MAGAN: Unsupervised Low-Light Image Enhancement Guided by Mixed-Attention
Big data mining and analytics(2022)
摘要
Most learning-based low-light image enhancement methods typically suffer from two problems. First, they require a large amount of paired data for training, which are difficult to acquire in most cases. Second, in the process of enhancement, image noise is difficult to be removed and may even be amplified. In other words, performing denoising and illumination enhancement at the same time is difficult. As an alternative to supervised learning strategies that use a large amount of paired data, as presented in previous work, this paper presents an mixed-attention guided generative adversarial network called MAGAN for low-light image enhancement in a fully unsupervised fashion. We introduce a mixed-attention module layer, which can model the relationship between each pixel and feature of the image. In this way, our network can enhance a low-light image and remove its noise simultaneously. In addition, we conduct extensive experiments on paired and no-reference datasets to show the superiority of our method in enhancing low-light images.
更多查看译文
关键词
low-light image enhancement,unsupervised learning,Generative Adversarial Network (GAN),mixed-attention
AI 理解论文
溯源树
样例
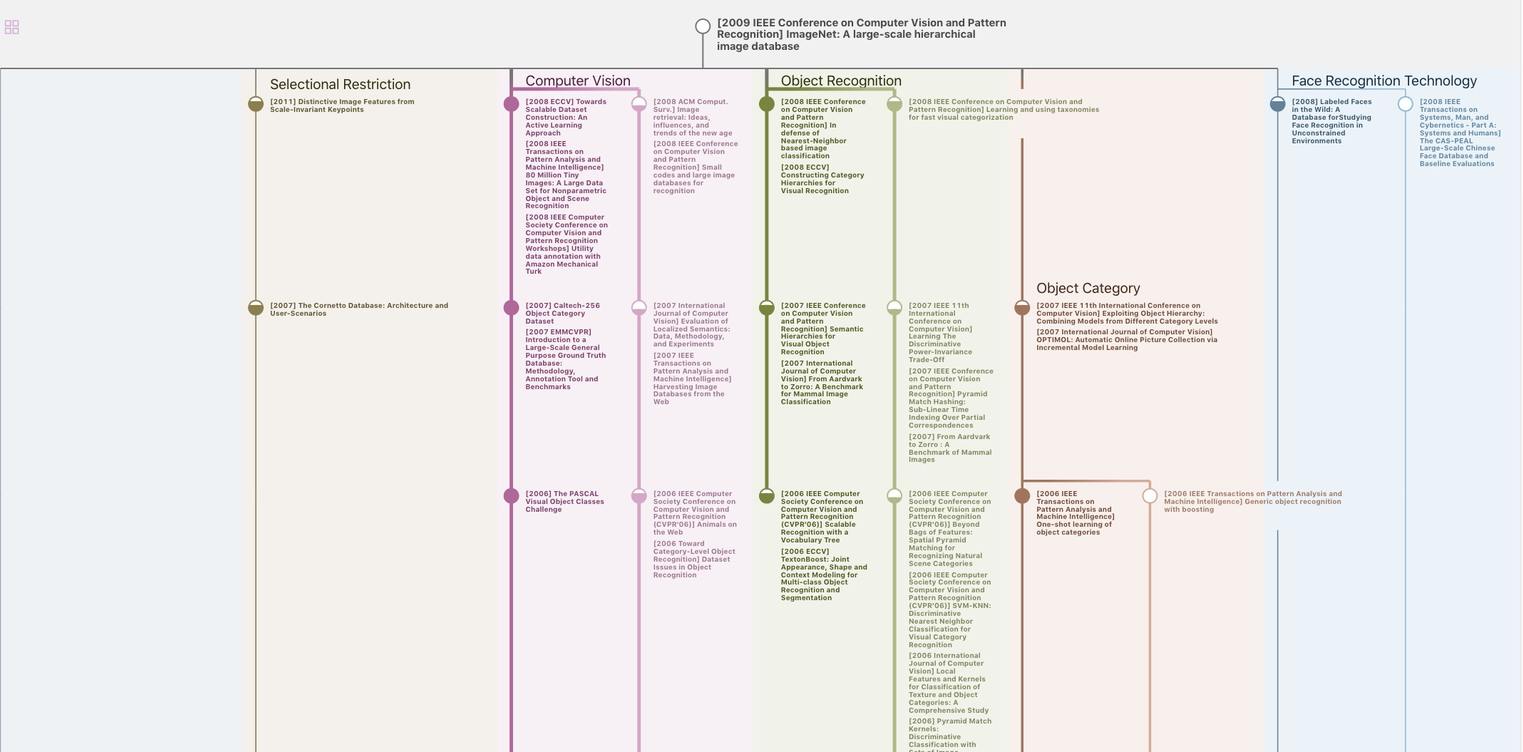
生成溯源树,研究论文发展脉络
Chat Paper
正在生成论文摘要