Respiratory support status from EHR data for adult population: classification, heuristics, and usage in predictive modeling
JOURNAL OF THE AMERICAN MEDICAL INFORMATICS ASSOCIATION(2022)
摘要
Objective Respiratory support status is critical in understanding patient status, but electronic health record data are often scattered, incomplete, and contradictory. Further, there has been limited work on standardizing representations for respiratory support. The objective of this work was to (1) propose a practical terminology system for respiratory support methods; (2) develop (meta-)heuristics for constructing respiratory support episodes; and (3) evaluate the utility of respiratory support information for mortality prediction. Materials and Methods All analyses were performed using electronic health record data of COVID-19-tested, emergency department-admit, adult patients at a large, Midwestern healthcare system between March 1, 2020 and April 1, 2021. Logistic regression and XGBoost models were trained with and without respiratory support information, and performance metrics were compared. Importance of respiratory-support-based features was explored using absolute coefficient values for logistic regression and SHapley Additive exPlanations values for the XGBoost model. Results The proposed terminology system for respiratory support methods is as follows: Low-Flow Oxygen Therapy (LFOT), High-Flow Oxygen Therapy (HFOT), Non-Invasive Mechanical Ventilation (NIMV), Invasive Mechanical Ventilation (IMV), and ExtraCorporeal Membrane Oxygenation (ECMO). The addition of respiratory support information significantly improved mortality prediction (logistic regression area under receiver operating characteristic curve, median [IQR] from 0.855 [0.852-0.855] to 0.881 [0.876-0.884]; area under precision recall curve from 0.262 [0.245-0.268] to 0.319 [0.313-0.325], both P < 0.01). The proposed generalizable, interpretable, and episodic representation had commensurate performance compared to alternate representations despite loss of granularity. Respiratory support features were among the most important in both models. Conclusion Respiratory support information is critical in understanding patient status and can facilitate downstream analyses.
更多查看译文
关键词
respiratory support, oxygen support, supplemental oxygen, electronic health records, predictive analytics, machine learning
AI 理解论文
溯源树
样例
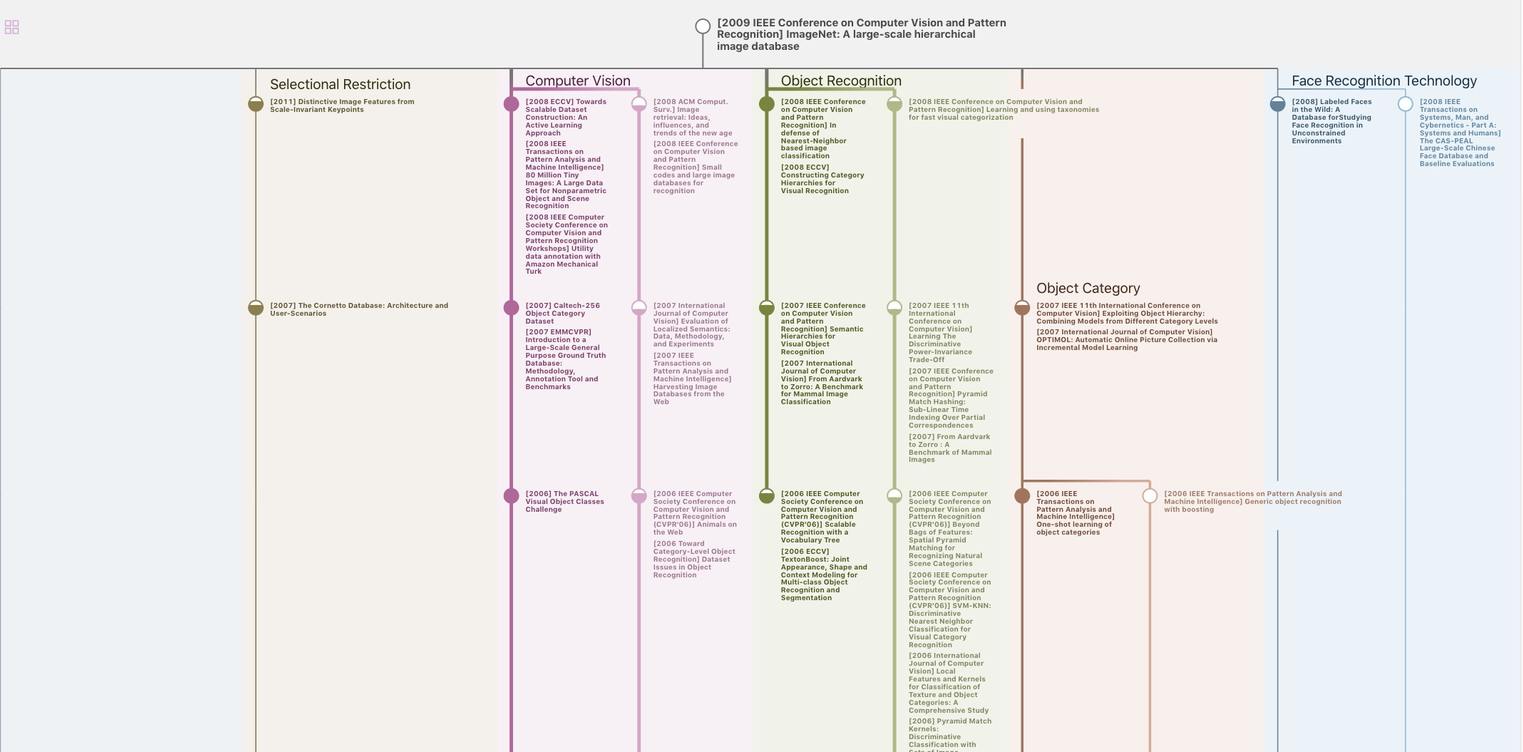
生成溯源树,研究论文发展脉络
Chat Paper
正在生成论文摘要