Virtual Adversarial Training for Semi-supervised Breast Mass Classification
BIOPHOTONICS AND IMMUNE RESPONSES XVII(2022)
摘要
The study aims to develop a novel computer-aided diagnosis (CAD) scheme for mammographic breast mass classification using semi-supervised learning. Although supervised deep learning has achieved huge success across various medical image analysis tasks, its success relies on large amounts of high-quality annotations, which can be challenging to acquire in practice. To overcome this limitation, we propose employing a semi-supervised method, i.e., virtual adversarial training (VAT), to leverage and learn useful information underlying in unlabeled data for better classification of breast masses. Accordingly, our VAT-based models have two types of losses, namely supervised and virtual adversarial losses. The former loss acts as in supervised classification, while the latter loss aims at enhancing the model's robustness against virtual adversarial perturbation, thus improving model generalizability. To evaluate the performance of our VAT-based CAD scheme, we retrospectively assembled a total of 1024 breast mass images, with equal number of benign and malignant masses. A large CNN and a small CNN were used in this investigation, and both were trained with and without the adversarial loss. When the labeled ratios were 40% and 80%, VAT-based CNNs delivered the highest classification accuracy of 0.740 +/- 0.015 and 0.760 +/- 0.015, respectively. The experimental results suggest that the VAT-based CAD scheme can effectively utilize meaningful knowledge from unlabeled data to better classify mammographic breast mass images.
更多查看译文
关键词
Computer-aided diagnosis, breast mass classification, digital mammogram, virtual adversarial training, semi-supervised learning
AI 理解论文
溯源树
样例
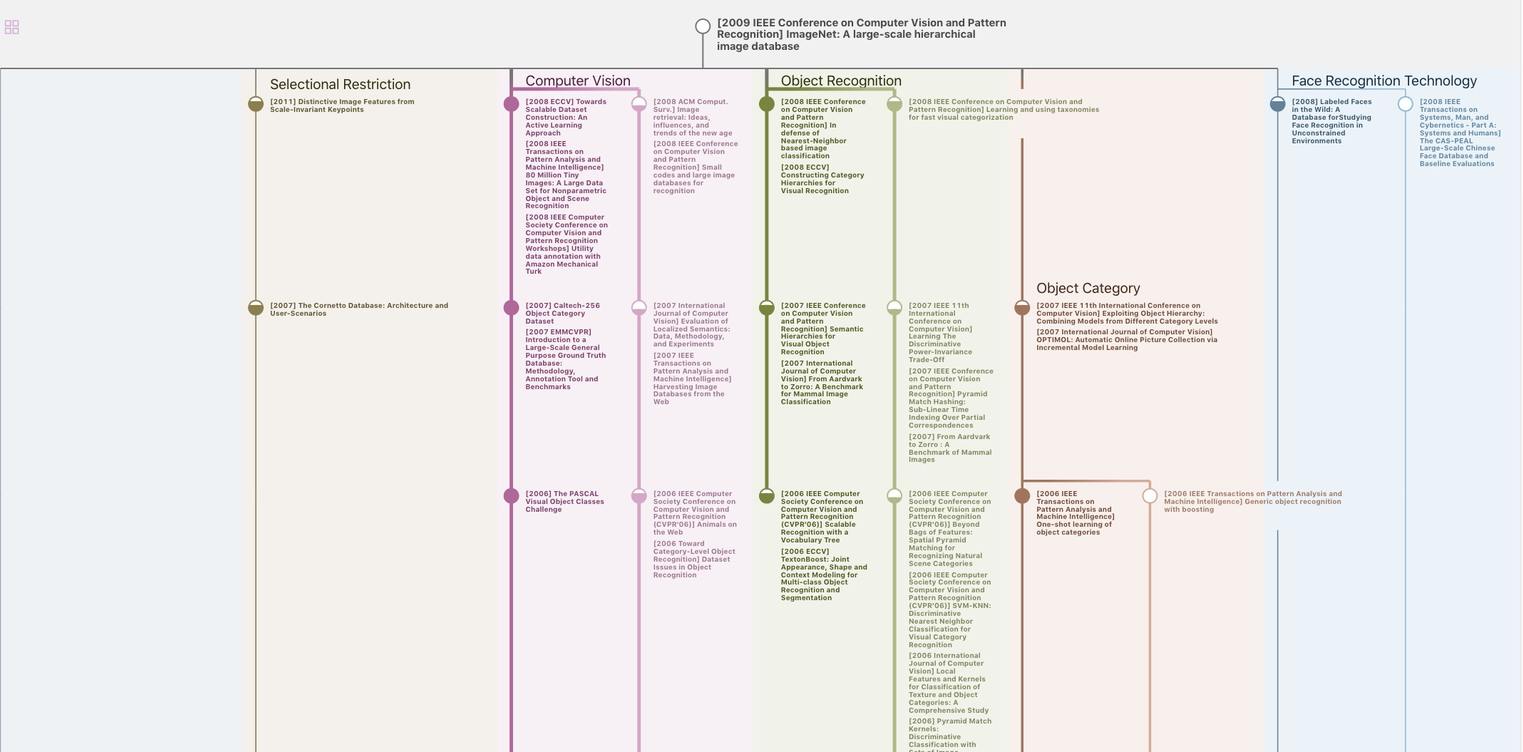
生成溯源树,研究论文发展脉络
Chat Paper
正在生成论文摘要