MonLAD: Money Laundering Agents Detection in Transaction Streams
WSDM(2022)
摘要
ABSTRACTGiven a stream of money transactions between accounts in a bank, how can we accurately detect money laundering agent accounts and suspected behaviors in real-time? Money laundering agents try to hide the origin of illegally obtained money by dispersive multiple small transactions and evade detection by smart strategies. Therefore, it is challenging to accurately catch such fraudsters in an unsupervised manner. Existing approaches do not consider the characteristics of those agent accounts and are not suitable to the streaming settings. Therefore, we propose MonLAD and MonLAD-W to detect money laundering agent accounts in a transaction stream by keeping track of their residuals and other features; we devise AnoScore algorithm to find anomalies based on the robust measure of statistical deviation. Experimental results show that MonLAD outperforms the state-of-the-art baselines on real-world data and finds various suspicious behavior patterns of money laundering. Additionally, several detected suspected accounts have been manually-verified as agents in real money laundering scenario.
更多查看译文
关键词
Anomaly detection, Money laundering, Stream algorithm
AI 理解论文
溯源树
样例
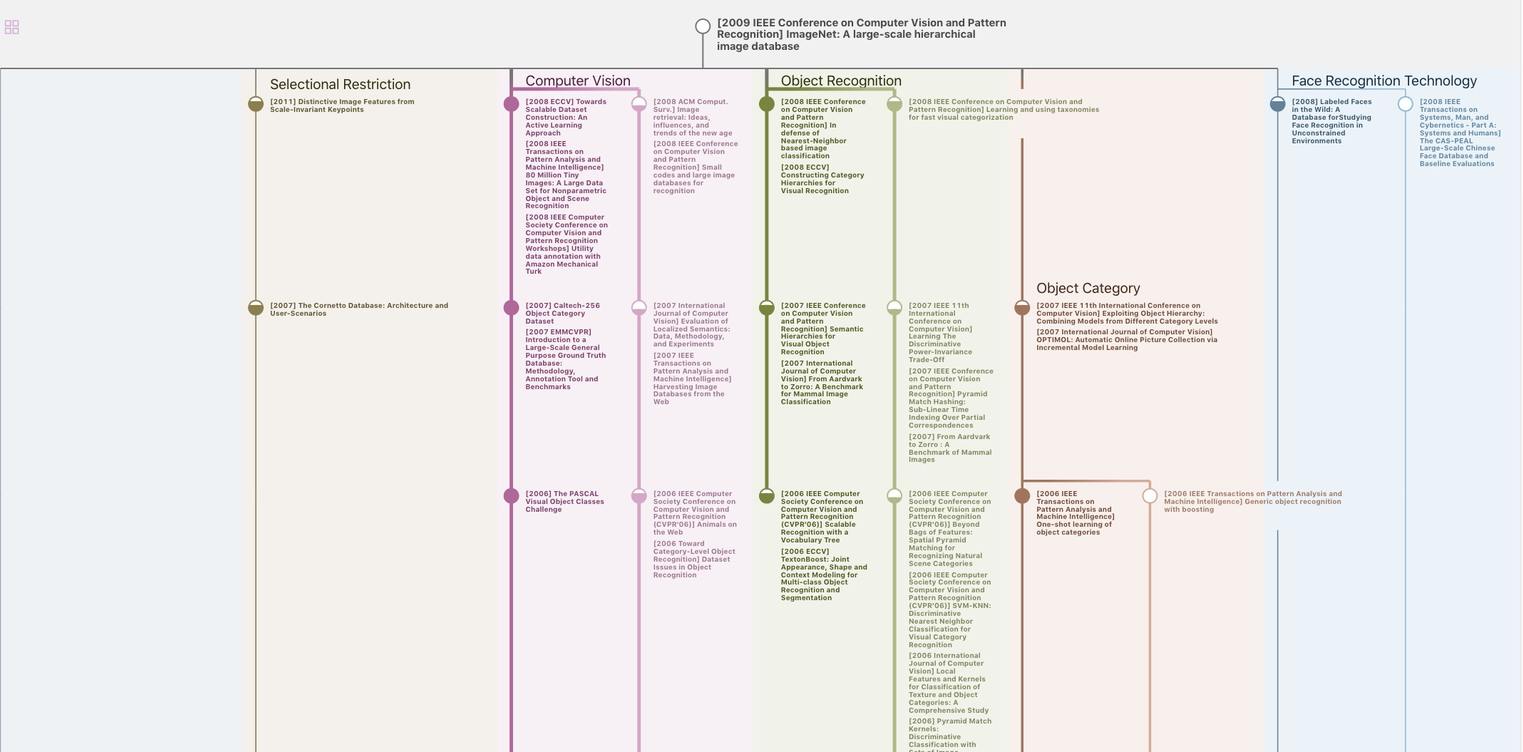
生成溯源树,研究论文发展脉络
Chat Paper
正在生成论文摘要