Near-Optimal Regret for Adversarial MDP with Delayed Bandit Feedback
NeurIPS 2022(2022)
摘要
The standard assumption in reinforcement learning (RL) is that agents observe feedback for their actions immediately. However, in practice feedback is often observed in delay. This paper studies online learning in episodic Markov decision process (MDP) with unknown transitions, adversarially changing costs, and unrestricted delayed bandit feedback. More precisely, the feedback for the agent in episode $k$ is revealed only in the end of episode $k + d^k$, where the delay $d^k$ can be changing over episodes and chosen by an oblivious adversary. We present the first algorithms that achieve near-optimal $\sqrt{K + D}$ regret, where $K$ is the number of episodes and $D = \sum_{k=1}^K d^k$ is the total delay, significantly improving upon the best known regret bound of $(K + D)^{2/3}$.
更多查看译文
关键词
regret minimization,adversarial mdp,delay,reinforcement learning,online learning
AI 理解论文
溯源树
样例
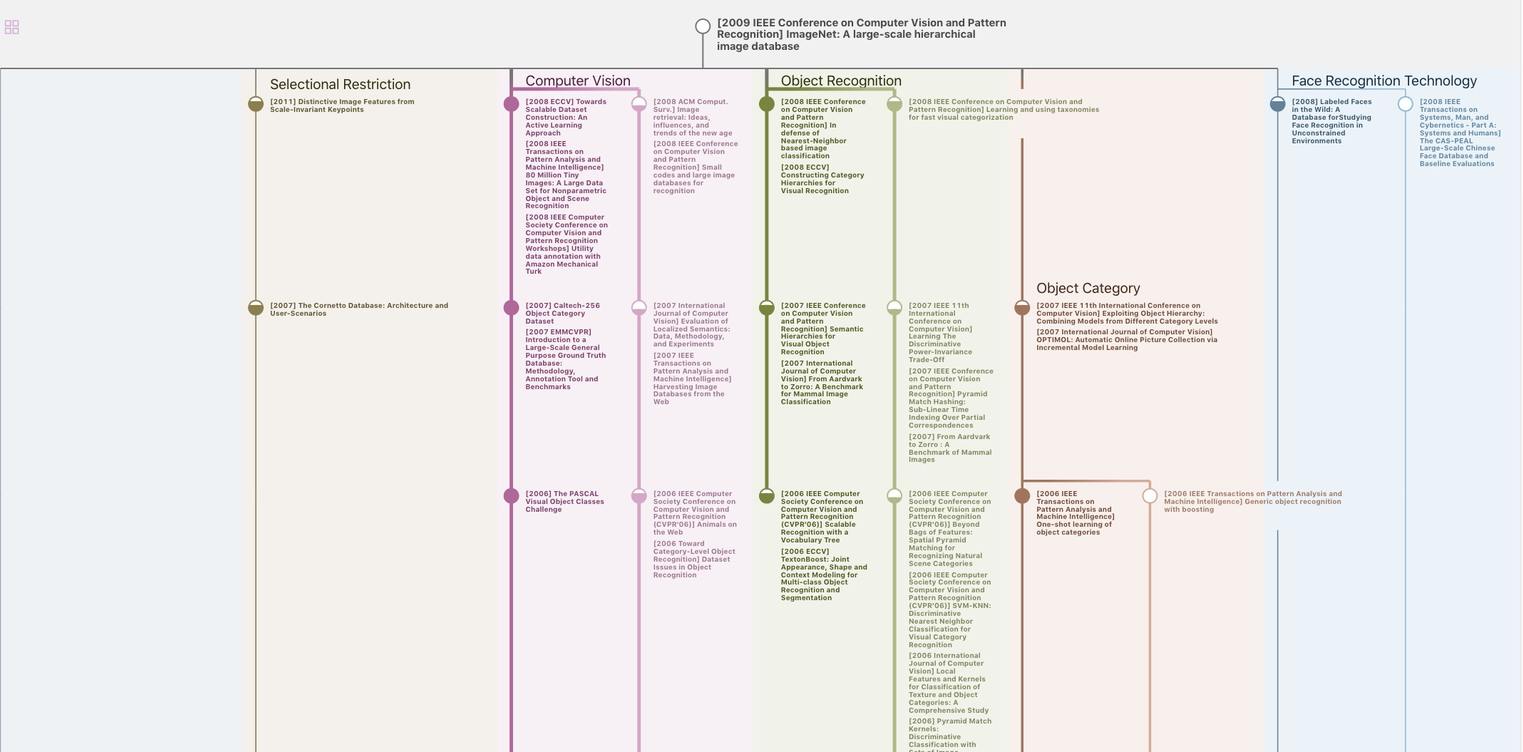
生成溯源树,研究论文发展脉络
Chat Paper
正在生成论文摘要