Forecasting the Market with Machine Learning Algorithms: an Application of NMC-BERT-LSTM-DQN-X Algorithm in Quantitative Trading
ACM transactions on knowledge discovery from data(2022)
摘要
Although machine learning (ML) algorithms have been widely used in forecasting the trend of stock market indices, they failed to consider the following crucial aspects for market forecasting: (1) that investors’ emotions and attitudes toward future market trends have material impacts on market trend forecasting (2) the length of past market data should be dynamically adjusted according to the market status and (3) the transition of market statutes should be considered when forecasting market trends. In this study, we proposed an innovative ML method to forecast China's stock market trends by addressing the three issues above. Specifically, sentimental factors (see Appendix [1] for full trans) were first collected to measure investors’ emotions and attitudes. Then, a non-stationary Markov chain (NMC) model was used to capture dynamic transitions of market statutes. We choose the state-of-the-art (SOTA) method, namely, Bidirectional Encoder Representations from Transformers ( BERT ), to predict the state of the market at time t , and a long short-term memory ( LSTM ) model was used to estimate the varying length of past market data in market trend prediction, where the input of LSTM (the state of the market at time t ) was the output of BERT and probabilities for opening and closing of the gates in the LSTM model were based on outputs of the NMC model. Finally, the optimum parameters of the proposed algorithm were calculated using a reinforced learning-based deep Q-Network. Compared to existing forecasting methods, the proposed algorithm achieves better results with a forecasting accuracy of 61.77%, annualized return of 29.25%, and maximum losses of −8.29%. Furthermore, the proposed model achieved the lowest forecasting error: mean square error (0.095), root mean square error (0.0739), mean absolute error (0.104), and mean absolute percent error (15.1%). As a result, the proposed market forecasting model can help investors obtain more accurate market forecast information.
更多查看译文
关键词
Machine learning,forecasting,quantitative trading,eXogenous variables,SOTA
AI 理解论文
溯源树
样例
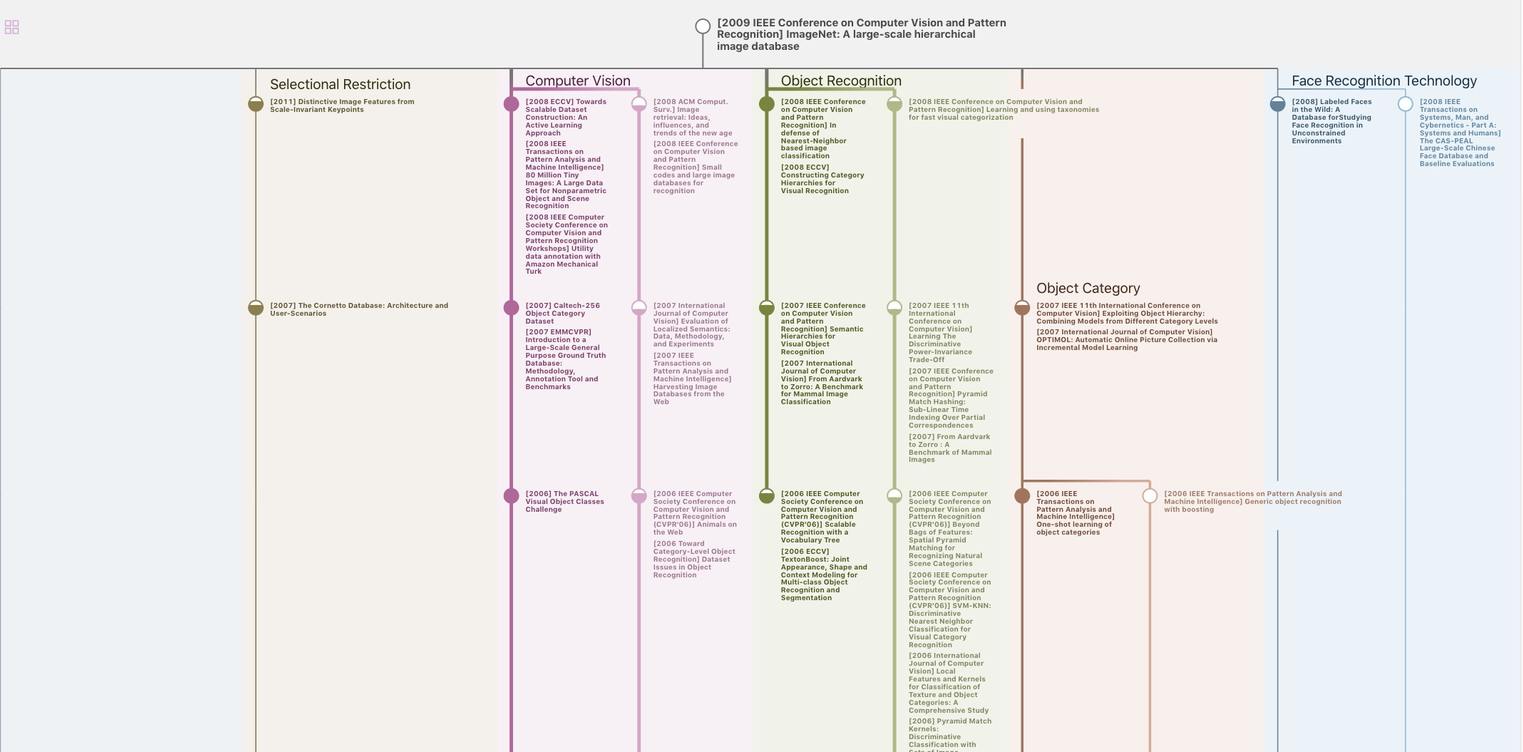
生成溯源树,研究论文发展脉络
Chat Paper
正在生成论文摘要