Studying the Robustness of Anti-Adversarial Federated Learning Models Detecting Cyberattacks in IoT Spectrum Sensors
IEEE Transactions on Dependable and Secure Computing/IEEE transactions on dependable and secure computing(2024)
摘要
Device fingerprinting combined with Machine and Deep Learning (ML/DL) report promising performance when detecting spectrum sensing data falsification (SSDF) attacks. However, the amount of data needed to train models and the scenario privacy concerns limit the applicability of centralized ML/DL. Federated learning (FL) addresses these drawbacks but is vulnerable to adversarial participants and attacks. The literature has proposed countermeasures, but more effort is required to evaluate the performance of FL detecting SSDF attacks and their robustness against adversaries. Thus, the first contribution of this work is to create an FL-oriented dataset modeling the behavior of resource-constrained spectrum sensors affected by SSDF attacks. The second contribution is a pool of experiments analyzing the robustness of FL models according to i) three families of sensors, ii) eight SSDF attacks, iii) four FL scenarios dealing with anomaly detection and binary classification, iv) up to 33% of participants implementing data and model poisoning attacks, and v) four aggregation functions acting as anti-adversarial mechanisms. In conclusion, FL achieves promising performance when detecting SSDF attacks. Without anti-adversarial mechanisms, FL models are particularly vulnerable with $>$ 16% of adversaries. Coordinate-wise-median is the best mitigation for anomaly detection, but binary classifiers are still affected with $>$ 33% of adversaries.
更多查看译文
关键词
Resource-constrained devices,cyberattacks,fingerprinting,federated learning,adversarial attacks,robustness
AI 理解论文
溯源树
样例
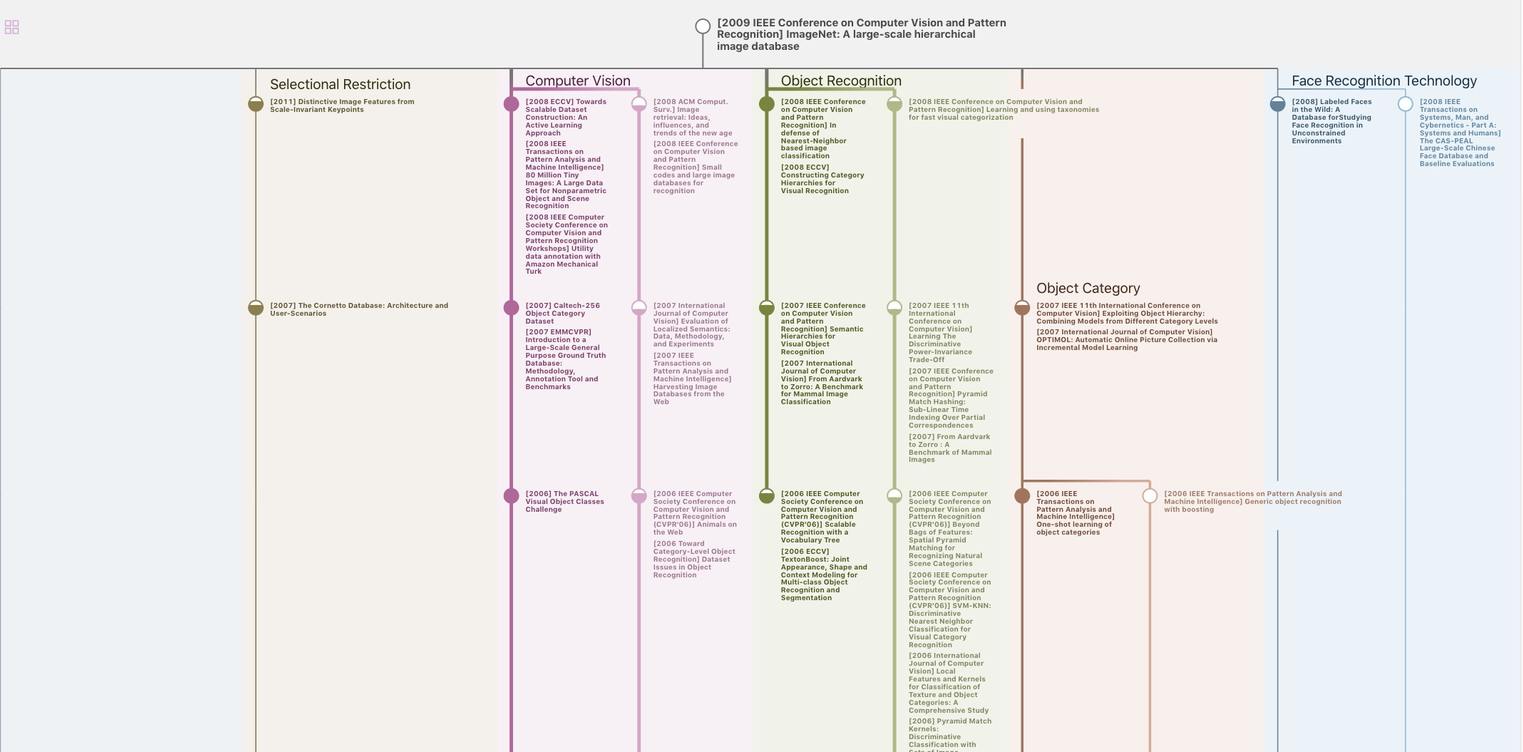
生成溯源树,研究论文发展脉络
Chat Paper
正在生成论文摘要