CodeRetriever: Unimodal and Bimodal Contrastive Learning for Code Search
arxiv(2022)
摘要
In this paper, we propose the CodeRetriever model, which learns the function-level code semantic representations through large-scale code-text contrastive pre-training. We adopt two contrastive learning schemes in CodeRetriever: unimodal contrastive learning and bimodal contrastive learning. For unimodal contrastive learning, we design an unsupervised learning approach to build semantic-related code pairs based on the documentation and function name. For bimodal contrastive learning, we leverage the documentation and in-line comments of code to build code-text pairs. Both contrastive objectives can fully leverage large-scale code corpus for pre-training. Extensive experimental results show that CodeRetriever achieves new state-of-the-art with significant improvement over existing code pre-trained models, on eleven domain/language-specific code search tasks with six programming languages in different code granularity (function-level, snippet-level and statement-level). These results demonstrate the effectiveness and robustness of CodeRetriever.
更多查看译文
关键词
bimodal contrastive learning,unimodal,search
AI 理解论文
溯源树
样例
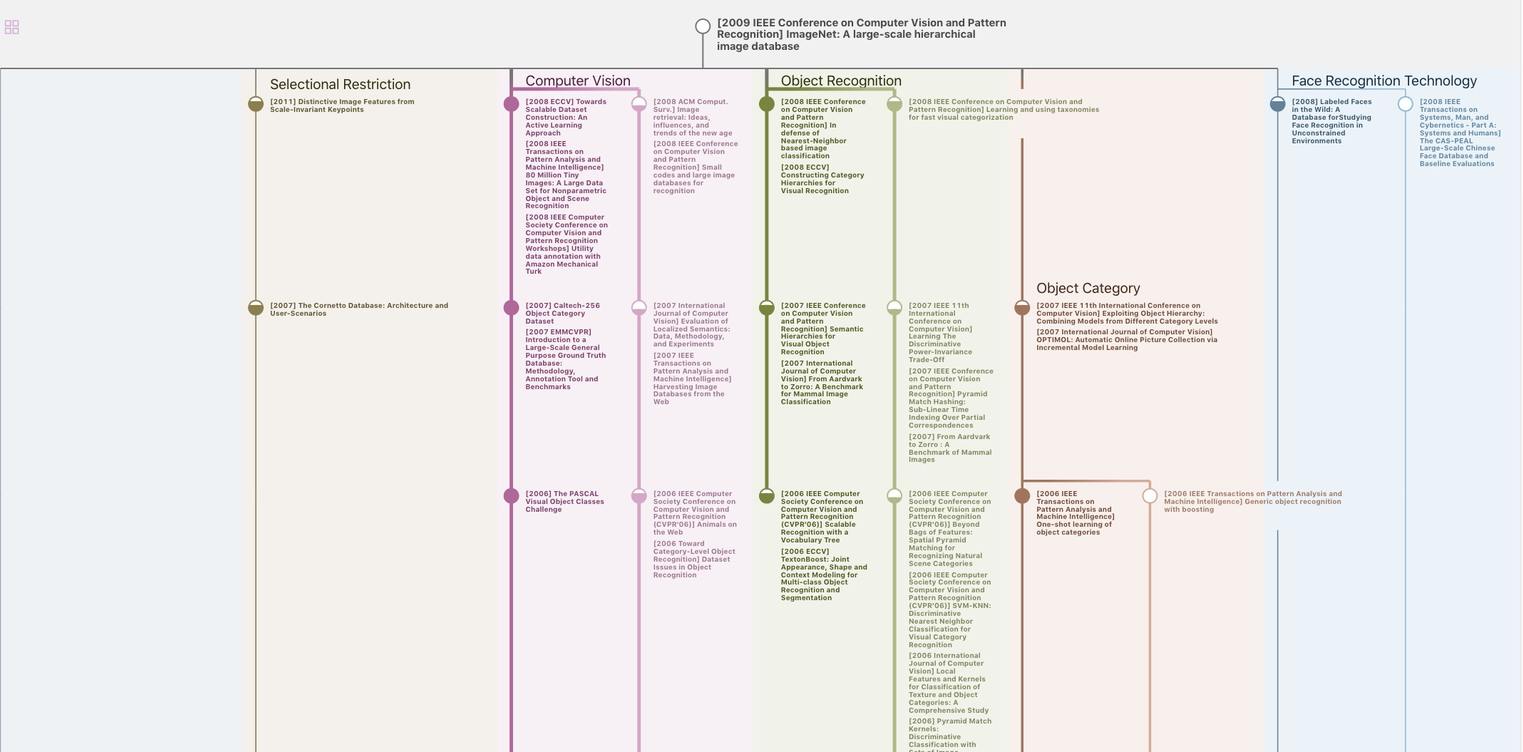
生成溯源树,研究论文发展脉络
Chat Paper
正在生成论文摘要