Unsupervised Anomaly Detection in 3D Brain MRI using Deep Learning with Multi-Task Brain Age Prediction
MEDICAL IMAGING 2022: COMPUTER-AIDED DIAGNOSIS(2022)
摘要
Lesion detection in brain Magnetic Resonance Images (MRIs) remains a challenging task. MRIs are typically read and interpreted by domain experts, which is a tedious and time-consuming process. Recently, unsupervised anomaly detection (UAD) in brain MRI with deep learning has shown promising results to provide a quick, initial assessment. So far, these methods only rely on the visual appearance of healthy brain anatomy for anomaly detection. Another biomarker for abnormal brain development is the deviation between the brain age and the chronological age, which is unexplored in combination with UAD. We propose deep learning for UAD in 3D brain MRI considering additional age information. We analyze the value of age information during training, as an additional anomaly score, and systematically study several architecture concepts. Based on our analysis, we propose a novel deep learning approach for UAD with multi-task age prediction. We use clinical T1-weighted MRIs of 1735 healthy subjects and the publicly available BraTs 2019 data set for our study. Our novel approach significantly improves UAD performance with an AUC of 92.60% compared to an AUC-score of 84.37% using previous approaches without age information.
更多查看译文
关键词
Anomaly Detection,Brain Age Prediction,Unsupervised,Brain MRI,3D Autoencoder
AI 理解论文
溯源树
样例
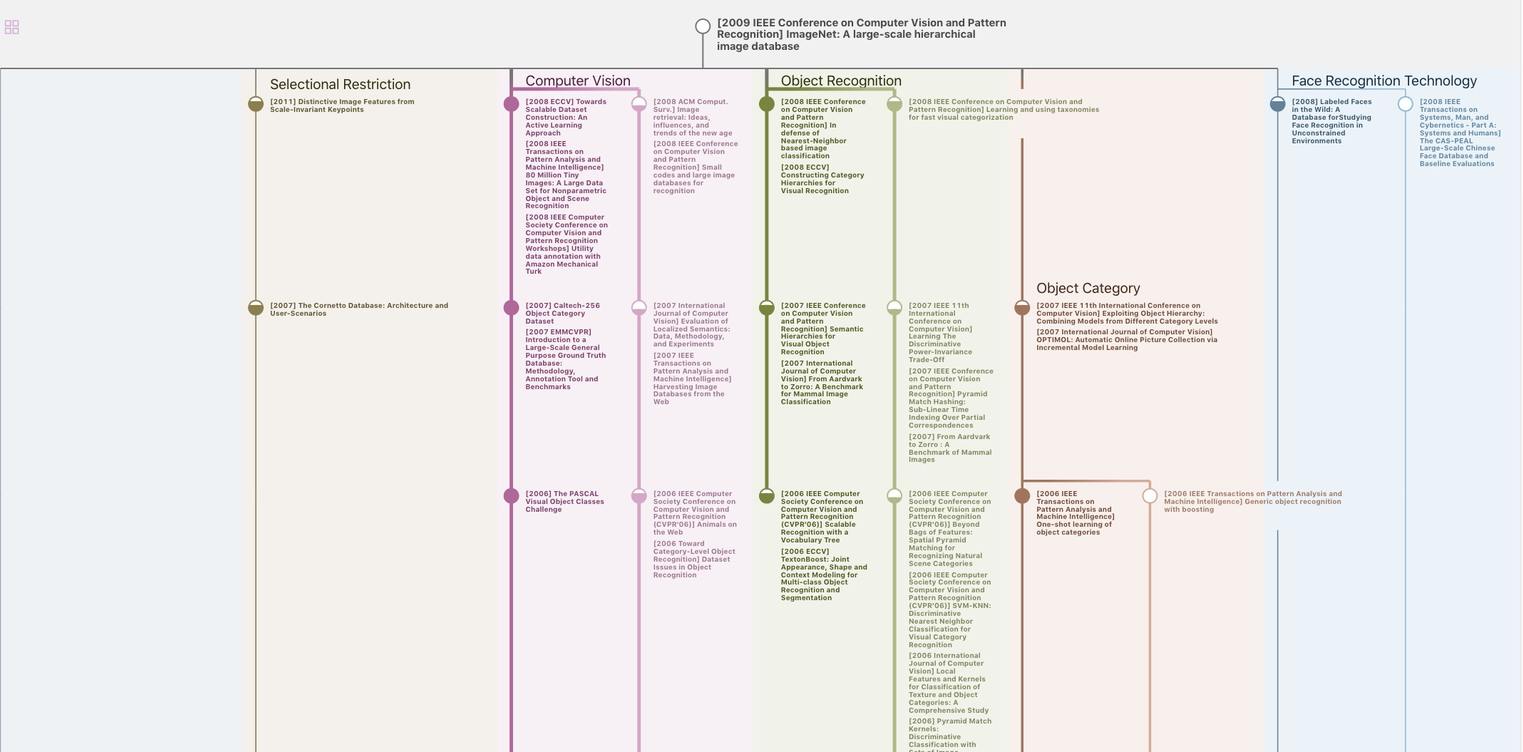
生成溯源树,研究论文发展脉络
Chat Paper
正在生成论文摘要