Eris: Measuring discord among multidimensional data sources
arxiv(2022)
摘要
Data integration is a classical problem in databases, typically decomposed into schema matching, entity matching and record merging. To solve the latter, it is mostly assumed that ground truth can be determined, either as master data or from user feedback. However, in many cases, this is not the case because firstly the merging processes cannot be accurate enough, and also the data gathering processes in the different sources are simply imperfect and cannot provide high quality data. Instead of enforcing consistency, we propose to evaluate how concordant or discordant sources are as a measure of trustworthiness (the more discordant are the sources, the less we can trust their data). Thus, we define the discord measurement problem in which given a set of uncertain raw observations or aggregate results (such as case/hospitalization/death data relevant to COVID-19) and information on the alignment of different data (for example, cases and deaths), we wish to assess whether the different sources are concordant, or if not, measure how discordant they are. We also define a set of algebraic operators to describe the alignments, together with two alternative relational implementations that reduce the problem to linear or quadratic programming. These are evaluated against both COVID-19 and synthetic data, and our experimental results show that discordancy measurement can be performed efficiently in realistic situations.
更多查看译文
关键词
discord,data
AI 理解论文
溯源树
样例
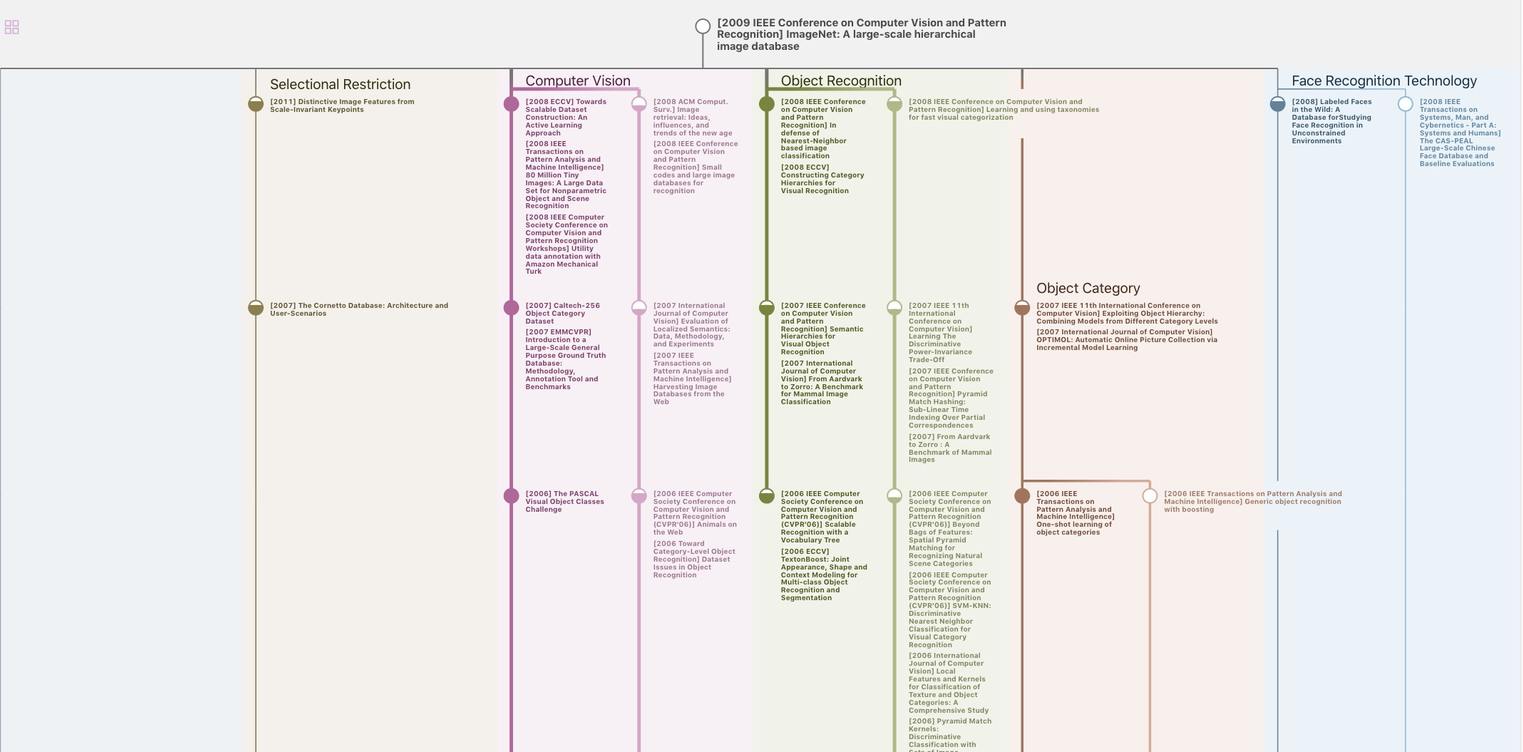
生成溯源树,研究论文发展脉络
Chat Paper
正在生成论文摘要