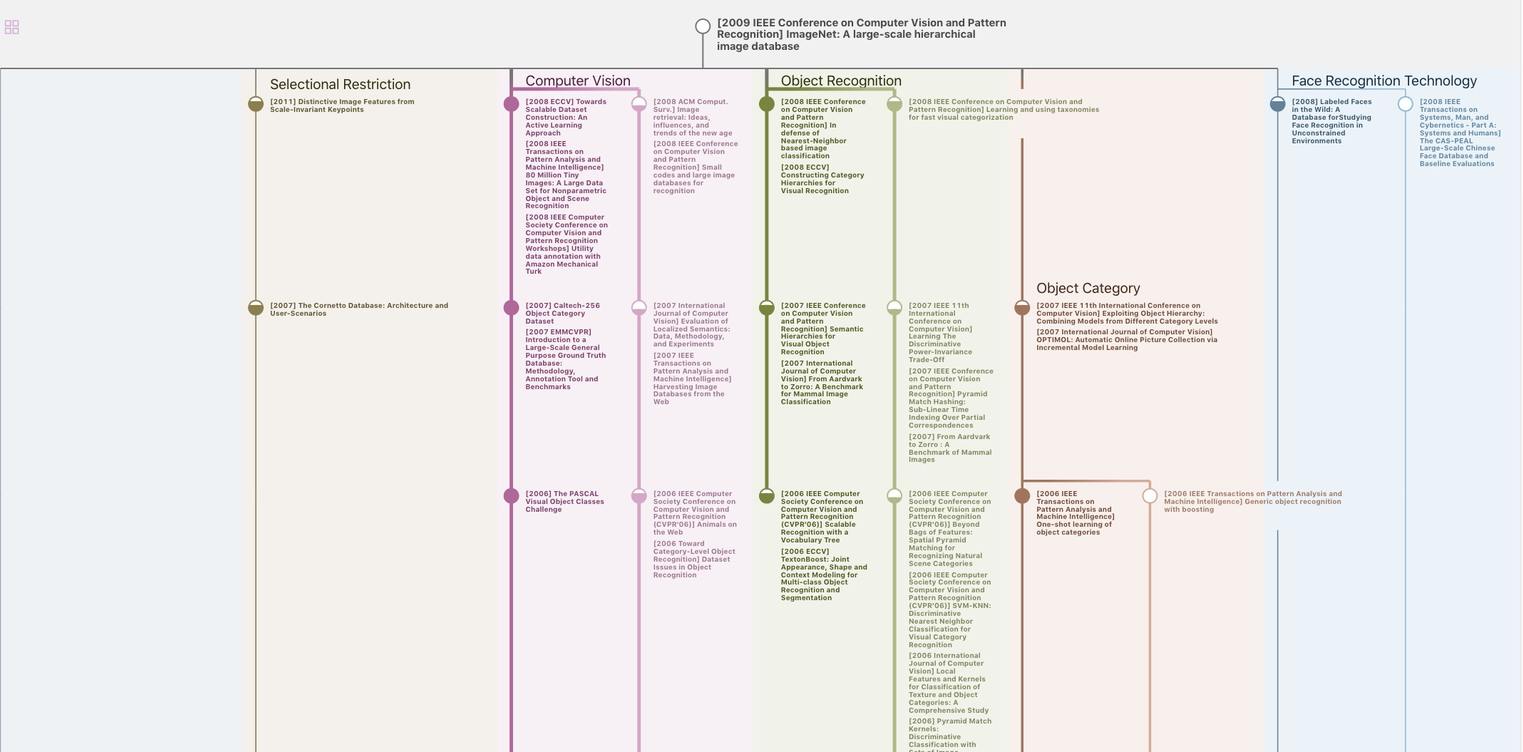
生成溯源树,研究论文发展脉络
Chat Paper
正在生成论文摘要