Similarity bias from consensus perturbational signatures from the L1000 Connectivity Map
biorxiv(2024)
摘要
The Next Generation L1000 Connectivity Map (L1000) is a massive, high-throughput dataset measuring transcriptional changes - or signatures - in cancer cell lines due to chemical and genetic perturbation. Zhu et al recently presented Deep Learning-Based Efficacy Prediction System (DLEPS), a method that models averages of many signatures (CTPs or meta-signatures) of the same compound across conditions. However, our analysis shows that averaging increasing numbers of signatures from L1000 results in a substantial positive increase in signature similarities even among entirely unrelated perturbations, drastically shifting the baseline threshold for similarity. Consequently, DLEPS overstates how informative the model is about each compound, highlighting the need to account for this similarity bias in any L1000 Connectivity Map analysis.
### Competing Interest Statement
BHK is a paid consultant and shareholder of Code Ocean Inc. and is part of the Scientific Advisory Board of the Break through Cancer (BTC) Foundation.
更多查看译文
关键词
connectivity map,similarity bias,signatures
AI 理解论文
溯源树
样例
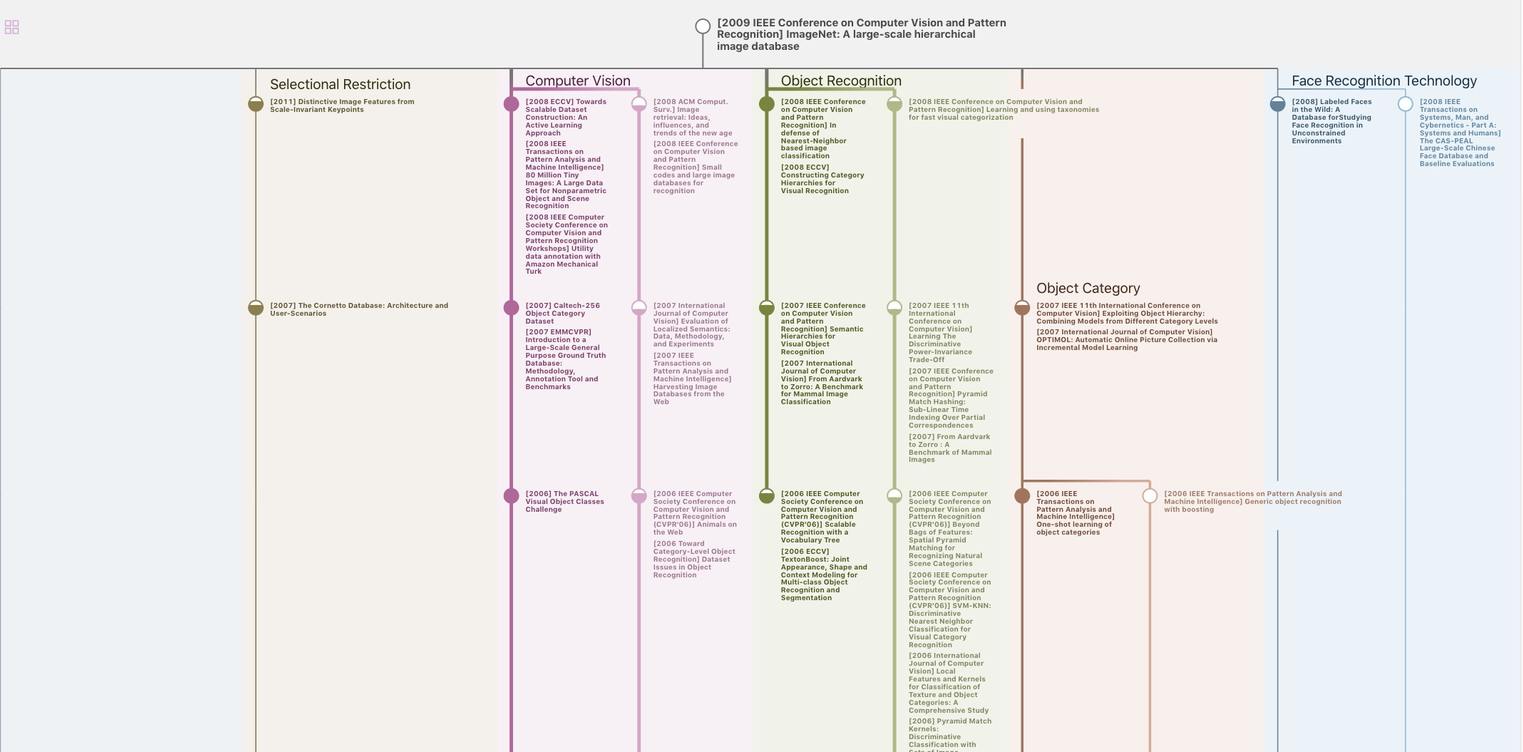
生成溯源树,研究论文发展脉络
Chat Paper
正在生成论文摘要