Neural Information Squeezer for Causal Emergence
Entropy(2023)
摘要
Conventional studies of causal emergence have revealed that stronger causality can be obtained on the macro-level than the micro-level of the same Markovian dynamical systems if an appropriate coarse-graining strategy has been conducted on the micro-states. However, identifying this emergent causality from data is still a difficult problem that has not been solved because the appropriate coarse-graining strategy can not be found easily. This paper proposes a general machine learning framework called Neural Information Squeezer to automatically extract the effective coarse-graining strategy and the macro-level dynamics, as well as identify causal emergence directly from time series data. By using invertible neural network, we can decompose any coarse-graining strategy into two separate procedures: information conversion and information discarding. In this way, we can not only exactly control the width of the information channel, but also can derive some important properties analytically. We also show how our framework can extract the coarse-graining functions and the dynamics on different levels, as well as identify causal emergence from the data on several exampled systems.
更多查看译文
关键词
causal emergence,coarse-graining,invertible neural network
AI 理解论文
溯源树
样例
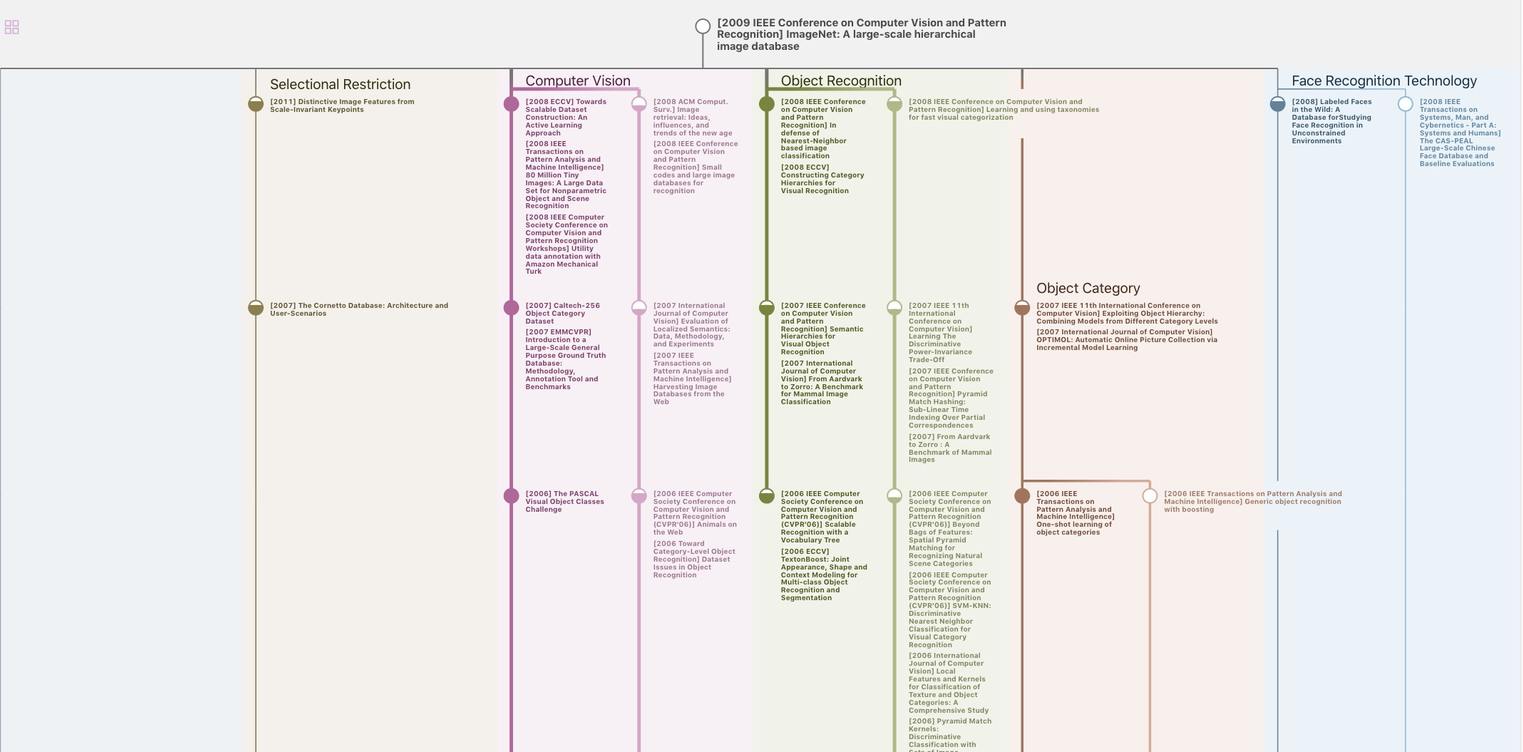
生成溯源树,研究论文发展脉络
Chat Paper
正在生成论文摘要