Second‐order Asymmetric Convolution Network for Breast Cancer Histopathology Image Classification
Journal of biophotonics(2022)
摘要
Recently, convolutional neural networks (CNNs) have been widely utilized for breast cancer histopathology image classification. Besides, research works have also convinced that deep high-order statistic models obviously outperform corresponding first-order counterparts in vision tasks. Inspired by this, we attempt to explore global deep high-order statistics to distinguish breast cancer histopathology images. To further boost the classification performance, we also integrate asymmetric convolution into the second-order network and propose a novel second-order asymmetric convolution network (SoACNet). SoACNet adopts a series of asymmetric convolution blocks to replace each stand square-kernel convolutional layer of the backbone architecture, followed by a global covariance pooling to compute second-order statistics of deep features, leading to a more robust representation of histopathology images. Extensive experiments on the public BreakHis dataset demonstrate the effectiveness of SoACNet for breast cancer histopathology image classification, which achieves competitive performance with the state-of-the-arts.
更多查看译文
关键词
asymmetric convolution,breast cancer histopathology image classification,convolutional neural network,covariance pooling,second-order statistics
AI 理解论文
溯源树
样例
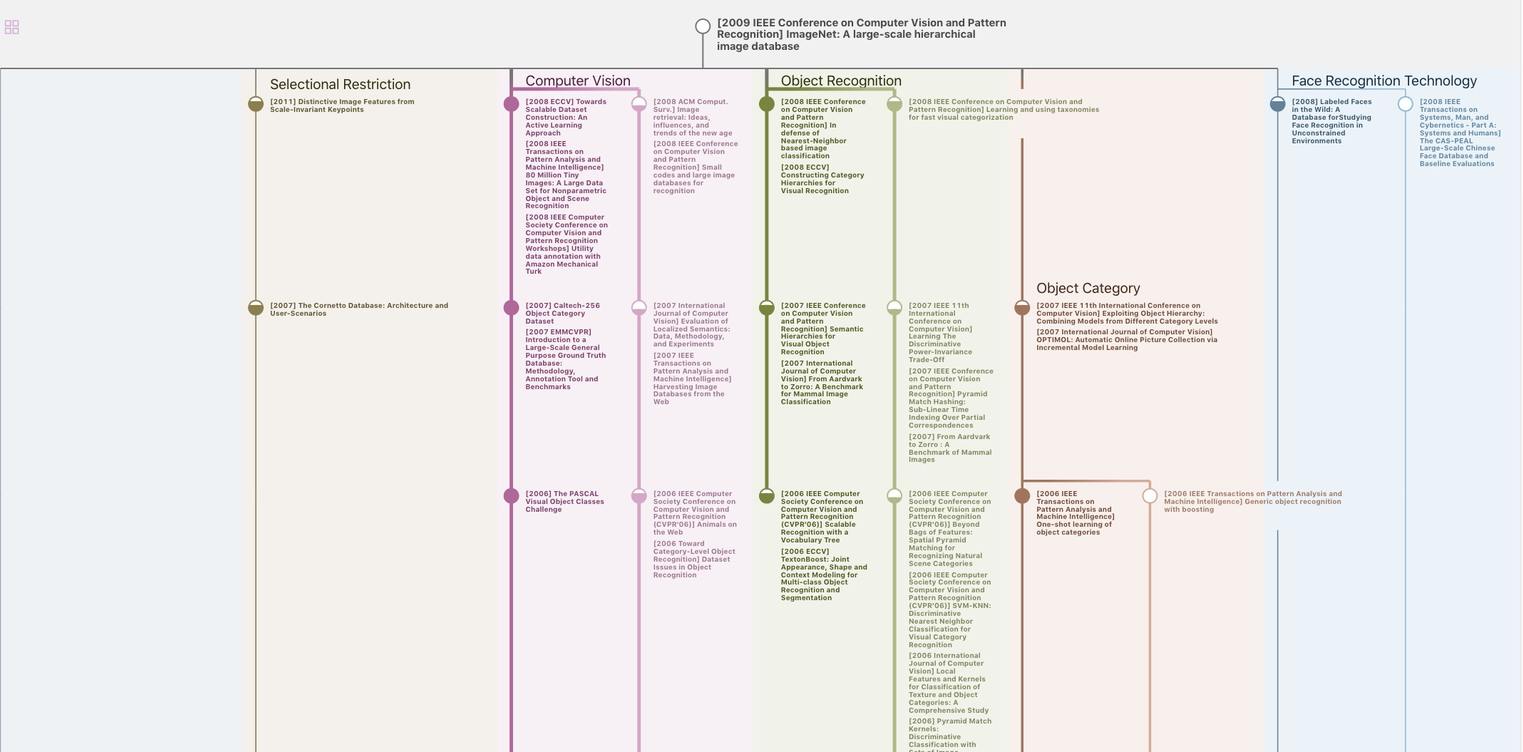
生成溯源树,研究论文发展脉络
Chat Paper
正在生成论文摘要