MSNet: A Deep Multi-scale Submanifold Network for Visual Classification
CoRR(2022)
摘要
The Symmetric Positive Definite (SPD) matrix has received wide attention as a tool for visual data representation in computer vision. Although there are many different attempts to develop effective deep architectures for data processing on the Riemannian manifold of SPD matrices, a very few solutions explicitly mine the local geometrical information in deep SPD feature representations. While CNNs have demonstrated the potential of hierarchical local pattern extraction even for SPD represented data, we argue that it is of utmost importance to ensure the preservation of local geometric information in the SPD networks. Accordingly, in this work we propose an SPD network designed with this objective in mind. In particular, we propose an architecture, referred to as MSNet, which fuses geometrical multi-scale information. We first analyse the convolution operator commonly used for mapping the local information in Euclidean deep networks from the perspective of a higher level of abstraction afforded by the Category Theory. Based on this analysis, we postulate a submanifold selection principle to guide the design of our MSNet. In particular, we use it to design a submanifold fusion block to take advantage of the rich local geometry encoded in the network layers. The experiments involving multiple visual tasks show that our algorithm outperforms most Riemannian SOTA competitors.
更多查看译文
AI 理解论文
溯源树
样例
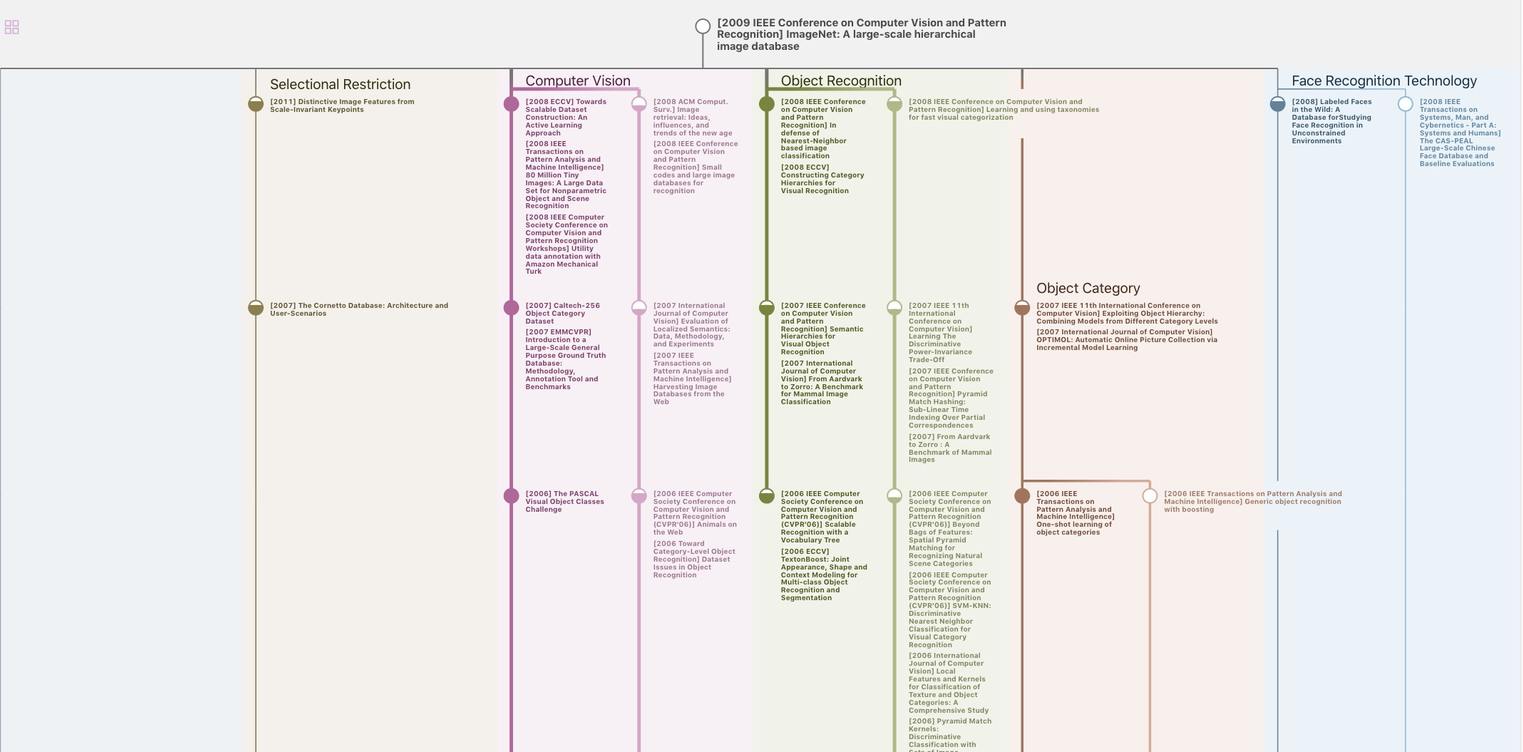
生成溯源树,研究论文发展脉络
Chat Paper
正在生成论文摘要