Machine Learning-Enabled Prediction and High-Throughput Screening of Polymer Membranes for Pervaporation Separation
ACS APPLIED MATERIALS & INTERFACES(2022)
摘要
Pervaporation (PV) is considered as a robust membrane-based separation technology for liquid mixtures. However, the development of PV membranes is impeded largely by the lack of adequate models capable of reliably predicting the performance of PV membranes. In this study, we collect an experimental data set with a total of 681 data samples including 16 polymers and 6 organic solvents for a wide variety of water/organic mixtures under various operating conditions. Then, two types of machine learning (ML) models are developed for prediction and high-throughput screening of polymer membranes for PV separation. Based on the intrinsic properties of polymer and solvent (water contact angle of polymer and solubility parameter of solvent) as gross descriptors, the first type accurately predicts PV separation performance (total flux and separation factor). The second type is based on the molecular representation of polymer and solvent, giving accuracy comparable to the first type, and applied to screen similar to 1 million hypothetical polymers for PV separation of water/ethanol mixtures. With a threshold of 700 for the PV separation index, 20 polymers are shortlisted, with many surpassing experimental samples. Among these, 10 are further identified to be synthesizable in terms of a synthetic complexity score. The ML models developed in this study would facilitate the optimization of operating conditions and accelerate the development of new polymer membranes for high-performance PV separation.
更多查看译文
关键词
pervaporation, membranes, polymers, machine learning, prediction, screening
AI 理解论文
溯源树
样例
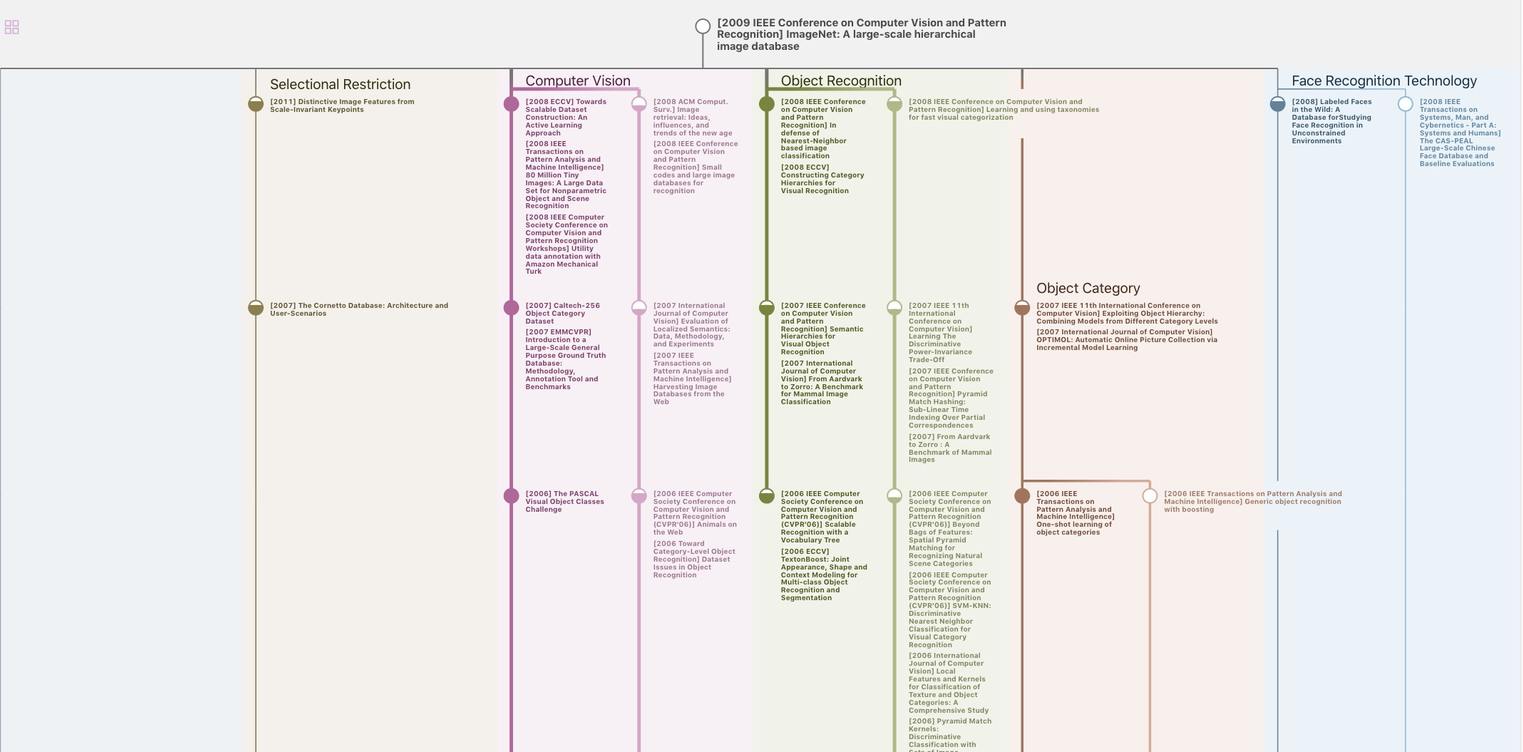
生成溯源树,研究论文发展脉络
Chat Paper
正在生成论文摘要