Transfer inhibitory potency prediction to binary classification: A model only needs a small training set
COMPUTER METHODS AND PROGRAMS IN BIOMEDICINE(2022)
摘要
One of the most laborious for drug discovery is to select compounds from a library for experimental evaluation. Hence, we propose a machine learning model only needs to be trained on a small dataset to predict the inhibition constant (Ki) and half maximal inhibitory concentration (IC50) for a compound. We transfer the prediction task to a simpler binary classification task based on a naive but effective idea that we only need the related rank of a compound to determine whether to take it for further examination. To achieve this, we design a data augmentation strategy to effectively leverage the relationship between the compounds in the training set. After that, we formulate a new reward function for deep reinforcement learning to balance the feature selection and the accuracy. We employ a particle swarm optimized support vector machine for the binary classification task. Finally, a soft voting mechanism is introduced to solve the contradiction from the binary classification. Sufficient experiments show that our model achieves high and reliable accuracy, and is capable of ranking compounds based on a selected set of molecular descriptors. The current results show that our model provides a potential ligand-based in silico approach for prioritizing chemicals for experimental studies. (C) 2022 Published by Elsevier B.V.
更多查看译文
关键词
Drug screening, Inhibitory potency prediction, Machine learning, Deep reinforcement learning, Feature selection
AI 理解论文
溯源树
样例
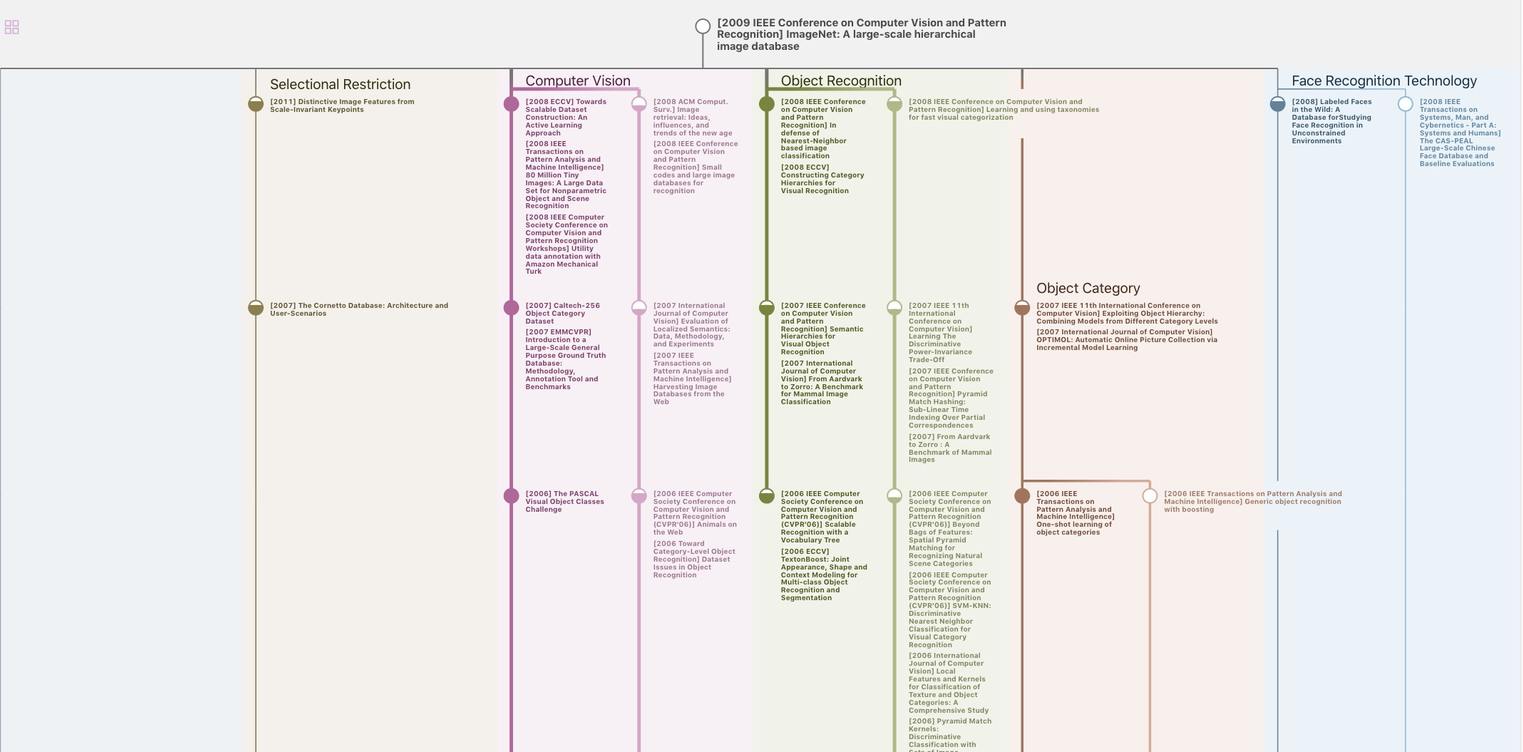
生成溯源树,研究论文发展脉络
Chat Paper
正在生成论文摘要